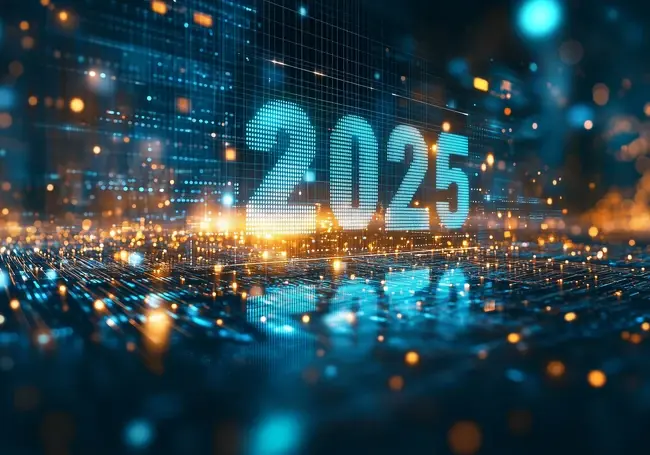
Expect Everything to Change
As we approach 2025, one question dominates: What will change? In the age of generative AI, the answer is simple—everything.
ChatBots Take Over. The rapid sophistication and scalability of AI chatbots will fundamentally change the way business users interact with data and tools. Gone are the days when users need to understand how to query or analyze data using SQL, a BI tool, or even a machine learning tool (as Elliott Cordo maintains). The interface of choice will be a chatbot, which has escaped the bounds of text and can now read structured data, giving users the ability to ask analytical questions in natural language and get an accurate answer. James Serra concludes that this will usher in an era of AI-powered decision-making.
But other experts warn that generative AI carries considerable risk. Some risks are intrinsic to AI, others are created by the data that feeds genAI models, and others result from how organizations implement AI chatbots. Dave Wells predicts companies will spend more effort evaluating data bias in 2025, while Matthew Arellano believes organizations will implement AI gateways to prevent divergent views of “chat truth.” Allen Wishman thinks we shouldn’t get too attached to LLMs since neuro-symbolic models might replace them, and Henrik Strandberg believes 100% of job screening interviews will be conducted via conversational bots.
ChatGPT’s Prediction. To get the inside scoop on generative AI, we went right to the source: we asked ChatGPT for a 2025 prediction. It responded with something quite novel: 2025 will see “the rise of data responsibility platforms (DRPs) designed to measure, monitor, and ensure responsible data usage alongside traditional analytics.” I couldn’t find reference to DRPs on Google, meaning ChatGPT did what any experienced industry analyst does and defined a new category of technology based on current trends. (Of course, cynics will say that either ChatGPT hallucinated or industry analysts always hallucinate and call it a job!) Either way, this former industry analyst is glad he’s now a full-time consultant! Or maybe Dave Wells is a chatbot since his prediction mirrors ChatGPT’s prediction. (Sorry Dave!)
Non-GenAI Predictions. Believe it or not, there is more to the future than GenAI. Michael Hejtmanek believes data governance will embrace both data protection and data enablement; Sean Hewitt believes quantum computing poses serious risks to data privacy and security; Elliott Cordo thinks data contracts will become a standard element in data pipelines; Gordon Wong believes semantic layers will become a standard repository for business rules; and yours truly believes data catalogs will become a standard component of data stacks.
We’d love to hear your predictions for 2025 and your reactions to ours!
Generative AI Predictions
From Rows to Riches: How Generative AI Will Dominate Structured Data in 2025
By James Serra, Data and AI Solutions Architect, Microsoft
Generative AI, once a tool confined to unstructured text like emails and documents, is poised to transform how we engage with structured data in 2025. Advancements in AI capabilities now enable seamless interaction with relational databases, spreadsheets, and CSV files—unlocking insights that were once buried under layers of complexity. With tools like ChatGPT, Microsoft Copilot, and Fabric AI Skills, businesses will shift from rigid query-based systems to conversational interfaces, extracting actionable insights with unprecedented ease and speed. By identifying patterns, generating predictions, and driving smarter decisions, generative AI is rewriting the rules of structured data analytics. As the technology matures, traditional data analysis workflows may become relics of the past, replaced by a new era of dynamic, AI-powered decision-making. In 2025, the convergence of AI and structured data won’t just enhance efficiency—it will redefine how organizations think, plan, and innovate.
AI Governance Has High Priority But Misguided Execution.
By Dave Wells, Senior Analyst Emeritus, Eckerson Group
Attention to AI Governance will be amplified early in 2025. As the EU AI Act takes effect, business and data leaders recognize the AI Governance imperative and prioritize it as essential and strategic. Despite this focus, most companies will still do an inadequate job of implementing AI Governance. They will emphasize regulatory compliance at the expense of AI bias. This approach misses a critical point: The goal of AI Governance shouldn’t be to avoid fines and penalties; it should be Do No Harm. Harmless AI must be fair and unbiased. Governance practices that consider bias are generally skewed toward minimizing model bias, neglecting the greater risk of data bias. To be fully effective, AI Governance must include rethinking data quality management. Data quality today focuses on correctness, integrity, and usability. AI Governance requires objectivity (absence of bias) as a fourth category with known indicators of bias and the right processes to detect, measure, and monitor data bias.
With So Many Co-Pilots Who is Flying the Plane?
By Matthew Arellano, Co-Founder, XponentL Data
In 2025, AI chatbots and co-pilots will proliferate within organizations, creating divergent versions of chat truth. These AI chatbots and co-pilots will be trained on different data and semantics, meaning users will get different answers to the same question depending on which chatbot they use. Currently, there are three categories of AI chatbots 1) those embedded in software applications, such as ServiceNow 2) those developed internally for business domains, such as HR, supply chain, etc., and 3) general-purpose chatbots, such as ChatGPT, Claude, etc.
To rein in the flood of chatbots, companies will establish AI Chatbot Gateways to consolidate all chatbot requests and funnel them to official domain-specific chatbots. At the same time, they will develop a standardized approach to evaluate, monitor, and govern AI chatbots to prevent general misuse, data loss prevention, PII / PHI risks, hallucinations, etc. The combination of AI ChatBot Gateways, official domain-specific chatbots, and governance standards will ensure companies deliver a single version of chat truth.
AI kills ML
By Elliott Cordo, Founder, DataFutures
2025 will be the year of mainstream AI adoption in the enterprise, but perhaps not in the way that you think. Over the past two years, many organizations, especially in the startup space, have been leveraging pre-trained AI models in place of traditional Machine Learning models. These use cases include feature extraction, labeling, classification, summarization, and general-purpose inference and reasoning operations. This has not only provided amazing time to benefit but largely avoided the need for specialized data science and ML skill sets. Additionally, many organizations lack high-quality labeled data, which is critical to training traditional ML models. On the other hand, pre-trained AI models need only a small number of labeled examples, or in some cases, none at all, enabling use cases that would have otherwise not been possible.
Neuro-Symbolic Models Become a Major Competitor to Transformer Model
By Allen Wishman, Senior Consultant, Eckerson Group
Neuro-symbolic models (NSM) combine the strengths of deep learning (neural networks) with symbolic AI (logic, knowledge representation). NSMs can learn patterns from data while also reasoning and explaining their decisions based on explicit knowledge. An intriguing difference between NSM and traditional transformer-based models like ChatGP, is the strong reasoning and explainability characteristics of these models. They excel at logical reasoning, handling complex relationships, and providing human-understandable explanations for their outputs, unlike black-box neural networks. They often require less training data than purely neural models, as they can leverage prior knowledge and logical rules. Some companies working to enable these models state costs & time savings of 100X less than transformer-based models. Due to these properties, it is no surprise that IBM, Google, Microsoft, Numenta, and several startups are exploring and developing these types of models. They may never have the number of users as models in the market today, however, there are many use cases that may only be able to use this type of model if they are to take advantage of AI.
Conversational AI Bots Usher in New Era of Job Interviews
By Henrik Strandberg, Digital Transformation Consultant
In 2025, we will start seeing conversational AI bots conduct phone screens with ALL applicants for certain roles, not just the 1-5% whose resumes were tagged by the recruiting software as containing the right mix of keywords and credentials. These AI phone screeners will be immune to common interview biases (accent, gender, jargon, name-dropping, etc), and pave the way for equitable and truly skill-based (rather than merit-based) hiring practices.
The Rise of Data Responsibility Platforms
By ChatGPT
By 2025, organizations will pivot toward platforms explicitly designed to manage data ethics, accountability, and societal impact. These Data Responsibility Platforms (DRPs) will become the next frontier for enterprises, offering tools to measure, monitor, and ensure responsible data usage alongside traditional analytics. The move to DRPs is driven by growing government regulation of data and AI, increased public concern about AI bias, surveillance, and misuse, and corporate risk management. Key features of a DRP include data lineage auditing, AI impact scoring, privacy engineering, and dashboards tailored to various stakeholders, such as consumers, regulators, and executives.
Non-GenAI Predictions
Data Governance Focuses on Enablement
By Michael Hejtmanek, Vice President, Corporate Solutions, Neudata
Data governance is going to undergo a revolution in 2025. Some data-leading orgs have already recognized how smarter policies that surgically broaden data access can add value. The fear of the new and unknown that has plagued the use of data for so long is beginning to recede. In 2025, companies will create data policies less based on fear and risk mitigation and more based on a rational evaluation of the risks versus rewards of providing more expansive access to data. This relaxation of data sharing will first happen internally within organizations and eventually shift to external data sharing.
Quantum Computing Poses New Cybersecurity Threats
By Sean Hewitt, Senior Consultant, Eckerson Group
As organizations start using quantum computers, they will increase their risks of data privacy breaches. Sensitive information that is encrypted today may no longer be safe once quantum computing becomes mainstream. Many of today’s encryption methods (e.g., RSA, ECC) rely on the difficulty of factoring large numbers or solving complex mathematical problems. Quantum computers could solve these problems exponentially faster than classical computers, making it possible for data thieves to today’s encryption locks. As quantum computing evolves, companies may need to update their compliance frameworks to adhere to new standards addressing quantum threats, potentially leading to increased costs and complexity in cybersecurity governance.
The Year of Data Contracts
By Elliott Cordo, Founder, DataFutures
Data Mesh rather quietly passed through the classic "trough" and is starting to ascend the "slope". Data Mesh was conceptual, and many early adopters were challenged by a lack of tooling, architectural patterns, best practices, and organizational resistance. Despite these challenges, Data Mesh was a great catalyst for adopting modern software engineering practices, with "contracts" gaining the largest momentum. Monolithic data architectures have been one of the biggest drivers of pain and dysfunction in analytics. Tools and standards such as dbt and ODSC (open data contracts standards) provide functional tooling for addressing this problem and accelerating "mesh-like" adoption.
Semantic Layers Displace Data Catalogs
By Gordon Wong, VP of Data and AI, Newfire Global Partners
Semantic layer management tools will integrate and even challenge data catalogs. Traditionally, business metrics lacked a home, leading to inconsistent data views for BI tools. Previous business layer attempts failed due to poor SQL generation and limited BI tool interfacing. However, advances in cloud data warehouses, better semantic layer to SQL conversion, and AI's text-to-semantic layer handling have made semantic layers viable for analytics. If the semantic layer becomes the home for business metrics, it could extend to become the hub for all analytics and data processing metadata, like pipelines, lineage, and data catalog.
Data Catalogs Become a Core Component
By Wayne Eckerson, President, Eckerson Group
Managing metadata has been a huge challenge since the beginning of the data warehousing era 30 years ago. But now there is a generally accepted solution for managing metadata at an enterprise level: data catalogs. First developed to facilitate the discovery of data by power users, data catalogs are now a staple of data governance teams that need to manage data quality, privacy, security, and compliance, as well as foster greater data sharing and usage. As a result, the data catalog joins the ranks of the core components required to build a modern data & analytics infrastructure, which includes a data platform, an ELT tool, and a BI tool. We’ve sat on a three-legged data & analytics stool for so long, it might take time to get used to sitting on a chair.
From all of us at Eckerson Group, godspeed on a cozy end to 2025, hopefully, spent with family and friends and a brilliant new year full of hope and possibility for the future.
This article was originally published on Eckerson Group