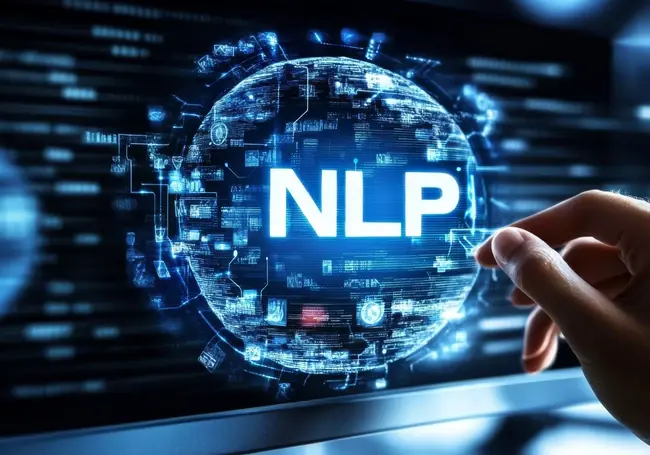
Use of generative AI models is rapidly growing. In the UK alone, the AI market is worth over £21 billion and is projected to reach £1 trillion by 2035. A 2023 government report found that one in six organisations had adopted at least one AI technology.
Among these smart technologies is Natural Language Processing (NLP), a branch of AI enabling computers to understand, interpret, and generate human language. We’re seeing NLP increasingly implemented in everyday business tools and services.
Whether you’re speaking to a virtual assistant like Siri or asking Alexa a question, you’re interacting with NLP models. It’s beginning to play a crucial role in how we communicate, access information, and automate tasks.
Defining Natural Language Processing
Natural Language Processing (NLP) is a form of artificial intelligence (AI) that combines disciplines, such as computer science and computational linguistics. It aims to bridge the gap between human language and computer understanding, allowing machines to work with both text and speech. Only then can NLP models analyse, understand, and respond to user input.
You may see these terms used in relation to NLP: Natural Language Understanding (NLU) and Natural Language Generation (NLG). Both refer to how computers understand and produce human-like language. NLG, for example, has the ability to provide a verbal description of a situation (also called “language out”) by summarising meaningful information in text using a concept called “grammar of graphics”.
Modern NLP applications are powered by large language models (LLMs) and support a wide variety of use cases, including spell checkers, spam filters, and real-time translation. LLMS are used in conjunction with other AI models, such as machine learning and deep learning. Google’s algorithm is an everyday example of an application using NLP to provide relevant search results to user queries.
What is the Purpose of Natural Language Processing?
The main purpose of NLP is to enable computers to understand, interpret, and generate human language in a human-like manner. It allows technology to become more intuitive and accessible for users.
Here are some of the core purposes of NLP:
- To understand human language in written and spoken form.
- To generate human language that replicates how we communicate, such as answering questions, translating text, and summarising large documents.
- To improve human-computer interaction by enabling the computer to understand and respond in “natural language”.
- To automate tasks, such as data extraction, classification, and summarisation, to free up more time and resources for organisations to complete more complex tasks relating to their business goals.
- To analyse text data and identify trends, patterns, and valuable insights.
By supporting better human-to-computer interaction, NLP helps improve organisational decision-making and streamlines operations across a range of industries.
What is natural language processing used for?
This generative model can be used to process any type of text data, including but not limited to:
- Customer reviews and testimonials.
- Emails.
- Survey responses.
- Product descriptions.
- Scientific publications.
NLP has many usages, including the ability to filter spam messages, convert spoken word into text data, and support Search Engine Optimisation (SEO) by understanding user search queries and providing relevant results.
The model is used in every industry. For example, in healthcare, it can analyse medical records to identify patterns, predict diseases, and improve patient care and satisfaction. In law, NLP programs can analyse legal documents to identify relevant information, extract evidence, and streamline processes.
Why is Natural Language Processing Important?
NLP helps computers to communicate with humans in their own language, making it possible for a computer to read text, hear speech, interpret it, measure its sentiment, and determine which aspects are important. It has the ability to work through differences in dialect, slang, and grammatical irregularities often found in day-to-day conversation.
It can analyse far more language data than a human ever could. What makes NLP important is down to the sheer amount of unstructured data generated daily, in which automation will be vital to fully analyse text and speech data efficiently. Around 80 per cent of a company’s data is unstructured. As such, NLP is proving to be an essential tool in many organisations’ arsenal.
Human language complexities
The human language is complex and diverse, offering multiple ways for us to express ourselves — whether that’s spoken or written. And although there are hundreds of languages and dialects, as well as unique grammar and syntax rules, NLP is important because it bridges the gap between human communication and machine learning.
It helps to resolve any ambiguity found in language through its ability to analyse language structure, meaning, and sentiment. It’s through this training and understanding that NLP supports translation, information retrieval, and conversational AI like Chat-GPT.
How Does Natural Language Processing Work?
Natural language processing combines computational linguistics, machine learning, and deep learning models to process human language.
Computational linguistics: The science of understanding and constructing human language models with computer and software tools. Tools like language translators, text-to-speech synthesisers, and speech recognition programs are based on computational linguistics.
Machine learning: Feeds NLP systems large volumes of data, allowing it to learn linguistic patterns and improve its predictions (and the accuracy of its responses).
Deep learning: A specific field of machine learning that teaches computers to learn and think like a human. It functions through neural networks which have data processing nodes structured to resemble the human brain. Deep learning enables computers to recognise, classify, and relate complex patterns in the input data (the data you train the model on).
Is natural language processing the same as machine learning?
Although they seem the same, NLP and machine learning are different but closely linked. NLP applications use machine learning to train systems on language data. A good example of this is algorithms and how it learns to detect patterns, understand syntax, and interpret semantics based on the quantities of data it’s trained on.
Most modern day NLP models rely on machine learning to enhance understanding and improve functionality over time. Sentences, phrases, and even entire books are fed into a machine learning system where the text is processed according to grammatical rules, the user’s linguistic habits, or both. Computers then rely on this data to recognise common patterns and determine what might come next in a sentence.
Human language features sarcasm, metaphors, and variations in sentence structure — to machines, these are complex attributes. By using NLP, computers/applications can recognise and accurately understand these language features from the get go and use the knowledge to its advantages when generating responses.
NLP Use Cases
Companies use NLP to automate many tasks, including but not limited to:
- Processing, analysing and archiving large documents.
- Running chatbots for automated customer service.
- Analyzing customer feedback or call centre recordings.
- Answering Who, What, When and Where questions.
- Classifying and extracting from text.
Organisations can integrate NLP models into its customer-facing applications to help better communicate with customers. For instance, a chatbot can save your business time, as it analyses and sorts customer queries for you. It can also respond automatically to common questions, meaning less resource is used for customer service tasks. It’s this sort of automation that enables businesses to reduce costs, improve customer service and satisfaction, and save time.
Supply operations
The model can help set up complex supply chains to handle inventory management, supplier selection, and order fulfilment. When used efficiently, your organisation can develop quality products at a lower price and enable you to meet your customer’s demands and needs through product development.
Internal operation optimisation
Through extensive NLP analysis, decision-making becomes a less arduous task for your business. An AI program can sieve through unstructured data to identify key areas of your business operations that face prevalent issues. From there, you can make improvements based on the data analysis report provided. For example, NLP can analyse error logs to see exactly where a problem occurred.
Risk prediction
NLP plays a crucial role in enhancing an organisation's risk prediction, through analysing unstructured data (e.g. news articles, customer reviews, and social media posts) to identify any potential risks or trends. It’s this ability that allows for more informed decision-making in industries such as finance, healthcare, and cyber security. While unstructured data is “unstructured”, it does prove useful for predicting potential risks.
Administrative tasks
Manually carrying out administrative tasks, like data entry and form completion, require time, money, and employee resources. With a tailored NLP solution, you can automate these repetitive tasks, freeing up your time to focus your efforts on business projects and goals. As such, generative NLP has a strong use case in industries with realms of regular admin responsibilities.
Regulatory compliance
The key aspect of NLP is automation, allowing your organisation’s compliance staff to save time on manually attempting to identify any potential regulatory violations. E.g. for GDPR purposes. NLP can automatically schedule compliance reviews at set times and highlight potential irregularities or violations to your compliance team. This frees up time and provides better accuracy.
Customer service
NLP programs are frequently used to optimise an organisation’s customer service. For instance, you can improve the value of your services by implementing AI chatbots and voice assistants to provide answers to customer queries much faster than a human might. It makes communication more efficient and effective, helping to improve efficiencies and reduce costs on hiring a large team of customer service advisers.
NLP Implementation
Implementing NLP begins with collecting and preparing unstructured text or speech data from various sources, such as cloud data warehouses, emails, or even internal business processes. This is carried out through the following pre-processing techniques:
Tokenization: The process of breaking down a sentence into individual words or phases and relating those subjects to numerical values.
Stemming: Reducing words down to its base or root form, typically by removing suffixes or prefixes. E.g. “Walking” “walker” and “walked” all have the root word “walk”.
Lemmatisation: A similar concept to stemming in which a word is converted to its base or dictionary form. E.g. “Running” will always become “run” as its dictionary form.
Stop word removal: Removing frequently occurring words that do not add any significant meaning to a sentence. E.g. “The”, “for”, and “with”.
Training
Once the data is processed, it’s used to train NLP models using machine learning algorithms. Training the algorithms involves feeding the model large data samples to increase the algorithms accuracy. This part of implementation helps ensure NLP frameworks generate the most appropriate human language response based on its input.
For instance, a customer support chatbot may be trained on past support tickets to learn how to handle future queries efficiently and effectively.
Deployment
After training, the model is tested and deployed — either as a standalone business tool or integrated into existing applications.
Using the above chatbot example, once deployed the model can handle live queries in real-time and continuously improve its performance and response capabilities.
What’s the Difference Between Natural Language Processing and AI?
The main difference between NLP models and AI is in their functionality. NLP models are used to process existing language-based data, with the intention of understanding and interpreting human language. Whereas generative AI intends to generate new content, such as text, photos, and video.
NLP models
NLP is most commonly used in applications that require in-depth understanding and processing of human language. It can also generate text based on the language data it’s trained on. You’ll find NLP models used in customer service automation, translation (such as Google Translate), and sentiment analysis for organisations to gauge how the public view their services/products.
Generative AI models
Generative AI, on the other hand, has more creative functionality, with many companies utilising the tool for content creation, as well as design and art. For example, ChatGPT is being used to support creative efforts, from writing articles and marketing copy to creative content that mimics human creativity.
Generative AI is also used in the healthcare industry to help researchers design new drugs by generating novel molecular structures that could result in effective treatments for patients.
Comments ( 0 )