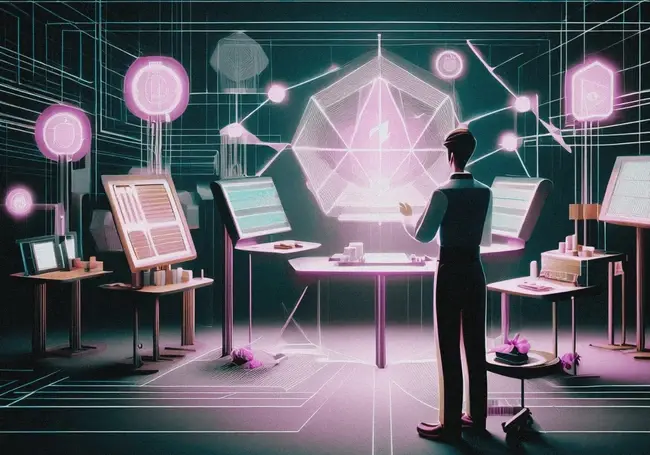
Scientists have come up with a new AI model to reduce the excessive energy consumption that goes into training large language models (LLMs).
They proposed an AI training system called – Perseus which reduces the “energy bloating” in LLMs.
Upon testing, the study found that the Perseus training model reduced the energy consumption by up to 30% when training large models including GPT-3 and Bloom “without any throughput loss or hardware modification.”
Now, envision a remotely working engineer who suddenly finds herself out at a mixer dinner and encounters a folk artist. Unsure how to continue the conversation which kicked off with basic information like habitat, profession, and how they find the city, the engineer suddenly feels stuck.
Fortunately or unfortunately, such a person in this day and age may resort to a generative artificial intelligence (gen AI) model like OpenAI’s ChatGPT for guidance.
But have you ever questioned how much energy actually goes into training and using these large language models (LLMs), such as the Open AI’s GPT series, Meta’s Llama Series, Google’s Gemini series, Anthropic’s Claude series, or the Mistral AI series?
According to The Association of Data Scientists, training GPT-3, which has 175 billion parameters, consumed an estimated 1,287 MWh (megawatt-hours) of electricity. This is roughly equivalent to the energy consumption of an average American household over 120 years.
Earlier this year, the International Energy Agency (IEA) reported that electricity demand for global data centres is likely to double from 2022 to 2026, vastly owing to the growth of AI.
The IEA estimated that global data centres consumed between 240 and 340 terawatt-hours (TWh) of electricity in 2022, which is about 1–1.3% of the world's total electricity demand.
Read: How AI Growth is Impacting Data Centre Demand
AI Costs ‘Outweigh Environmental Benefits’?
With AI driving the energy demand of data centres and causing increasing emissions each year, the new AI training model comes at a timely moment.
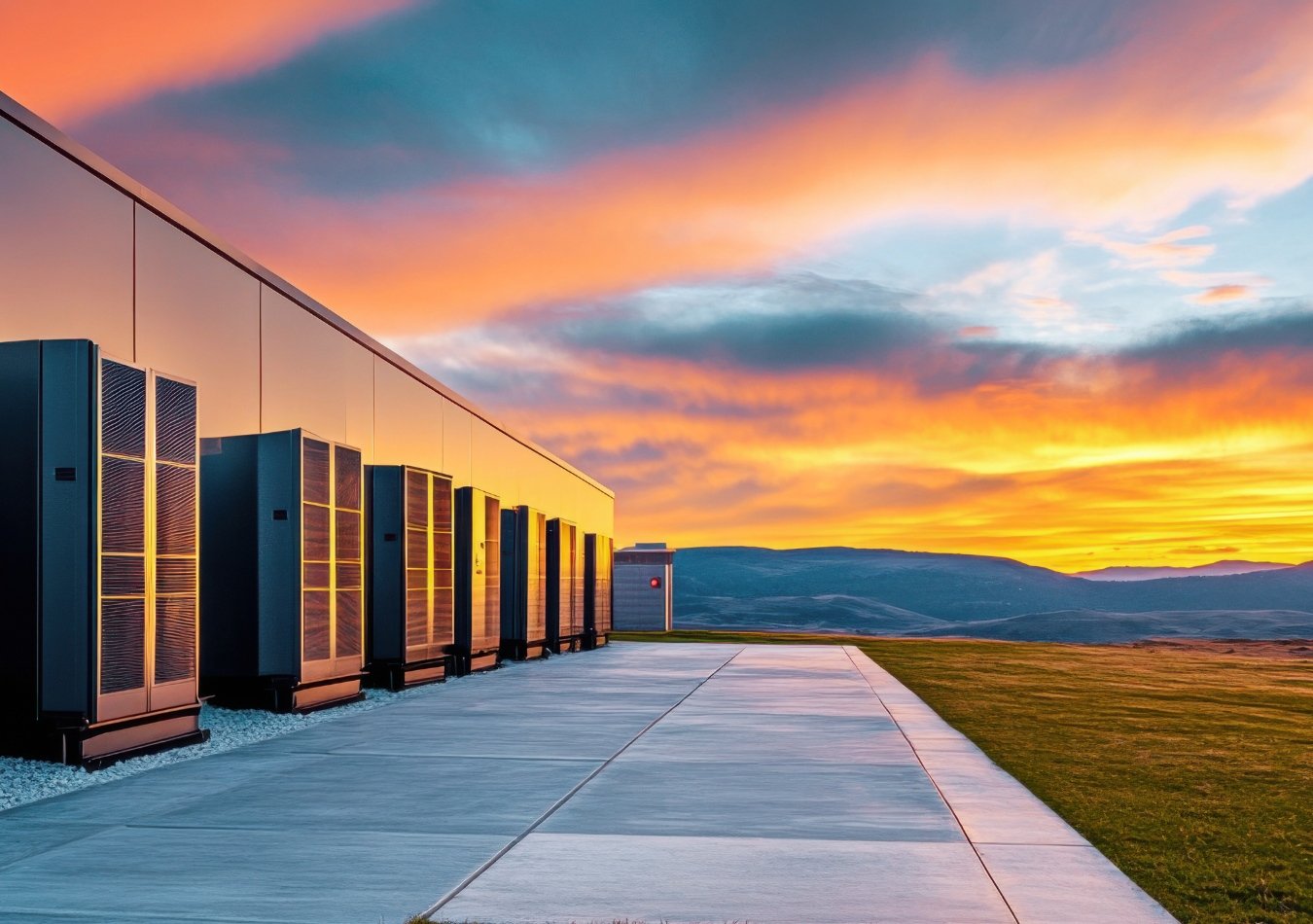
It may seem contradictory to many to create another AI model that reduces the energy bloating of LLMs. However, some experts believe that these costs could be outweighed by environmental benefits.
According to a statement by the University of Michigan, AI could be a “game changer” for fighting climate change by identifying ways to optimise supply chains and the grid, manage our energy needs, and improve research on climate change.
“Why spend something when there’s no point?” expressed Mosharaf Chowdhury, U-M associate professor and corresponding author of the study.
“We can’t keep building bigger and bigger data centres because we won’t have the power to run them. If we can reduce the energy consumed by AI, we can reduce AI’s carbon footprint and cooling requirements and allow for more computation to fit within our current energy constraints.”
Training such large-gen AI models on Graphics Processing Units (GPUs) – computer processors specialised for large data and graphics applications, is consuming huge amounts of energy and supplying loads of power for processing is the biggest challenge.
This increases the AI workload resulting in enormous energy consumption of data centres which ultimately contributes to carbon emissions.
//subhead//
Perseus Finds Energy-Efficient Processing Path
Current training approaches for LLMs like the GPT series are processed at top speed where processing units with lighter units complete their calculations before other processors.
Instead of speeding up training, it slows it because each processor has to finish its operations before to large AI models. Also, faster processes consume a lot more energy.
There’s another often overlooked factor – energy waste. This is linked to faulty hardware or network delays that slow down a single processor’s computing speed.
Perseus, the new AI training model spots a vital approach or a series of subtasks that take the longest to complete.
It then slows down processors that aren’t on the crucial path so all of them can complete their tasks at the same time, eradicating the unnecessary use of energy.
“Reducing the power cost of AI can have important implications for equitable AI access,” Chowdhury says.
“If a country doesn’t have enough power to run a big model, they might need to use services from far away or be stuck running smaller, less accurate models. This gap could further perpetuate disparity between different communities.”
The new AI training model was tested by training GPT-3 along with three other LLMs and a computer vision model.
Perseus is an open-sourced tool available as part of Zeus, a tool for measuring and optimising AI energy consumption.
Comments ( 0 )