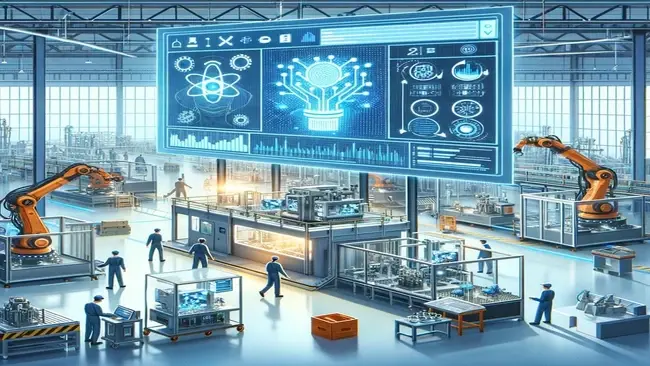
Production planning in manufacturing is a critical process that involves planning and organizing the manufacturing activities of a company. It is designed to ensure that production processes are carried out efficiently and effectively, with the goal of meeting customer demands and maximizing profitability. Key aspects of production planning include:
Demand Forecasting: Estimating the future demand for products, which is crucial for deciding the production quantity.
Resource Allocation: Determining the resources (like raw materials, workforce, machinery, etc.) required for production and ensuring their availability.
Process Planning: Deciding on the sequence of operations, the path that each product or part will follow through the production process.
Capacity Planning: Ensuring that the manufacturing facility has enough capacity (in terms of labor, equipment, and space) to meet the production goals.
Inventory Management: Managing the inventory of raw materials, work-in-progress, and finished goods to balance the costs with production and customer demand.
Scheduling: Developing a detailed timetable for production, specifying when each task should start and end.
Quality Control: Implementing systems to ensure that products meet quality standards and customer expectations.
Cost Control: Monitoring and controlling the costs associated with the production process.
Supply Chain Coordination: Coordinating with suppliers and logistics partners to ensure the timely delivery of raw materials and the shipment of finished products.
Continuous Improvement: Regularly analyzing the production process and implementing improvements to increase efficiency and effectiveness.
Effective production planning helps in reducing production costs, increasing productivity, and ensuring timely delivery of products, thereby playing a crucial role in the success of manufacturing operations.
AI in Production Planning
Manufacturers can leverage AI to optimize production planning in various ways. Here's a detailed look at the types of AI used, the necessary technology and data, the benefits to the business, and the key metrics to monitor:
Types of AI Used in Production Planning
Machine Learning (ML): For predictive analytics, demand forecasting, and process optimization.
Deep Learning: Advanced form of ML, useful in complex pattern recognition (e.g., quality control).
Natural Language Processing (NLP): For interpreting and acting on human language instructions or feedback.
Robotic Process Automation (RPA): For automating repetitive tasks in the production process.
Technology Needed
Data Analytics Platforms: Tools like TensorFlow, PyTorch for building and deploying AI models.
Cloud Computing Services: To store and process large datasets efficiently.
Internet of Things (IoT) Devices: Sensors and smart devices to collect real-time data from the production floor.
Advanced Robotics: For automating physical tasks.
Data Needed and Sources
Historical Production Data: Sales, inventory levels, production speed, and quality metrics.
Supply Chain Data: Supplier performance, lead times, and logistics.
Market Data: Customer demand patterns, trends, and forecasts.
Machine Data: From IoT sensors for real-time monitoring of equipment.
Impact of AI Across Production Planning Activities
Demand Forecasting and Capacity Planning
AI can play a significant role in demand forecasting to support production planning in manufacturing. Here's how:
Predictive Analytics: AI algorithms can analyze historical sales data, market trends, seasonal variations, and even global economic factors to make accurate predictions about future demand. This helps in planning production schedules more effectively.
Real-time Data Processing: AI systems can process real-time data from various sources like point of sale systems, online transactions, and social media trends. This allows manufacturers to adjust their production plans dynamically to meet actual market demand.
Real-time Monitoring and Control: AI systems can monitor production processes in real-time, making adjustments to optimize throughput and ensure that capacity is not underutilized.
Improved Accuracy: Traditional forecasting methods often rely on simple historical data and linear models. AI, on the other hand, can use complex algorithms, including machine learning models, which improve accuracy by learning from new data and identifying non-linear patterns.
Inventory Optimization: AI helps in maintaining optimal inventory levels. By predicting future demand accurately, manufacturers can reduce the costs associated with overproduction and storage, and also minimize the risk of stockouts.
Integrating External Factors: AI models can incorporate a wide range of external factors such as political changes, weather conditions, and economic shifts that could impact demand. This holistic view aids in more robust production planning.
Customization and Personalization: AI can analyze customer preferences and trends at a granular level, allowing manufacturers to tailor their products and production volumes more closely to specific market segments or even individual customer preferences.
Supply Chain Coordination: AI can enhance coordination across the supply chain, ensuring that suppliers are in sync with the manufacturer’s production schedules, which is critical for just-in-time manufacturing.
Risk Management: By forecasting potential disruptions in demand, AI helps in risk management. Manufacturers can prepare contingency plans for scenarios such as sudden demand spikes or drops.
Energy and Resource Management: In cases where manufacturing processes are energy-intensive, AI can help in planning production in a way that optimizes energy usage, contributing to both cost savings and environmental sustainability.
Scenario Planning and Simulation: AI can simulate various production scenarios, including changes in demand, supply chain disruptions, or new product introductions. This helps in understanding the impact on capacity and making informed decisions.
Human-Centric Decision Support: AI provides valuable insights and recommendations, but it also leaves room for human judgment and expertise, especially in situations where contextual understanding or strategic thinking is required.
The use of AI in demand forecasting enables more efficient, flexible, and responsive production planning in manufacturing, leading to cost savings, improved customer satisfaction, and a stronger competitive position in the market.
Resource Allocation and Production Scheduling
AI can play a significant role in resource allocation to support production planning in manufacturing. Here are some key areas where it can be particularly impactful:
Optimizing Production Schedules: AI can optimize production schedules by considering various constraints like machine availability, labor shifts, maintenance schedules, and supply chain logistics. AI algorithms can process vast amounts of data to optimize production schedules. They can consider various factors such as machine availability, labor shifts, maintenance schedules, and material supply, enabling efficient use of resources and minimizing downtime. This ensures that resources are used effectively, reducing idle time and increasing throughput.
Supply Chain Management: AI can improve supply chain visibility and predict potential disruptions. By analyzing data from various points in the supply chain, AI can help in making informed decisions about when and how much raw material to order, which suppliers to choose, and how to manage inventory levels effectively.
Quality Control: AI-driven quality control systems can help identify defects or issues in real-time, allowing for immediate corrective actions during the manufacturing process. This reduces waste, rework and ensures that resources are not spent on defective products.
Predictive Maintenance: AI can predict when machines and equipment are likely to fail or need maintenance. This proactive approach prevents unexpected downtimes and helps in better planning of maintenance schedules, ensuring that the production process is not disrupted.
Energy Management: AI can optimize the energy consumption of manufacturing plants by analyzing usage patterns and automatically adjusting systems for maximum efficiency. This not only saves costs but also reduces the environmental impact.
Customization, Flexibility and Personalization: In cases where manufacturing is moving towards mass customization, AI can help in dynamically allocating resources to produce customized products efficiently, without compromising on scale. AI systems can enable more flexible and customized production runs, adapting quickly to changes in product design or customer preferences.
Human Resource Allocation: AI can also assist in workforce planning, ensuring that skilled labor is available when needed and helping in training workers by identifying skill gaps.
Real-time Decision Making: AI systems can process large volumes of data in real-time, providing insights and recommendations for immediate adjustments in production planning and resource allocation. They can adapt production schedules in real-time in response to changing conditions such as order cancellations, new orders, supply chain disruptions, or equipment malfunctions. This flexibility enhances responsiveness and efficiency.
Collaborative Robots (Cobots): AI-enabled robots can work alongside humans, taking over repetitive or dangerous tasks, which optimizes the allocation of human resources to more critical areas.
Employee Training and Safety: AI can be used for training purposes, using simulations and predictive models to enhance worker safety and efficiency.
Benefits to the Business
Increased Efficiency: Optimized resource allocation and reduced wastage.
Enhanced Quality Control: AI-driven analytics for detecting and rectifying defects.
Demand Forecasting: Better prediction of market needs, leading to optimized inventory levels.
Cost Reduction: Streamlining operations and reducing manual interventions.
Important Metrics to Monitor
Production Yield: The ratio of usable output to total output.
Inventory Turnover: How quickly inventory is sold and replaced.
Equipment Efficiency: Machine uptime, maintenance needs, and performance.
Quality Metrics: Rate of defects or returns.
Supply Chain Efficiency: Lead times, supplier reliability, and transportation costs.
Implementation Considerations
Data Collection and Management: Establish a robust data infrastructure.
Model Development and Training: Create AI models tailored to specific business needs.
Integration with Existing Systems: Ensuring AI tools work seamlessly with current production systems.
Continuous Monitoring and Updating: AI models should evolve with changing data and market conditions.
Change Management: Implementing an AI-enabled production planning system in a business environment involves not only technical changes but also significant shifts in how people work and interact with new systems. Change management plays a crucial role in this transition and is vital for ensuring that the transition to an AI-enabled production planning system is smooth, effective, and leads to sustainable improvements in the organization’s operations. Here's how it can help:
Communication and Awareness: Change management helps in communicating the purpose and benefits of the new AI system to all stakeholders. By creating awareness, it reduces resistance and builds a positive perception of the change.
Training and Skill Development: Change management ensures that employees are adequately trained to use the new system. This includes not just technical training but also understanding how to interpret and act on the insights generated by AI.
Leadership Alignment and Sponsorship: Effective change management involves getting buy-in from leadership and ensuring there is a sponsorship for the change. Leaders play a key role in motivating and guiding their teams through the transition.
Cultural Change: Introducing AI into production planning can significantly alter the workplace culture. Change management helps in reshaping the culture to be more accepting of AI and data-driven decision-making.
Managing Resistance: Resistance to change is natural. Change management provides strategies and tools to identify, understand, and address resistance from employees, helping them transition smoothly into the new way of working.
Process Redesign: AI implementation often requires changes in existing processes. Change management helps in redesigning processes to integrate AI effectively, ensuring that the system complements and enhances existing workflows.
Feedback Mechanisms: Establishing channels for feedback helps in understanding how the change is being received and what adjustments may be necessary. Change management facilitates the creation of these feedback mechanisms.
Continuous Improvement: Change management doesn't stop at implementation. It involves monitoring the ongoing effectiveness of the change, ensuring continuous improvement and adaptation as necessary.
Risk Management: Implementing AI systems comes with risks, including technical failures, data inaccuracies, and human errors. Change management helps identify, assess, and mitigate these risks.
Sustainability of Change: Finally, change management ensures that the new AI-enabled system is not just implemented but also sustained over time. This includes regular updates, maintenance, and ongoing support.
Challenges and Considerations
Implementing an AI-enabled production planning system in manufacturing comes with a variety of challenges and considerations:
Data Quality and Availability: For AI systems to be effective, they require large volumes of high-quality, relevant data. In manufacturing, gathering such data can be challenging due to inconsistent data collection practices, legacy systems, or data silos.
Integration with Existing Systems: Many manufacturing facilities use a mix of old and new technologies. Integrating AI solutions with these varying systems without disrupting ongoing operations is a significant challenge.
Cost and ROI Concerns: The initial investment for AI technology can be substantial. Businesses must consider the return on investment, including cost savings and efficiency gains, to justify the expense.
Scalability and Flexibility: The AI system should be scalable to adapt to the changing needs of the business and flexible enough to handle different types of manufacturing processes and products.
Reliability and Maintenance: AI systems must be reliable and consistently available. Regular maintenance and updates are necessary to ensure they continue to function optimally.
Security and Privacy: Manufacturing data can be sensitive. Ensuring the security and privacy of data within AI systems is crucial to protect intellectual property and comply with regulations.
Regulatory Compliance: The manufacturing sector is often subject to strict regulatory standards. AI systems must comply with these regulations, which can vary by region and product type.
Ethical Considerations: There can be ethical implications, such as the impact on employment due to automation, biases in AI decision-making, and ensuring AI is used responsibly.
Technical Expertise: Developing and maintaining AI systems requires a high level of technical expertise. Manufacturers must either develop this expertise in-house or rely on external partners.
Customization and Adaptability: Manufacturing processes can vary widely. An AI system might need significant customization to suit specific processes, products, or business goals.
Outcome Predictability and Explainability: It's important for the AI system to not only make decisions but also provide explanations for those decisions, especially in scenarios where accountability and traceability are important.
Each of these challenges requires careful consideration and planning to ensure the successful implementation of AI in production planning in the manufacturing sector. AI can significantly enhance production planning by making processes more efficient, predictive, and responsive to market demands. The key lies in the effective use of data, the right technology stack, and a clear focus on relevant business metrics.