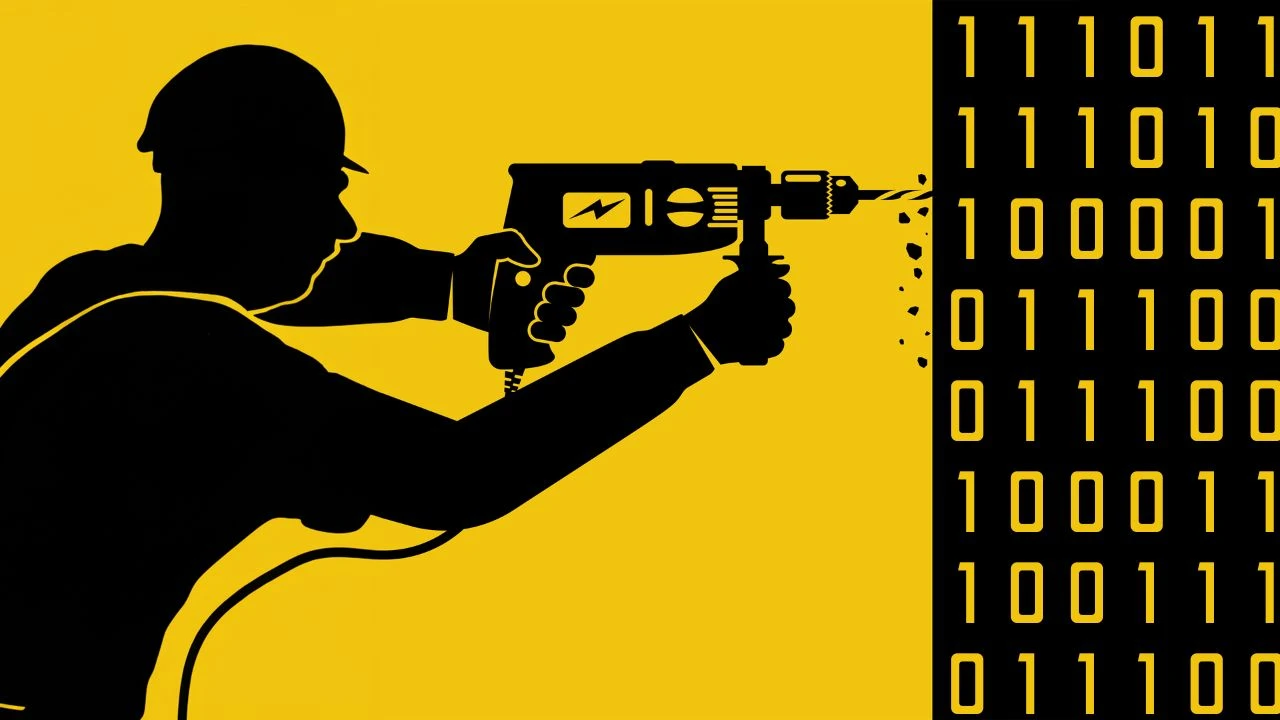
Businesses are responsible for more data than ever, however, over 70% of this data is left unused due to it being so difficult to organize- that’s where data warehouse tools come in.
Data warehouse tools are applications or platforms that streamline the entire data warehousing process. Choosing the right data warehouse tool depends on factors like the volume and variety of data, budget, technical expertise, and desired functionalities.
What is a data warehouse?
A data warehouse is a central location where businesses store information for analysis purposes.
The often large archive gathers data from multiple sources within a company such as sales figures, customer information, or website traffic.
This data is then organized and formatted in a way that makes it easy for analysts to identify trends and patterns.
Data warehouses are different from regular databases. They typically hold historical data to allow users to see how things have changed over time.
Data warehouses are designed for analysis, so they are optimized for complex queries and reports.
Data in a warehouse is usually cleansed and transformed to ensure consistency and accuracy.
Read: What is Data Warehousing
Data lake vs data warehouse
A data lake offers more comprehensive storage than a data warehouse. A data lake can hold any type of data, including raw, unstructured data like social media posts, sensor readings, or video files.
In a data lake structure is applied when the data is analyzed, whereas in a data warehouse structure is applied when it is stored.
The best data warehouse tools
Choosing the right data warehouse tool depends on factors like the volume and variety of data, budget, technical expertise, and desired functionalities.
In this article, we’re counting down the top ten data warehouse tools that streamline the entire data warehousing process.
Panoply
Panoply stands out as a unique data warehousing tool because it combines features of both ETL (Extract, Transform, Load) and data warehousing into a single platform. Unlike traditional data warehouses that rely on separate ETL tools, Panoply streamlines the data management process.
Panoply offers data analysis functionalities within the platform, allowing users to explore and visualize data without needing additional BI tools. They offer a variety of pre-built connectors that simplify data extraction from various sources, eliminating the need for complex coding typically required in ETL tools.
Crucially, Panoply boasts a user-friendly interface that minimizes the need for coding expertise, making it accessible to a broader range of users within a business.
Oracle
Oracle is a major player in the data warehousing space, offering both on-premise and cloud-based solutions. Their data warehouses are known for their ability to handle massive datasets and deliver fast query performance. The solutions offer a range of built-in features for advanced analytics, including machine learning and in-memory processing capabilities.
Oracle Autonomous Data Warehouse (ADW) offers a fully managed cloud-based data warehouse solution. This eliminates the need for extensive IT infrastructure management, allowing businesses to focus on data analysis. Their cloud solution offers self-service functionalities for provisioning, configuration, scaling, and maintenance.
IBM DB2
IBM DB2 Warehouse is built for the cloud, ensuring scalability, elasticity, and ease of deployment in cloud environments. Db2 excels at handling both transactional workloads (OLTP) and analytical workloads (OLAP) within the same platform.
DB2 goes beyond traditional relational data. It can store and manage structured, unstructured, and semi-structured data, providing flexibility for diverse data sources. DB2 is designed to handle large datasets and complex queries efficiently, making it suitable for growing businesses.
Dremio
Dremio takes data warehousing to the next level with its data lakehouse. Dremio builds upon existing data lakes, which store large amounts of raw and processed data in various formats. Instead of moving data to a separate data warehouse, Dremio allows querying data directly in the lake.
Dremio prioritizes fast and efficient querying of data in the data lake, eliminating the need for complex data transformation processes typically associated with data warehouses.
Snowflake
Snowflake has carved a niche for itself in the data warehousing space by offering a unique cloud-based solution. Designed from the ground up for the cloud, Snowflake offers exceptional scalability and elasticity. You can easily scale compute resources up or down to meet your data processing needs without worrying about underlying infrastructure.
Snowflake separates data storage and compute resources. This allows you to store vast amounts of data without incurring compute costs when the data isn't actively being analyzed.
Teradata Database
Teradata Database is a popular data warehousing tool, know for its capability to handle massive datasets and complex analytical workloads. Unlike traditional data warehouses that rely on a single server, Teradata distributes data and processing tasks across multiple nodes, enabling parallel processing and exceptional performance for large datasets and complex queries.
Each Teradata node operates independently with its own CPU, memory, and storage. This eliminates bottlenecks and ensures scalability as you add more nodes to handle growing data volumes. Teradata is specifically designed for data warehousing needs. It excels at storing and managing historical data for in-depth analysis.
Google BigQuery
Google BigQuery stands out for its unique approach to data warehouses. Unlike traditional data warehouses that require managing servers and infrastructure, BigQuery is entirely serverless.
Built on Google's robust cloud infrastructure, BigQuery seamlessly scales to handle petabytes of data. BigQuery goes beyond structured data. It can store and analyze semi-structured and unstructured data formats like JSON, allowing you to gain insights from a wider range of data sources. It also integrates seamlessly with Google Cloud Machine Learning tools.
Informatica
Informatica isn't just a data warehouse solution, it's a broader software company specializing in enterprise cloud data management and data integration. Informatica PowerCenter, is a powerful ETL tool that streamlines the process of extracting data from diverse sources like databases, applications, and flat files. It can quickly transform data by cleaning, filtering, and formatting it to ensure consistency and meet the specific requirements of the data warehouse schema. The software can then load the transformed data into the data warehouse for analysis.
Informatica's tools prioritize data quality during the transformation stage, ensuring clean and reliable data in your data warehouse.
Databricks Lakehouse
Databricks Lakehouse is an architectural approach to data management offered by the Databricks Data Platform. It combines elements of traditional data lakes and data warehouses to provide a more flexible and cost-effective solution for storing and analyzing data. The ability to store and analyze all data types in a single platform provides greater flexibility and agility for evolving data needs and analytics requirements.
Databricks Lakehouse is built on open-source technologies like Apache Spark, Delta Lake, and MLflow. This ensures open standards, avoids vendor lock-in, and fosters a broader developer community. The cloud-native architecture of the Databricks platform allows for seamless scaling to handle growing data volumes.
Amazon Redshift
Amazon Redshift is a cloud-based data warehouse service offered by Amazon Web Services (AWS). It's designed to handle large datasets and complex analytical workloads, making it a valuable tool for businesses that need to extract insights from vast amounts of data.
Redshift leverages MPP architecture. This means it distributes data and processing tasks across multiple nodes working in parallel. This parallel processing enables Redshift to handle massive datasets efficiently and deliver fast query response times, even for complex analytical tasks. It also allows you to easily scale your data warehouse up or down based on your needs. You can add or remove nodes to accommodate growing data volumes or fluctuating processing demands. It can also integrate seamlessly with other AWS services, such as Amazon S3 for data storage and Amazon QuickSight for data visualization.
Comments ( 0 )