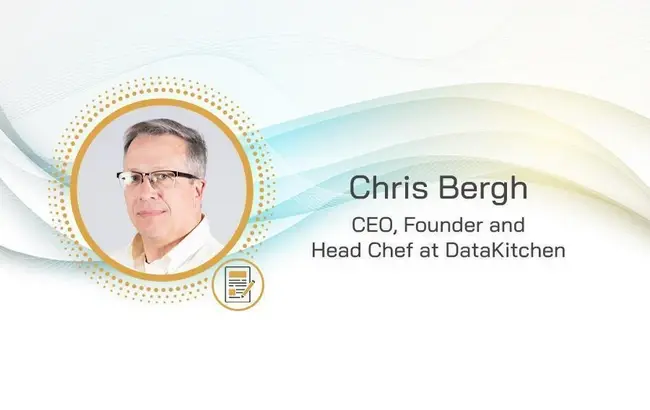
“If you can’t stand the heat, keep out of the kitchen.”
For three IT professionals some 20 years ago, this saying became their reality. While working at a small company that offered data analytics services, they found that they were constantly tackling complex data pipelines, which led to embarrassing errors and an inability to meet customer demands. Worse still, they were discouraged from attempting any experimentation to combat these challenges, leaving the trio at an impasse that could not be overcome.
Enough was enough, they thought. There had to be another way. Soon, the trio’s path of action became clear: they needed to get out of that kitchen, but into a new one, a better one. One that would see analytics teams thrive and customers more satisfied.
Like another old adage goes, “if you want something done, you need to do it yourself.” That’s exactly what the did; they departed from the small company together to start one of their own, with the aim of freeing data professionals from the challenges that the trio once faced. After closely observing the same challenges in other industries (namely software development and manufacturing), the three were able to take the tools and methodologies from these fields and fashion a hybrid approach, which they would call ‘DataOps’.
Today, that same trio are the proud founders of DataKitchen, a company which spearheads the DataOps approach. In particular, DataKitchen is on a mission to solve the analytic industry’s data problems while ensuring that professionals in the field are no longer subject to the same issues and embarrassing errors.
The entire DataKitchen brand is ingeniously based on the culinary arts. The kitchen metaphor extends across their offering, which encompasses their ‘recipes’, ‘ingredients’, and ‘order’ capabilities, to name a few. Above all kitchen parallels is the continued messaging that analytics is a team sport, much like cheffing is too.
At the helm of the company is Chris Bergh, CEO, Founder, and Head Chef. Chris has been instrumental in championing the company’s unique branding and culture, as well as its commitment to empowering and celebrating data professionals. With our interest well and truly piqued, we grilled (sorry) Chris to find out more about DataKitchen’s culture and how the company came to be.
Thank you for taking the time to speak with us, Chris! Firstly, it’d be great to get a bit of background on the technical challenges you were facing at your previous company and how DataKitchen has addressed them. What can you tell us?
I spent many years in unpleasant circumstances. I managed data teams that battled a constant barrage of quality problems, missed milestones, depleted budgets and angry customers, not to mention all of the missed family time. This is still the day-to-day experience of many data professionals. In those days, when I came to work in the morning, I felt myself wishing I was somewhere else. At some point, I just felt like there has to be a better way – a way to make being a data professional fun again.
In the DataKitchen DataOps Cookbook, you mention that, at your previous company, employees were discouraged from trying new tools and thinking innovatively. What do you think the cultural and business impacts are of quashing experimentation?
In business, everyone wants to speed up the time it takes to respond to continually evolving threats and opportunities. In data analytics, business agility can be expressed using a metric called “cycle time.” We define cycle time as the period elapsed between the proposal of a new idea (or a new question) and the deployment of finished analytics. The time that the data team waits for access to a new data set lengthens cycle time. The fire drill that results when data operations go offline (putting new development on hold until the problem is solved) affects cycle time. The time required to build a development environment for a new data project impacts cycle time. Everything that lengthens cycle time handicaps innovation and decreases competitiveness.
Consider an example data team producing analytics for a VP of Marketing. Imagine the VP has an idea to feed user search history to the algorithm that displays product offers to potential customers. A data analytics team with an average cycle time of ten weeks comes back in a couple of months with a prototype model. As we all know, “version 1.0” of a great application doesn’t always work as hoped. Perhaps the VP then asks to add both search history and customer ratings into the algorithm. After another ten weeks, the data team delivers the second iteration. At this pace, the VP can only propose several ideas per year.
What happens when analytics cycle time is reduced, for example, through DataOps automation, to a single day? Now the VP can make several requests per week. The marketing team can brainstorm ideas. Working closely together, marketing and data analytics iterate toward more effective algorithms. With enough iterations, they’ll eventually stumble onto a blockbuster idea. We’ve seen it happen many times.
How do you think your previous experience has shaped the way you lead DataKitchen?
Like many other data professionals, I lived in a world of long cycle times, constant fire drills due to errors and lots of pressure and unpleasantness. I began to read books on quality: W. Edwards Deming, lean manufacturing, Total Quality Management, Six Sigma and more. I began to understand how these tried and true concepts are being applied in software development in the form of Agile Development and DevOps. We started very humbly with a quality circle and working from an issues list tracked using a spreadsheet. Eventually, I realized that data analytics pipelines are quite similar to manufacturing operations. You start with raw materials (data), progress through a series of processing and transformation steps and output finished goods (charts and graphs). We looked at the statistical process control used in automotive manufacturing and saw that we could use those same techniques to improve data quality. Eventually, all of these ideas were put together under one umbrella term, “DataOps.” The other DataKitchen founders and I felt strongly that DataOps could help data organizations address their quality and cycle time challenges and perhaps restore a little sanity to their work lives.
Data teams are arguably the unsung heroes of businesses today. How does DataKitchen help to empower them and drive their success?
It’s important to understand the perspective of business leaders in context. In our DataOps survey, 97% of respondents reported one or more data analytics errors per month. 30% of companies have an error every few days. It’s not hard to imagine why a CEO who receives charts and graphs with errors on a regular basis expresses a lack of enthusiasm about the data analytics team.
When data analytics look wrong or business-critical systems go offline, many enterprises depend on key personnel to swoop in and save the day when things go wrong. Data analytics teams work long hours to compensate for the gap between performance and expectations. When a deliverable is met, the data analytics team is considered heroes. However, heroism is a trap. Heroes give up work-life balance. Yesterday’s heroes are quickly forgotten when there is a new deliverable to meet. The long hours eventually lead to burnout, anxiety and even depression. Heroism is difficult to sustain over a long period of time, and it ultimately just resets expectations at a higher level without providing additional resources.
DataKitchen was founded on the principle that heroism is not a management strategy. Data organizations need to create robust, repeatable processes that rapidly deliver high-quality analytics to users. DataOps automation delivers on this goal as it equally empowers the most senior data science guru and the entry-level data analyst. What truly matters for data organizations is workflow efficiency as measured in cycle time and a low error rate. These metrics power innovation by freeing up the data team from non-value-add tasks to focus their considerable talents on the enterprise’s most urgent top and bottom-line challenges.
The kitchen metaphor is a perfect fit for what the company offers, and we love how it extends beyond just the brand name. In fact, probably one of our favourite things about the company is that you’re known to wear chef uniforms at events! What kind of reaction do you guys get?
We have a lot of fun with it. Occasionally someone tries to order something to eat. The next time you see us at a trade show, please stop by and get a DataKitchen wooden spoon.
If anything is clear, it’s that DataKitchen has a great company culture. What kind of cultural values do you encourage and, in turn, what is life at the business like?
We practice what we preach. DataKitchen is a DataOps enterprise. We published the principles that guide our company in a document called the DataOps Manifesto, which has to-date been signed by over 7,000 people. With DataOps automation, we’ve been able to establish a robust, repeatable workflow for creating, maintaining and monitoring analytics with virtually zero errors. DataOps keeps everything running smoothly.
We love data science, and we equally love being able to predictably finish work at 5pm to enjoy the other parts of our lives. We thank DataOps automation for putting the enjoyment back into data analytics and enabling us to spend most of our work time being creative.
And just for fun: when you’re in the kitchen at home, what’s your signature dish?
When we founded DataKitchen, we adopted the kitchen metaphor for data analytics because, after all, cooking up charts and graphs requires the right ingredients and recipes. We wrote The DataOps Cookbook which is first and foremost a serious and thorough discussion of methodologies and tools that reduce data analytics cycle time while improving quality. In keeping with our relaxed corporate culture, we also included some real recipes that DataKitchen employees contributed. My entry was “DataOps Ribeye,” which is my own personal “Tyrannosaurus Rex” method of grilling steaks. I hope you’ll try it.
Comments ( 0 )