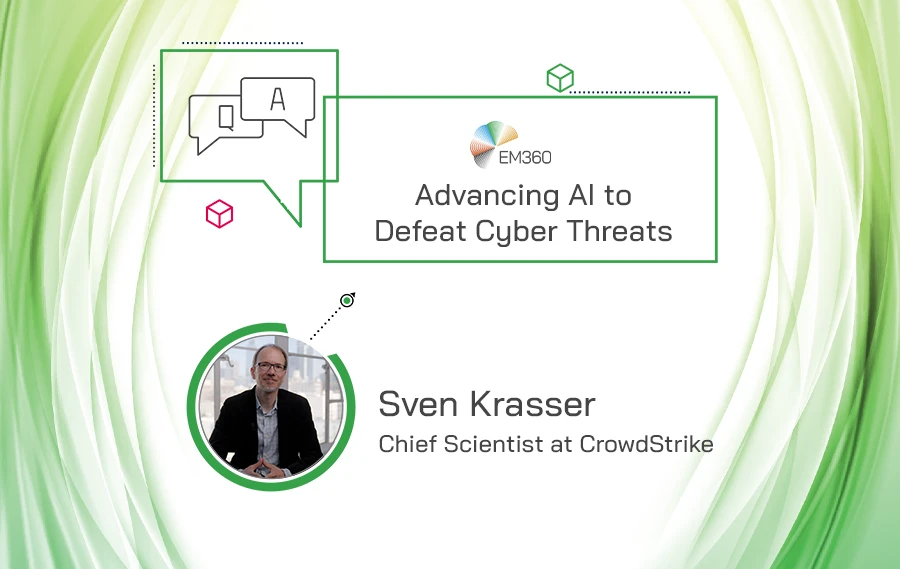
The world as we know it is in the midst of a cyber-pandemic, and whilst businesses struggle to fight this wave, there are unsung heroes behind the scenes who are helping businesses to stay protected. Introducing Sven Krasser, Senior VP, Chief Scientist at CrowdStrike. Sven is a long-standing player in the endless game of cybersecurity ‘cat and mouse’. A lead inventor of numerous key patented network and host security technologies, plus the author of various publications on AI/ML in cybersecurity, he is undoubtedly pioneering the field and supporting organisations with their security strategies. In this week’s Q&A, we sit down with Sven to discuss his role within cybersecurity and the use of advanced AI to defeat cyber threats in the enterprise.
1. To begin, I can’t ignore your exceptional title. What does it take to be a Chief Scientist, especially at such a senior level within an AI-cybersecurity organisation?
Both cybersecurity and the field of AI are moving at a rapid pace, so the first order of business is staying ahead of the game. That means keeping an eye on new threats and attacks while also determining how new research in AI can help to thwart these. Research alone doesn’t stop any breaches, so I also work closely with teams across the company to integrate those new technologies we’ve developed into our products
2. What lessons have you learnt from being on the frontline of the globe’s ongoing wave of attacks?
Cybersecurity is an ongoing battle and not a problem that has been or will be solved for good -- contrary to frequent claims. Behind every cyberthreat and every attack is a human adversary with an agenda and often with strong pecuniary incentives. As long as there are motivated adversaries, we will see them persistently searching for means to evade detection.
Therefore, it is not only important to have a high detection efficacy. It is also important to have the capabilities to spot the remaining, entrenched threats that inevitably make it through some defences. At CrowdStrike, we have a strong focus on avoiding such silent failure and to ensure that we have no blind spots, we have threat hunters which, through human analysis on a 24/7/365 basis, are able to relentlessly hunt for anomalous or novel attacker tradecraft designed to evade other detection techniques.
3. Why should the enterprise view and implement AI as a foundational tool in fighting the cyber-pandemic we’re facing today?
In today’s world, effective cybersecurity requires working with larger and larger data volumes. In addition, data is getting more and more complex. These shifts are especially evident in the opportunities that cloud computing is offering us as defenders. In the case of CrowdStrike, that amounts to over a trillion new events that our cloud platform is analysing every day.
While such contemporary security data sets offer a unique global vantage point into the threat landscape, they also go beyond human cognition and cannot be effectively reasoned about by mere manual means. AI allows us to make sense out of this plethora of information: it can process more data of higher dimensionality in shorter amounts of time and as a result, produce output that is more actionable to us humans.
4. Earlier this year, you wrote an article on the SUNSPOT malware that was used to insert backdoor into SolarWinds. Is file-based machine learning the key to detecting and preventing these types of threats?
No, any file-based technique alone, whether AI-based or not, is not enough. While this example shows how an AI classifier can outperform signature-based approaches by detecting a completely novel, previously unseen threat, a defence based on only analysing files is hobbled in multiple ways.
First, there is an inherent asymmetry in resources. An adversary can craft a malware file over the course of months, monitoring its evasive properties and adjusting it as necessary. A security product has a sub-second time window to render a decision when it evaluates that file.
Second, the contents of a file are completely under control of the adversary. Adversaries can stick whatever bytes they please into it. When you play chess against an opponent, you don’t want to give them free rein in setting up the board. Instead, we want to introduce hard constraints the attacker cannot get around, e.g. because they are required to access specific functions of a system to accomplish their goals. Going beyond file contents and including the broader execution context or looking at aspects like provenance provides for a significantly more robust detector. At CrowdStrike, we accomplish this by leveraging our ThreatGraph database, for example.
Third, many attacks simply don’t rely on malware files. So if one is just looking for those, one is putting blinders on and missing the bigger picture. These file-less attacks are often called “living off the land” attacks because the adversary uses whatever tools are already available on the victim’s machine. Therefore, we do not only look for artefacts on disk or in memory, so-called Indicators of Compromise. We also look for Indicators of Attack, i.e. the behaviours an adversary is forced to expose to be able to achieve their objectives.
5. Some security analysts argue that AI in cybersecurity isn’t a panacea because it still has a fair bit of maturing to do before it can alleviate human talent. What are your thoughts on this?
AI is not a panacea, but that is not due to a lack of maturity. AI is best understood as table stakes. A successful security solution will need to leverage AI as part of its data pipeline to stay competitive. But that, in turn, does not mean that AI can comprehensively deliver on every feature a security solution needs to provide.
No, AI is not a silver bullet; the undue reliance on an AI monoculture brings its own risks. AI introduces a new, distinct attack surface into security products. For example, AI algorithms are susceptible to data poisoning attacks, i.e. the introduction of training data by an adversary that is designed to create blind spots in the final model. Furthermore, adversaries can use their own AI to defeat the AI in security products. This field of research is called Adversarial Machine Learning. So-called adversarial examples can be created by adding carefully crafted noise to a malicious artefact, which is then no longer detected by AI models. Systems can be designed to make the creation of adversarial examples harder, but there is no way to completely eliminate that weakness.
Companies that successfully apply AI are well-aware of its inherent limitations and the trade-offs that must be made. Effective cybersecurity approaches require handling of cloud-scale data, but without AI processing of data at that scale is impractical. Therefore, using AI is necessary but requires more foresight than simply jamming it into the data pipeline. Be wary of solutions that completely avoid AI as well as those that are fully reliant on AI.
6. How is CrowdStrike building patterns to support frontline security and helping its clients to stay one step ahead of cybercriminals?
At CrowdStrike, our cloud processes over one trillion events per day. This volume of data gives us unprecedented insights into the global security landscape—observing emerging threats across companies, organisations, or borders. To be able to interpret this volume of data, we require powerful tools such as AI, but we also utilise other tools such as Indicators of Attack, which allow us to prevent threats by describing the underlying causal relationships.
Our use of AI allows us to make rapid autonomous decisions, for example on the end host. We also leverage models that analyse larger volumes of data in the cloud where it is feasible to conduct complex computations on big data and where we can easily look at the bigger picture, going beyond a single host or a single corporate network. This is what we call “the fast loop.”
Outside of detections, we also use AI to surface unusual patterns in the data, which we then subject to further review. The Falcon Overwatch team, among others, analyses such flagged data and provides an expert disposition, which we feed back into our AI algorithms. Over this route, our AI models receive a constant stream of feedback about where they were successful and where we spotted novel attacks by other means. The AI learns from this feedback and incorporates it into future detections. We call this part “the long loop.”
This setup is deliberate. Every day we benchmark how well our AI detectors work, and every day we expand the pool of ground truth that we can use to train a better AI. Humans constantly translate their expertise into data we can feed into our AI systems, e.g. when analysing the puzzle pieces of a novel attack. Access to a large pool of high-quality ground truth is a necessity for training effective AI detectors, and we have set up our system so that every day we are guaranteed to do better than the day before.
Comments ( 0 )