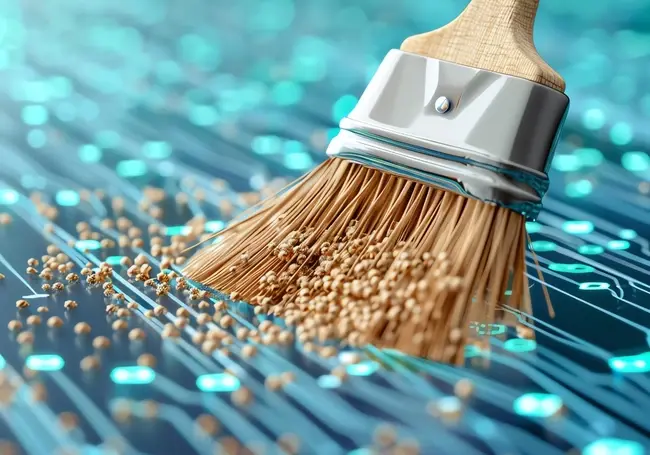
Data has never been more important for organizations looking to make smarter decisions. More knowledge about your customer’s preferences and needs allows you to understand and predict consumer behaviour, spot future trends, and drive more conversions.
But many data teams still struggle to find a structured approach to managing their data, making it difficult to see if data is complete, up-to-date and reliable – especially if they possess huge volumes of unstructured data,
When organisations have more raw data than they know what to do with, they often miss out on leveraging the valuable data they possess despite it being crucial to the success of their business. This is where data enrichment can help.
This article tells you everything you need to know about data enrichment, including what it is and how it can help you to make smarter business decisions.
What is data enrichment? Definition
Data Enrichment is the process of enhancing data with additional, relevant information from external sources. It works by taking raw, incomplete, or unrefined data and enhancing it with additional information from external or internal sources to provide more context and depth.
This helps improve the quality, completeness, and accuracy of your dataset, making it more valuable for analysis, decision-making, and other applications.
By combining your existing data with external information, you can gain deeper insights, identify trends, and make more informed decisions. For example, if you have a customer database, you might enrich it with demographic data, social media activity, or purchase history to better understand customer preferences and behaviors.
Data enrichment can be automated or manual, depending on the size and complexity of the dataset. In many cases, enrichment involves integrating third-party data sources, such as APIs from data providers, public databases, or social media platforms, with internal data.
This process often relies on technology and tools like data integration platforms, APIs, machine learning algorithms, and data management systems. Automation is also often used to handle large datasets efficiently while maintaining data accuracy and integrity.
Steps of Data Enrichment
1. Data Collection and Identification
The first step in data enrichment is identifying the data that needs to be enriched. This could be customer data, product information, or operational metrics. The data is collected from internal databases, spreadsheets, CRM systems, or other repositories. Once the data is identified, the specific gaps or areas for enrichment are pinpointed.
2. External or Internal Data Integration
After identifying the gaps, the next step is to source the additional data required for enrichment. This can come from internal sources, such as other departments within the organization (e.g., sales, marketing, or support), or external third-party providers, like data brokers, public records, or APIs. For instance, a business might integrate geographic data from a mapping API, social media activity from social platforms, or consumer demographics from a third-party data provider.
3. Data Matching and Validation
Once the additional data is sourced, it is matched with the existing records to enrich them, which involves ensuring that the correct information is linked to the corresponding data points. Data validation is crucial at this stage to ensure accuracy and avoid erroneous or duplicate information, and automated processes like fuzzy matching algorithms or data cleansing tools are often used to ensure that the enrichment process is precise and accurate.
4. Data Standardization and Formatting
After the data has been successfully matched and validated, it is standardized to ensure consistency. This involves converting the data into a common format or structure that fits the existing data framework to help make the enriched data easier to analyze and integrate with existing business processes.
5. Application of Enriched Data
Once enriched, the data is ready to be used across various applications. Businesses can leverage it for customer segmentation, personalized marketing campaigns, predictive analytics, or operational improvements. For example, enriched customer profiles may allow a company to create highly targeted marketing campaigns or improve customer service by offering tailored solutions based on a customer's historical behavior or preferences.
Why is data enrichment important?
Data enrichment is important because it transforms raw, incomplete, or unstructured data into more meaningful and actionable information. Enriched data gives businesses deeper insights into their operations, customers, and market trends, enabling them to make more informed decisions.
Without enrichment, data may lack the context or detail necessary to uncover useful patterns, leaving companies at a disadvantage. For example, customer data with just names and emails offers limited insight, but when enriched with demographic, geographic, or behavioural data, it enables better segmentation, more personalized marketing, and a deeper understanding of customer needs and preferences.
Another key reason data enrichment is crucial is its role in improving data accuracy and completeness. Incomplete or outdated data can lead to incorrect assumptions, flawed decision-making, and wasted resources. By enhancing the data with up-to-date and relevant information, businesses can reduce redundancies, eliminate errors, and ensure that their data is reliable and fit for purpose.
Data enrichment also helps improve customer experience and engagement. By having a richer understanding of customers’ preferences, habits, and behaviors, businesses can deliver more personalized services and communication. This leads to better customer satisfaction, higher retention rates, and increased brand loyalty.
Data Enrichment Best practices
1. Define Clear Objectives
Before starting the data enrichment process, it’s crucial to have a clear understanding of why the data is being enriched and what business goals it aims to achieve. Whether it's for improving customer segmentation, enhancing personalization, or optimizing operational efficiency, defining clear objectives ensures that the enrichment process aligns with business needs. This clarity helps in selecting the right data sources and enrichment methods that are most relevant to the end goals.
2. Prioritize Data Quality
High-quality data is foundational to successful data enrichment. Before enriching, ensure that the existing data is clean, accurate, and up-to-date. Implement data cleansing techniques to remove duplicates, errors, and inconsistencies. In the enrichment process, prioritize data sources known for their reliability and accuracy. Poor-quality or outdated data can lead to flawed insights and business decisions, defeating the purpose of data enrichment.
3. Choose Relevant Data Sources
Data enrichment should be conducted using relevant and credible external or internal sources. Ensure that the sources selected for enrichment provide data that is accurate, trustworthy, and aligned with the specific needs of your organization. For instance, if you're enriching customer profiles, use reputable third-party data providers for demographic or behavioral data. If location-based insights are required, integrate geographic data from reliable sources like public datasets or specialized APIs.
4. Ensure Data Privacy and Compliance
Data privacy and compliance with regulations like GDPR (General Data Protection Regulation) and CCPA (California Consumer Privacy Act) are critical in data enrichment. Organizations should ensure that the data they collect and enrich, especially personal data, complies with legal standards.
This includes obtaining proper consent from users for the use of their data, ensuring secure handling of sensitive information, and respecting users' rights to privacy. Data enrichment efforts must be transparent and avoid enriching data with sensitive information that could lead to violations of privacy laws.
5. Leverage Automation and AI
Enrichment can be a complex process, especially when dealing with large datasets. To scale efficiently, it’s important to use automation tools and AI-based solutions for data integration, cleansing, and matching. Machine learning algorithms can assist with intelligent data matching, filling in gaps, and identifying patterns. Automation minimizes manual effort, reduces errors, and enables faster data enrichment, while AI can enhance the accuracy of the enriched data by learning from existing patterns.
Data Enrichment vs Data Cleansing: What’s the difference?
Data enrichment and data cleansing are both essential processes in data management, but they serve different purposes and focus on distinct aspects of data quality. While data cleansing improves data quality by fixing errors and inconsistencies, data enrichment enhances data value by adding new and relevant information to make it more informative and actionable.
Both processes are critical for ensuring that data is usable and meaningful for business operations and analytics.
Data cleansing removes incomplete records, fixing typographical errors, standardizing data formats, and eliminating outliers or inconsistencies that could skew analysis. For example, cleansing a customer database might involve removing duplicate entries, correcting misspelled names, or ensuring phone numbers are in a standardized format. Ultimately, data cleansing focuses on improving the quality of the existing data by making it more reliable, accurate, and consistent for further use.
On the other hand, data enrichment goes beyond cleansing by adding additional information to existing datasets. The goal of enrichment is to enhance the dataset with more context or detail, often by incorporating external data sources.
Examples of data enrichment
1. Customer Demographic Enrichment
A company might start with basic customer information, such as names and email addresses, but by enriching the data with demographic attributes – such as age, gender, income, and occupation – they can create more detailed customer profiles.
For example, a retailer could use this enriched data to better segment its audience and target different customer groups with personalized offers or marketing campaigns.
2. Geolocation Enrichment
Businesses can enrich their customer or sales data with geolocation information, such as adding latitude and longitude or addresses.
For example, a logistics company might use geolocation enrichment to optimize delivery routes by understanding the geographic distribution of customers. Similarly, a retail company could enrich transaction data with store location details to analyze geographic trends in sales.
3. Behavioral Enrichment
Behavioral data enrichment involves adding information about a customer’s interactions with a brand, such as browsing history, purchase behavior, or product preferences.
For instance, an e-commerce platform might enrich its customer data with browsing history to offer personalized product recommendations or tailored promotions, improving the overall shopping experience and boosting sales.
Comments ( 0 )