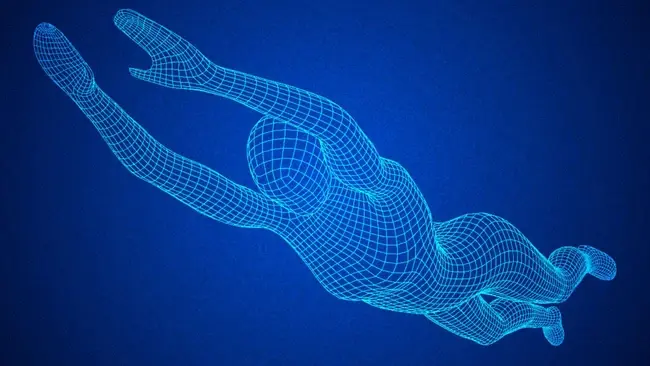
Today's large enterprises find themselves swimming in data from multiple sources but struggling to navigate towards meaningful insights.
While enterprise data is bubbling with valuable insights to help organisations move their business forward, many struggle to stay afloat in the sea of information in their possession.
The sheer volume and variety of data – from customer transactions to sensor readings and machine logs – can be overwhelming. Traditional methods of data analysis often fall short, leaving businesses adrift in a sea of information paralysis.
For organizations drowning in data, data discovery can help them ride the waves and achieve their data goals.
Too much data, not enough insights
Many businesses today operate in an increasingly complex multi-cloud environment where data is dispersed into several disparate, often siloed data sources across their organisation. In such conditions, data is difficult to find, understand, trust, and gain value from.
In fact, according to research by Forrester, between 60% to 73% of all data that enterprises possess goes completely unused.
The impact of not having a handle on what data you have, where it’s stored, who owns it, and the relationships between it, can be staggering. When organisations don’t have tabs on their data, they tend to lose sight of specific goals. They might collect everything without a clear purpose, leading to a "data lake" with untapped potential, or focus on irrelevant data due to poor data governance and lack of prioritization further exacerbating the problem.
Large datasets often also contain inaccuracies, inconsistencies, and missing values, leading to unreliable and misleading insights, and hindering effective decision-making. To unlock the hidden treasures in data, enterprises must become masters of data navigation to extract valuable insights and transform their data into actionable strategies.
For this, they’ll need to overcome data overload, prioritize quality, break down silos, invest in talent and technology, and foster a data-driven culture to truly harness the power of their information assets.
What is Data Discovery? Definition
Data discovery is the process of finding and analyzing data from various sources to identify patterns, trends, and insights. Its purpose is to reveal relevant data insights, communicate these insights to business users in a way that is accessible to non-technical users, and ultimately improve business processes.
The data discovery process is accomplished with visual data discovery tools and business intelligence tools that extract data from various sources and consolidate the data into a single location, where users can have a “big picture” view of their data.
The most crucial component of data discovery is the interactive visual analysis, which enables users to interactively and view their data from every angle, and drill down to hyper-specific events. Visual data discovery is the subsequent step after data exploration has first refined the data sets.
In the age of big data, organizations are generating more data than ever before. This data can be a valuable asset, but it can also be overwhelming. Data discovery helps you to make sense of your data and turn it into actionable insights.
As organisations explore your data, they may discover new questions that lead you to gather more data or refine your analysis. The key is to be curious, experiment, and keep iterating until you've uncovered the insights you're looking for.
The Role of AI in Data Discovery
The integration of AI into data discovery brings a new level of efficiency and accuracy to the enterprise. Machine learning algorithms can analyze data at a speed and scale that surpasses human capabilities, highlighting and identifying hidden patterns, correlations, and outliers within datasets, leading to more informed decision-making.
AI-powered data discovery tools can also ingest data from various sources, including databases, cloud storage, emails, social media, and even unstructured data in the form of documents like PDFs and images. This tool automatically analyzes the data to identify its type (e.g., financial data, customer data, sensor data), extract key entities and relationships, and understand its meaning and context.
Based on its understanding of the data, the AI tool can then generate insights, identify patterns and trends, and recommend actions to take. This could include suggesting relevant data sets for specific analysis, flagging potential risks or anomalies, or even generating automated reports and dashboards.
What are the benefits of data discovery?
Data discovery can be a game-changer for businesses struggling to extract value from their data, especially when they have large amounts of it. algorithms can delve deep into your data, identifying hidden patterns and relationships that might be invisible to human analysts. This leads to benefits such as :
1. Informed Decision-Making
AI-powered data discovery empowers organizations to make decisions based on data-driven insights rather than intuition alone. The ability to uncover hidden patterns and trends allows for more informed and strategic decision-making, leading to a competitive advantage in the market.
2. Operational Efficiency and Cost Savings
By automating data analysis and discovery processes, organizations can achieve significant operational efficiencies. Reduced manual efforts in data preparation and analysis translate to cost savings and allow employees to focus on higher-value tasks that require human creativity and intuition.
3. Innovation and New Revenue Streams
The insights generated through AI-powered data discovery can uncover new opportunities for innovation and revenue generation. Organizations can identify market trends, customer preferences, and emerging technologies, providing a roadmap for innovation and the development of new products or services.
4. Customer Experience Enhancement
Understanding customer behaviour and preferences is a key factor in delivering a superior customer experience. AI-powered data discovery enables organizations to analyze vast amounts of customer data, leading to personalized and targeted strategies that enhance customer satisfaction and loyalty.
As well as identifying patterns in your data, AI-powered data discovery can automate tedious tasks like data classification, cleansing, and preparation, freeing up your team to focus on higher-level analysis and interpretation.
It can also integrate data from various sources, departments, and systems, providing a holistic view of your business. This is because everyone can access and analyze the same data, fostering better communication and decision-making across departments.
By seeing how different parts of your business interact, you can find new ways to optimize operations and customer experiences.
Examples of Data Discovery
AI-powered data discovery has found applications across various industries, demonstrating its versatility and ability to extract meaningful insights from diverse datasets. Here are some notable examples of data discovery to show practical applications of the practice in action:
1. Advanced and AI Analytics
Businesses today are racing to leverage AI and (ML for advanced analytics and data science initiatives that can help them seize competitive advantage and deliver industry-changing innovation. But AI and ML projects require vast amounts of data.
Intelligent, automated data discovery enables data scientists and DataOps teams to rapidly find the data they need. In iterative ML projects that require additional data assets, AI-powered data discovery can help DataOps teams find trusted datasets to make available in data pipelines and streamline data preparation.
2. Customer experience
Delivering the experience customers expect requires your organization to have a 360-degree view of your customers and an end-to-end view of each customer’s journey and experience with the company each step of the way.
AI-powered data discovery enables you to build the data foundation for successful customer engagement by discovering and cataloguing customer data wherever it resides, identifying and classifying customer data domains, profiling data quality, and providing visibility into data lineage across customer touchpoints.
3. Data Governance and Privacy
Modern data governance leaders, along with data workers throughout the organization, need a solution that automatically discovers and identifies key data elements that need to be protected to safely enable use with revenue-generating applications. Intelligent, automated data discovery helps you discover and classify data—including sensitive data such as personal information—so it can be trusted, understood, and secured.
This helps ensure compliance with data privacy mandates while reducing the risk of abuse that could violate consumer rights policies or result in a data security breach. And by automatically relating data to business terms and definitions, an intelligent, automated data discovery solution can provide business context to data for enterprise data governance and fuel business value with trusted data.
4. Fraud Detection and Risk Management
In the financial industry, AI-powered data discovery plays a crucial role in fraud detection and risk management. Machine learning algorithms can analyze vast datasets of transactional data to identify patterns indicative of fraudulent activities. By detecting anomalies and suspicious patterns in real-time, financial institutions can mitigate risks, prevent fraud, and enhance overall security.
Final thoughts
As we generate more data than ever, our ability to unlock the true potential has become crucial. But there are key things you need to know before implementing data discovery in your business.
- Data discovery is a powerful tool, but it's not a magic wand. Uncovering valuable insights requires thoughtful exploration, collaboration, and critical thinking. Don't expect instant answers - the journey is as important as the destination.
- Remember, data is just one piece of the puzzle. Context is crucial. Combine your findings with domain knowledge, intuition, and ethical considerations to make informed decisions that benefit everyone.
- Embrace the iterative nature of data discovery. New questions will arise, leading to deeper exploration. Stay curious, stay flexible, and be prepared to refine your understanding as you learn more.
- Data democratisation is on the rise, empowering more people to leverage its potential. Advocate for data literacy and responsible analysis practices to ensure everyone can benefit from the insights we uncover.
- The future of data discovery is bright. Emerging technologies like AI and automation will augment our capabilities, but human judgment and creativity will remain essential. Use these tools wisely to unlock the true potential of data for good.