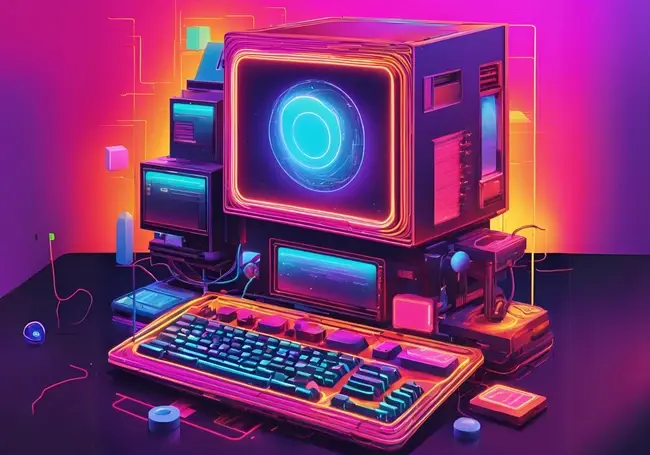
When it comes to AI in the enterprise, the ability to automate is crucial. At its core, Automated Machine Learning (AutoML) refers to using algorithms to automate the traditionally manual tasks in building machine learning (ML) models.
By handling repetitive and time-consuming steps, AutoML accelerates the model development process and makes ML more accessible to businesses and individuals, even those without extensive technical expertise.
This article will discuss what Automated Machine Learning is, its importance to the enterprise, and some downsides.
What is Automated Machine Learning (AutoML)?
AutoML makes it much easier to develop and deploy ML models. Automated complex processes such as data preprocessing, feature engineering, and hyperparameter tuning will enable enterprises and individuals to fully use the power of AI with extensive technical knowledge in a noncomplicated way.
With advanced AutoML tools, it's now possible to drastically reduce time and cost while increasing the accuracy of building predictive models. Whether a data scientist trying to streamline the workflow or a business executive seeking to integrate data-driven decision-making into operations, AutoML platforms are reshaping how machine learning is leveraged today in industries far and wide.
For example, Azure Machine Learning will train multiple parallel pipelines with the investigation of different ML algorithms and their parameters. Each iteration will output a model scored on your metric of choice for optimisation, ensuring the best fit of your data. It automatically stops when it meets the defined criteria for fit, thus streamlining model creation and evaluation.
How Does AutoML Work?
This process automates the iterative tasks involved in building and fine-tuning ML models. From model selection to parameter tuning, AutoML software handles these steps with high efficiency.
The result? Faster, more accurate outputs and the ability for teams to scale their ML applications effectively.
Why is AutoML Important?
AutoML is key in advancing ML and AI by addressing one of their long-standing challenges—the black box problem. This issue refers to the difficulty of understanding how ML algorithms produce their outputs.
While these algorithms enhance efficiency and boost processing power, their opaque nature often makes it hard to determine how they arrived at a specific result. As a result, selecting the correct ML model for a particular task becomes a challenge, especially when outcomes are unpredictable due to the black-box nature of some models.
With AutoML, these hurdles are significantly reduced. AutoML tools simplify the ML pipeline, making ML less of a black box. By automating crucial parts of the process, AutoML enables users to apply algorithms to real-world problems without requiring an in-depth understanding of the inner workings of these algorithms.
Tasks traditionally demanding significant human expertise, such as model selection, hyperparameter tuning, and feature engineering, can now be performed efficiently and at scale with AutoML solutions.
Thus, the automation of the ML process is revolutionising industries by:
- Improving efficiency: AutoML eliminates manual coding, reducing development time.
- Enhancing accuracy: Automated algorithms often outperform traditional, hand-coded approaches.
- Increasing accessibility: Businesses without in-house data scientists can benefit from robust AI-driven solutions.
What Are the Downsides of AutoML?
AutoML offers a streamlined approach to building machine learning models, but it comes with notable downsides that businesses should consider. One key limitation is its lack of customisation. While these tools are designed to simplify processes, they often prioritise automation over flexibility, leaving advanced users unable to tailor models to meet specific needs or address highly specialised applications.
Another significant drawback is its reliance on black box models, which lack transparency. These models make it challenging to understand how decisions are made, raising concerns in industries such as finance or healthcare, where explainability and compliance are critical.
This can create barriers to adoption, particularly for organisations that must demonstrate clear reasoning behind predictions or outcomes.
The computational demands of AutoML also present challenges. Running multiple algorithms to determine the best-performing model can be resource-intensive, leading to increased costs for organisations, especially those with smaller budgets or limited computing capacity.
Despite its promise of accessibility it still requires users to have some foundational knowledge of ML and data science. Without this, interpreting results or selecting appropriate data inputs can be a significant hurdle for non-technical users.
Finally, AutoML is not always the optimal solution for solving complex problems. While it performs well on standard tasks, it can struggle with unstructured data, such as images or natural language, where manual intervention or custom-built models may yield better results.
Comments ( 0 )