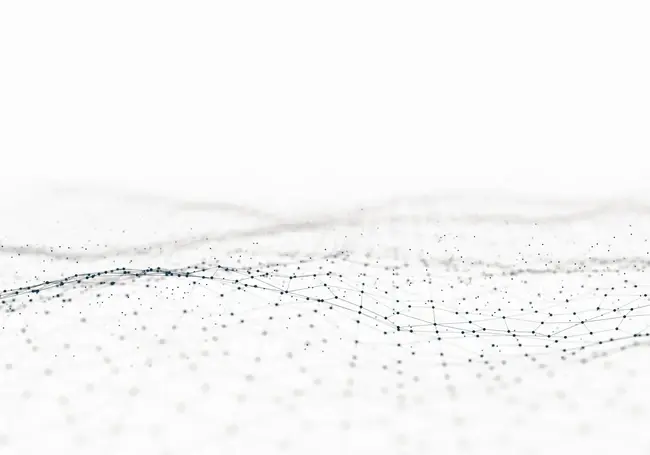
As organizations work on training AI models using vast volumes of datasets, the quality of the data being used to train the model will make or break the model. The more accurate the model’s output needs to be for the use case (e.g., data fueling self-driving cars vs. data for “getting to Springfield,” as my colleague Elise London explains), the more critical the quality and structure of the data that feeds the model.
The Importance of Clean Data
Data scientists stress the importance of high-quality data. But what does “high-quality data” really mean? Depth, breadth, history, frequency of collection, consistency, and structure all contribute to the quality of data. With today's massive data volume, removing poor or unnecessary data is crucial to AI success. The saying "garbage in, garbage out" has never been more relevant. After all, who would make critical decisions on bad info?
Clean data guarantees that your AI models are learning from accurate and reliable sources, preventing any costly future mistakes and enabling informed decision-making from day one.
What Is Clean Data?
“Clean” data is characterized not just by accuracy and consistency but also how data is collected and recorded. The more often data is collected the better, as long as it is well structured and easily to sort and understand. Training AI on poor data leads to unreliable and even dangerous outcomes. A recent report shows that trust in AI depends entirely on the quality of its data. Without trust, widespread adoption and successful outcomes become elusive, so training AI models depends on quality, clean data.
How to Get Clean Data
Given the growing investment in generative AI, the need for clean data is more pressing than ever. So, how can organizations assure themselves that they have the necessary data foundation for AI success? I’d offer 6 specific techniques to ensure you are cleaning and preparing your data to build trustworthy AI models and optimize AI performance:
1. Recognize importance: The first step towards using clean data is recognizing its importance. Once this is acknowledged, the next crucial step is to implement strategies that certify the data is clean. How you do that is covered in the next 5 steps.
2. Automate collection: Human error is a common pitfall in data collection. Automated systems can eliminate these errors and improve data accuracy. This not only improves the quality of your data but also helps prevent hallucinations in AI models. Automation also alleviates the subjectivity and bias that can arise from human interpretation, leading to more consistent and reliable data.
3. Focus on quantitative data: While employee sentiment is valuable, it can be influenced by emotions and biases. For more accurate data and AI models, prioritize objective metrics like user interactions and device performance to avoid any subjectivity of sentiment-based data.
4. Collect with purpose: Collect data that serves a specific purpose. Consider, what is the problem you are trying to solve and what data would I need to solve that problem? Every piece of data has a potential story to tell. If you understand that story and how to use it effectively, you can avoid collecting excessive data that won’t be used.
5. Validate and refine: Even after cleaning your data, you need to still validate the data and the AI model for accuracy. AI can make mistakes, so this step is critical. Does the data make sense? Regular checks and feedback stages can help guarantee the model is performing as expected and making reliable predictions.
6. Don’t forget the human element: Even with advanced technology, human oversight remains crucial. As the notorious Mars Climate Orbiter incident demonstrated, human errors can have serious consequences. Keeping humans in the loop helps reduce risks and ensure AI reliability.
Data excellence is an ongoing process, not a final goal. While absolute perfection is impossible to achieve, cleaner data is the first step towards that destination. By focusing on data quality and consistent collection methods, organizations boost the accuracy and trustworthiness of their AI systems.
Comments ( 0 )