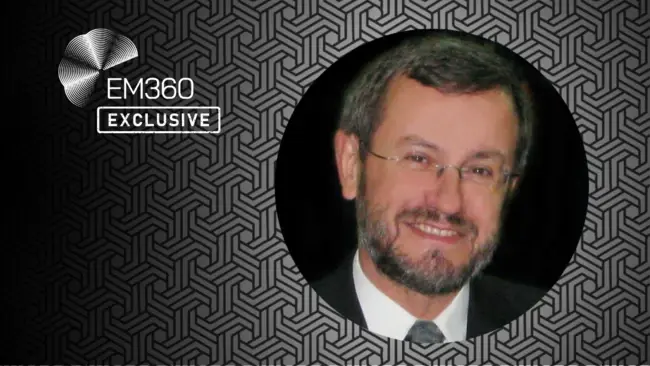
The AI revolution is upon us. It began with the launch of OpenAI’s ChatGPT last November, which dominated headlines for its impressive, human-like capabilities and incredible accuracy.
Now, AI is gripping Silicon Valley. Microsoft has so far invested over $11 billion into OpenAI as it hones in on integrating the technology into Microsoft products and services, while Google is moving huge amounts of resources towards AI departments, issuing “code-red” in a bid to defend its long-standing dominion on the search market.
As the AI race heats up, however, concerns are mounting about the rapid pace of AI development and the potential impact it could have on society.
Public figures including Elon Musk, Cognitive scientist Gary Marcus and Apple co-founder Steve Wozanik have signed an open letter calling for a temporary halt on AI research to prevent damage to society. Meanwhile, a privacy watchdog in Italy temporarily banned ChatGPT due to privacy concerns related to how the LLM-wired bot handles user data.
With the risk of rapid AI development becoming increasingly clear, what does the future hold for AI technologies? Will innovation prevail over fear, or does the impact of AI hinder its potential to transform society?
EM360's Ellis Stewart spoke to Prof. Ioannis Pitas, Director of the AI and Information Analysis (AIIA) lab at the Aristotle University of Thessaloniki and Management Board Chair of the AI Doctoral Academy, about the current state of AI technology and pressing questions about its impact on society.
Ellis: AI is obviously an incredibly hot topic right now. Where can you see the technology heading within the next ten years?
Prof. Pitas: “Large Language Models (LLMs) are the current trendsetter in AI research. They are only a precursor to future Large Perception Models (LPMs) that can incorporate the processing and analysis of multimodal information, notably aural and visual ones. Such advances are quite near, as the basic underlying LLM technology (attention and transformer networks) has already been used to solve computer vision tasks.
“However, much more needs to be done in LPM research as key scientific components are still missing. For example, we can neither quantify LPM ‘knowledge’, nor their knowledge capacity. In the meantime, an explosion in AI development is expected to come in the form of a confluence of LPMs with autonomous systems and robotics. The end result could be explainable, trustworthy and safe autonomous machines.
“An increase in physical intelligence complexity is needed so that artificial systems stand on par with complex living organisms like mammals; not to mention humans. Once this is done, it will pave the way for revolutionary AI science and engineering changes.
“The big question will then be whether such LLM inference mechanisms are just an illusion or a revolutionary approach to solving core AI research issues, such as reconciling Symbolic AI with Machine Learning while advancing the former, which has been stagnant for many decades.
“If LLMs indeed reconcile Machine Learning and Symbolic AI, this will be a revolutionary leap forward in AI Science and Engineering. LLMs can also provide incredible insight and new ways to study human intelligence. They are able to produce text to quality that varies from very good to passable. Such proficient text authoring by a machine can be analysed to create hypotheses on how human linguistic skills have evolved and how the related brain networks function.
“LLM-produced text and essays typically contain rather accurate factual information (though LLMs may at times hallucinate facts). A closed LLM system having billions of parameters should have a finite storage capacity, so It is quite puzzling how it can store an infinite amount of factual knowledge. This LLM storage capacity must be studied more carefully, as it could give us hints on how biological (including human) memory mechanisms function.
“Finally, the aforementioned LLM inference qualities can provide hints on how human inference and the mind operate. Such studies of human intelligence will give a much-needed impetus to Neuroscience, which lags in theory and is rather stagnant compared to the current AI revolution.
“In my view advanced AI systems will gradually approach a higher kind of 'machine intelligence' rather than human intelligence, which may ultimately be completely different from each other.
“They will obey the same physical laws but be completely different in terms of their material basis and architecture in the way aeroplanes are different from birds. This situation will last until we are able to understand better the nature of both humans and artificial intelligence and therefore develop complex biological artificial systems. Both of these developments are a long way away.
“Ever more complex and powerful AI systems could approximate human intelligence to a point that we cannot discern their difference anymore, particularly in remote or virtual environments. Then the legitimate question follows: will there be any difference between human and machine intelligence that is worth philosophically talking about? Maybe a new, by-design life form will enter into existence.”
Ellis: Stanford’s 2023 AI Index revealed that AI research is moving away from academia to an era of corporate control. As an AI academic, how do you think this could shape the future of AI?
Prof. Pitas: “It is indeed true that current AI Engineering research needs huge data, computing resources and funding that are no longer available even in the world’s top Universities.
“However, we already see the limits of such a research setting: extremely complex LLM systems are built, but their functionality is largely unknown. This raises not only scientific questions but also huge social risks – hence, the recent call for a 6-month ban on LLM development.
“However, such a ban is the wrong reply to the issues at hand. Instead, governments must push an acceleration of AI Science research, which is done at a slower and more controlled pace in Universities. It is this focus on AI science that sparked the development of generative AI in the first place.
“When Convolutional Neural Networks (CNNs) sparked the current AI revolution in the early 2010s, CNNs worked fine, but nobody knew why. It took AI scientists a couple of years to figure out the ‘whys’. This understanding sparked the development of generative AI (GANs).
“In my view, there are two ways forward: either the AI companies absorb academic research (and research Universities) or they open up their research to Universities. In either case, much stronger Industry-University cooperation is needed, whose forms go well beyond the current joint research and education projects.
Ellis: Italy temporarily banned ChatGPT due to data privacy concerns. Cybersecurity experts have also warned that AI may be used for Cybercrime. If halting AI development entirely isn't the answer, how can AI researchers limit the risks associated with AI?
Prof. Pitas: “There are at least two different issues related to this question. The first is the intellectual property of private data. LLM training is, at least partly, fed by using data that can be acquired, e.g., during LLM interview sessions or by web search.
“Needless to say, such session dialogues are the property of each interviewer, at least their part corresponding to her/his replies and comments. Unfortunately, LLM developers use the old trick to misappropriate interviewers’ data: they offer free access to LLMs in exchange for using such data in LLM training, without citing their goals and the related data use protocols.
“This has happened too many times in the past, e.g., to train web recommendation systems, web search engines, and for social media profiling. Such use of personal data is in violation of the user’s intellectual property rights and privacy. Furthermore, such bartering transactions raise serious multi-billion-dollar taxation issues.
“States all over the world have been too slow in regulating related transactions. However, compared to past inactivity, we do see an increased vigilance of some European national authorities, e.g., in Italy, on such issues grounded on General Data Protection Regulation (GDPR) provisions.
“The second issue is risk management. The proposed ban on LLM development will certainly not minimize AI-induced risks. Instead, a partial slowdown of LLM deployment could allow regulatory authorities to react to security and safety threats. However, in my view, even such a slowdown will not offer much.
“The best way to address these risks is the immediate and worldwide investments in regulation procedures and infrastructure. As Geopolitics are a crucial factor, we shall not be naïve in expecting an immediate solution to every threat.
“But it seems that all major global players now see the threat, each from its own point of view. Much can be done at the national/regional level and also at the international level. For instance, AI systems should be required by international law to be registered in an ‘AI system register’; notify their users that they converse with or use the results of an AI system and avoid claiming human qualities (e.g., love) that they do not possess anyway. Such simple regulatory solutions can help to build trust in AI systems, reducing technophobia.
“The true solution to countering risks is to overhaul the global education system at all levels, towards building/morphing knowledgeable citizens (I call it morphosis) that can reap the benefits of advances in development, while minimising the risks.”
Ellis: You mentioned in your opinion piece that AI must be developed openly and democratically. How can we democratise AI research?
Prof. Pitas: “My opinion piece gives a clue to research democratisation: advanced technologies that are now possessed by AI companies, should become open.
“Artificial intelligence-related data should (at least partially) become open, again towards maximizing benefit and socio-economic progress. However, proper strong financial compensation schemes must be introduced to compensate for any profit loss, due to the fore-said open-ness and to ensure strong future investments in AI R&D.
“As data is a primary form of social and personal wealth, the attitude towards private data openness should be changed, particularly in Western societies.
“We should move from punitive regulations to positive incentive-based ones that maximise citizen protection, data democratisation and valorisation”
Ellis: Everyone can’t stop talking about OpenAI’s ChatGPT, but are there any other notable AI tools that people aren’t talking about? Are there any hidden use cases of AI?
Prof. Pitas: “Artificial Intelligence systems and software are already ubiquitous. They are routinely used in many application domains, e.g., in web search, biometrics for passport control, medical imaging and diagnosis, recommendation systems and dating services in social media, to name a few.
“A notable recent advancement relates to Generative Adversarial Networks (GANs), which can be trained to generate beautiful images and videos from poetry.
“This technology could be used by properly educated artists to create new generative art forms that could be different and possibly superior to current art. GANs have been around for a few years already, but they only stirred public interest recently, due to their use in the creation of fake photos of famous people.
“They are as equally innovative as ChatGPT. However, it created much more noise in social media, as, for too many people, it challenged their belief that humans are superior to machines in a tangible way.”
More about this discussion can be found in Prof. Pitas’ book “AI and Society”. The latest edition of the four-volume book delves deep into the problem of AI risk, exploring how education is key to creating a knowledge society able to reap the benefits of AI research.
Read the four volumes below:
- Volume A: https://www.amazon.com/dp/9609156460?ref_=pe_3052080_397514860
- Volume B: https://www.amazon.com/dp/9609156479?ref_=pe_3052080_397514860
- Volume C:https://www.amazon.com/dp/9609156487?ref_=pe_3052080_397514860
- Volume D: https://www.amazon.com/dp/9609156495?ref_=pe_3052080_397514860
Prof. Ioannis Pitas (IEEE fellow, IEEE Distinguished Lecturer, EURASIP fellow) received the Diploma and PhD degree in Electrical Engineering, both from the Aristotle University of Thessaloniki (AUTH), Greece. Since 1994, he has been a Professor at the Department of Informatics of AUTH and Director of the Artificial Intelligence and Information Analysis (AIIA) lab. He served as a Visiting Professor at several Universities.
His current interests are in the areas of computer vision, machine learning, autonomous systems, intelligent digital media, image/video processing, human-centred computing, affective computing, 3D imaging and biomedical imaging.
He has published over 920 papers, contributed to 45 books in his areas of interest and edited or (co-)authored another 11 books. He has also been a member of the program committee of many scientific conferences and workshops. In the past, he served as Associate Editor or co-Editor of 13 international journals and General or Technical Chair of 5 international conferences. He delivered 98 keynote/invited speeches worldwide.