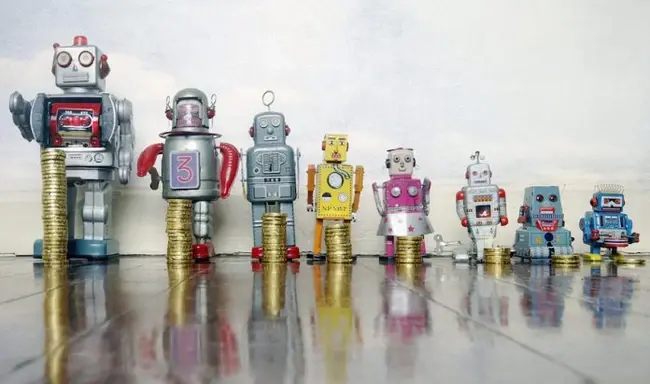
Today, many companies now process huge amounts of data using artificial intelligence (AI). If the data that fuels AI algorithms is unrepresentative of society, however, these programs essentially learn and adopt our biases.
AI's inherent bias
More organisations are now opting to employ algorithmic decision-making in order to reduce bias and improve operations. Nevertheless, it is possible for these algorithms to share many of the same vulnerabilities found in a human decision-making process.
Indeed, the interim report Bias in Algorithmic Decision Making released in July this year supports this. Published by the Centre for Data Ethics and Innovation (CDEI), the research illustrates evidence of historic bias in decision-making.
As the volume and variety of data increases, the algorithms used to interpret this information also become more complex. As a result, there are now growing concerns that algorithms risk "entrenching and potentially worsening bias" due to a lack of oversight.
Can data solve the issue?
While data is often the cause of AI's bias, it is also essential when it comes to tackling the issue. As the report outlines, it is common practice to avoid using data on protected characteristics in a decision-making process as it could be illegal.
For example, organisations collecting diversity data must ensure that this is separate from decisions about employment and promotion. On the other hand, some companies refuse to collect diversity information on the basis that it could perpetuate bias under certain circumstances.
In turn, however, this limits a company's ability to properly assess whether a system is creating biased outcomes. As the report notes, it is impossible to "establish the existence of a gender pay gap without knowing whether each employee is a man or woman."
For many companies, there is a need to create algorithms which are "blind" to protected characteristics. However, this creates tension with the need to check for bias against those same characteristics.
Removing our biases
It is evident that blinding algorithms to demographic differences and proxies may not always lead to fair outcomes. As the report exemplifies, an algorithm that calculates the risk of criminals reoffending without taking into account would likely result in disproportionately harsher sentences for women.
This is due to the fact that women tend to reoffend less often than their male counterparts. By excluding this key factor, therefore, the algorithm becomes less accurate for women and so, arguably, less fair.
As the aforementioned evidence illustrates, removing data bias is one of the most important ethical issues of our time. If we are to develop a fairer society in conjunction with technological innovation, organisations must ensure that their data is representative.
Looking to learn more about the ethical issues surrounding AI? Check out our podcast with Kasia Borowska, Managing Director at Brainpool AI