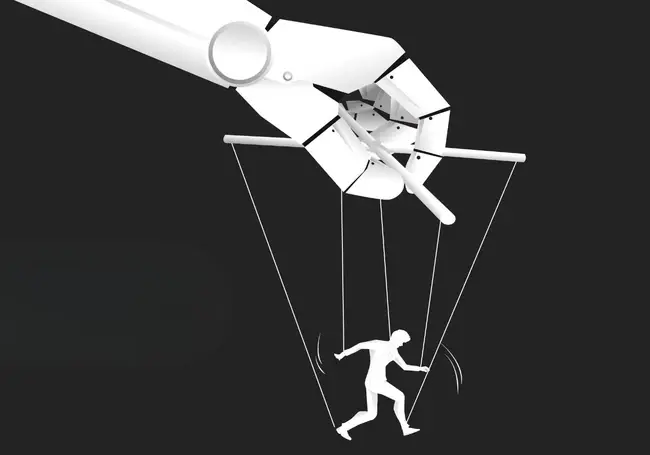
Your future could be decided by an algorithm you'll never understand. This isn't dystopian horror. It's already happening- it's called algorithmic bias.
While algorithms aren't inherently biased themselves, it's important to acknowledge the potential for bias and take steps to mitigate it where possible.
This includes using diverse and representative datasets, employing robust algorithms, and continuously monitoring and evaluating AI systems for potential biases.
It is also important to not overcorrect. Google faced criticism for “missing the mark” with its generative art feature in Gemini AI after users reported the tool generated images depicting a historically inaccurate variety of genders and ethnicities.
This article will explain algorithmic bias, how it happens and what happens when it gets taken to the extreme in the case of algorithmic radicalization.
What is Algorithmic Bias?
Algorithmic bias is the process in which a computer system produces results that are skewed or unfair.
This has become more apparent over the past few years where machine learning algorithms have become the norm. Individuals are now more aware of how these systems can prioritize certain information over others, raising concerns about fairness and transparency.
The rise of AI has led to algorithmic bias being a more built-in problem. Machine learning models not only decide what content we see to entertain but also the news sources and information we are able to access easily .
Companies like Buzzfeed aim to revert to an earlier form of the social internet, before the heavy reliance on algorithmic content feeds.
Algorithmic bias isn't just an online issue, it's a real world social problem that can affect peoples lives significantly.
Facial recognition systems rely on algorithms and often exhibit significant disparities in accuracy, performing far worse on individuals with darker skin tones, particularly Black women. This bias stems from a lack of diverse training data, primarily featuring lighter-skinned faces.
AI-powered hiring tools that are designed to automate resume screening and candidate evaluation, often unintentionally discriminate against women and racial minorities. These algorithms might pick up on subtle cues in resumes or application materials that reflect historical biases in the workplace.
Algorithms used for loan approvals can unfairly deny loans to individuals based on their race or zip code, even if those factors aren't explicitly considered. The algorithm might identify correlations between these factors and creditworthiness based on historical data.
This can restrict access to capital, affect economic mobility and perpetuate financial disparities. It can prevent people from buying homes, starting businesses, or pursuing educational opportunities.
Algorithmic bias reinforces existing inequalities and can lead to discrimination.
Biased algorithms can result in unequal access to care, misdiagnosis for certain groups, and the denial of necessary medical treatments.
Algorithms used for risk assessment or predictive policing can lead to harsher sentencing, increased surveillance in certain communities, and a perpetuation of racial profiling.
In education they can perpetuate stereotypes and limit opportunities for students from marginalized backgrounds.
How Does Algorithmic Bias Happen?
Algorithms and computer systems, like people, can never be perfect. However, algorithmic bias isn't simply a matter of occasional errors or misjudgements; it's a systematic skew in the results produced.
Algorithms learn from the data they are trained on. If this data reflects existing societal biases, the algorithm will inadvertently learn and perpetuate those biases.
There are a few ways that data can be skewed and impact bias. Historical data used to train algorithms can reflect past discriminatory practices, leading the algorithm to perpetuate those biases even if explicit discriminatory rules are removed.
Unrepresentative data, where certain groups are underrepresented in the training dataset, can result in algorithms that are less accurate or effective for those groups
Data with skewed labels, where the categories used to classify data are themselves biased, can cause the algorithm to learn and amplify those biases, leading to unfair or discriminatory outcomes.
What is Algorithmic Radicalization?
Algorithmic radicalization is an extreme result of unchecked algorithmic bias.
Social media, search engine and content recommendation algorithms can be designed to maximize user engagement above all else. This can inadvertently lead individuals towards increasingly extreme and radical viewpoints.
Algorithms track user interactions – likes, shares, comments, watch time – and then recommend similar content, creating personalized feeds designed to maximize engagement. Engagement is easier to win with content that is more shocking or novel, making users linger longer than they would on more nuanced complicated topics.
Simply put, sensational or controversial content, even if it's harmful or misleading, simply because it generates strong reactions meaning more engagement.
Personalized algorithms also lead to echo chambers that reinforce pre-existing beliefs, regardless of accuracy or extremity. Within these echo chambers, the algorithm may continue to push users towards even more radical content to maintain engagement, resulting in a gradual escalation of extremist viewpoints.
The consequences of algorithmic radicalization are significant. It can contribute to the rapid spread of misinformation and conspiracy theories. In some cases, this can lead to the adoption of extremist ideologies and violence.
Comments ( 0 )