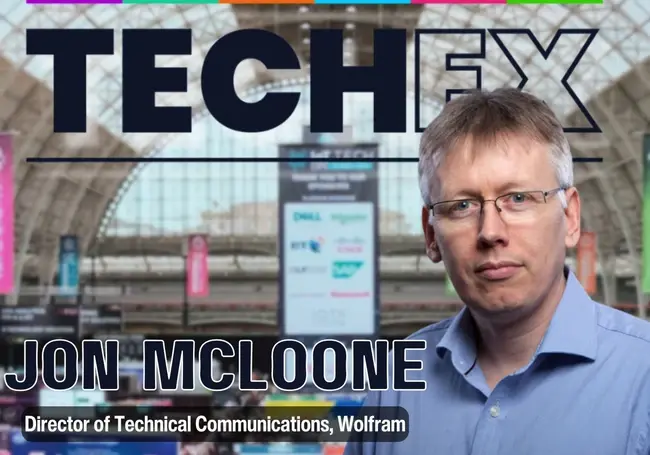
LONDON – TechEx Global, a leading enterprise technology exhibition and conference, wrapped up its two-day event on February 6th, showcasing the latest innovations across a range of sectors.
This year's event highlights the growing importance of AI, Big Data, Cyber Security, and other key technologies in driving digital transformation.
TechEx has been running for over eight years with the aim to bring industry leaders together responsible for pushing game-changing tech and strategy together, to craft relationships and creative solutions.
EM360Tech’s Shubhangi Dua spoke with Jon McLoone, the Director of Technical Communication and Strategy at Wolfram Research Europe at TechEx Global about the challenges and opportunities in the AI and data science industry.
Dua: What was the highlight of your day at TechEx?
McLoone: Wolfram has been in computation for 35 years. We do everything computational, whether it's engineering calculations, maths, data science, or statistics. Our technology stack and a services division are centred around that.
At TechEx, we're really talking about a subset of that, which is data science and the AI components. We’re looking for people with interesting projects that are data-driven or kind of trying to inject computational intelligence into what they're doing. We'll see if we can get projects built for them, or just let them use our tech stack and they can do it for themselves.
Dua: What's the biggest challenge you’ve observed in the tech industry, and how are you preparing for this?
McLoone: Right now, the big challenge is AI, because we're at the top of the hype cycle of calling it amazing, “we need to use this”. It’s amazing for certain things, but AI has massive limitations.
The basic challenge is that generative AI is fluent but not reliable. Generative AI is trying to make the point that because it's so prone to making stuff up or guessing, then it's great as an interface. It's great fun structured data, but it’s not the kind of golden bullet that people think it is.
So people are rushing into that space, spending a lot of money making proof of concepts that never get production because they just never do each of reliability, and that's where old fashioned computation, symbolic AI comes in.
What we're trying to do is engage with people, to help them understand, to help them realise that there is a route to reliability that you inject into the AI, but you never want to get the facts from the Gen AI. It's just no good for facts. It’s not good for anything that requires computation, like modelling, statistics or data science. It's great for some things, but people believe that they can do everything, and they're spending a lot of money just to fail.
Maybe this is off the record for me, but I feel that Jenny, I can be really stupid sometimes, because it cannot interpret numbers at all, no matter how much you write. Because I totally get your point.
Gen AI appears to be intelligent and actually can make intelligent decisions about certain kinds of things that it's very hard to write code for because we don't really understand how we make those decisions. It can't even count. If you just ask it to do some big multiplications, it can't get them right unless it's seen them before.
Dua: What technology do you see disrupting markets in the next couple of years and how do plan to tap into it?
McLoone: At the moment, we're still seeing a lot of hype around generative AI. However, there's also real potential for valuable applications. It's a rapidly evolving field think we're going to see more low-cost models. For a while, the focus was on bigger and bigger models, driven by the desire for smarter and smarter AI.
However, we've reached something of a limit—not quite the absolute limit, but we're running out of readily available data, and the cost of computing power is a constraint. So, I think that trend is starting to fade. But the availability of cheap, low-cost models allows you to do things like run multiple generative AI instances—say, 20—in competition with each other, each tackling different parts of a problem, orchestrated through that competitive process. There's a lot of potential there, and we're only just beginning to explore it.
Dua: What's the one key takeaway you'd like CIOs and CEOs to remember from our conversation today?
I'll give you a different point, which I think is something for the CIO group: There are certain technologies that are C-suite decisions. People worry about how data storage is done, and what systems they have. But manufacturing computation is not typically a C-suite decision.
I think that's completely wrong, because they leave it to random engineers, assuming the engineers know what tools they need to do their job. And so, all you end up with are collections of random different tools throughout the organisation—some engineering teams using Python libraries, others using R, and still others using, you know, some old-fashioned provider system. Some of them are using our technology.
What we're trying to do is create a kind of cover-everything platform, both for doing work and deploying work, so that computation can become the kind of infrastructure decision that databases are. If you're a CTO, you should be thinking, "What is my strategy for unified computation throughout my organisation?" so that engineers in one department can communicate with data scientists in another using the same language, and so that anyone can deploy anything immediately because there's a platform that will simply run it.
Of course, we're trying to provide that solution. But when we start these conversations, while people really like our technology, many aren't even asking themselves that fundamental question: "What is our computation strategy?"
Comments ( 1 )