Pimcore: 8 MDM & PIM Challenges You Can’t Ignore
Value Realisation in Data & Analytics Transformation
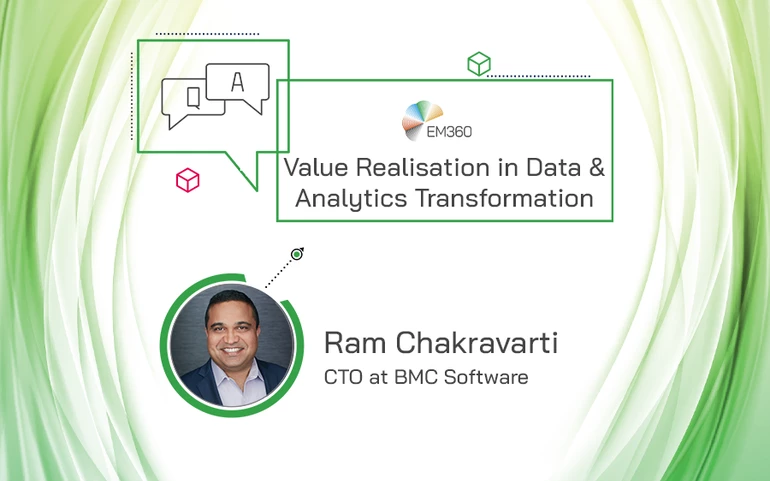
Unlocking the value of data for data owners has been headline news in business media outlets for over half a decade, which begs the question ‘Are organisations genuinely realising the true power and business benefits of data and analytics?’. Ram Chakravarti, CTO at BMC Software, proposed this very issue at this year’s AI & Big Data Expo. His keynote ‘Value Realisation in Data & Analytics Transformation’ explored his ultimate recipe for success for rapidly turning new insights into fully operationalised production deliverables – all designed to unlock tangible business value from data. To find out more, we spoke to Ram about the meaning behind his talk and why the enterprise needs to wake up to data’s full potential. In his role at BMC, Ram oversees the company’s overall technology strategy, corporate development, and shared services.
Drawing on your eponymous keynote at TechEx Global’s 2021 AI & Big Data Expo, what does value realisation in data and analytics transformation mean?
Value realisation of data is the ability to rapidly turn new insights into fully operationalised product deliverables that unlock business value from that data. I call this transformation DataOps and define it as applying the application of agile engineering and DevOps best practices to the field of data management. Success requires extensive collaboration across the data management ecosystem between data managers (such as data owners, data stewards, data architects and data engineers) and data consumers (who include data scientists, business owners, power users and end-users). No easy feat, but if organisations start with small scale rollouts and build on the lessons learned, they should have success in transforming their data and analytics practice.
Tell us about the traditional approaches to data and analytics transformations. Why do they need to be disregarded in the current digital era?
There are several reasons why a significant number of data and analytics transformations fail to deliver business expectations. These include new data or more complex business models making simplification challenging, as well as ‘process mismatch,’ whereby traditional data management processes do not work well with innovative technologies, such as artificial intelligence.
Some organisations find there is a lack of collaboration within the business or inadequate business involvement to drive a successful cultural shift, as well as a lack of budget for the amount of investment involved. Additionally, data analytics teams often require a combination of internal domain knowledge and deep technical knowledge, meaning there can be a scarce talent pool within the industry, and challenges in operationalising at scale with rapidly rising stakeholder expectations on speed, flexibility, timeliness, and customisation of new capabilities.
What kind of valuable information is hidden in enterprise data? Is there an untapped goldmine that many are overlooking or unaware of?
As an industry, we’re getting good at the intelligence and predictive analytics capabilities of AIOps to predict and solve traditional IT-related problems. But that same analytical capability can be applied to operational data from physical systems such as wind turbines, cell towers, and trucking fleets. All of this data can be analysed to help predict physical failures and improve maintenance schedules. Noisy datasets will be a barrier of the past as smarter strategies and centralised AIOps solutions help organisations improve the customer experience, deliver on modern application assurance and optimisation, coupled with intelligent automation to help organisations thrive as autonomous digital enterprises.
It doesn’t end there. By 2025, we can expect data to be reaching unprecedented levels, meaning essentially every company will become a technology-driven business. Industrial IoT can be used for predictive maintenance and improving the monitoring of physical systems. Customer engagement and social media data are all part of delivering a transcendent customer experience. The ability to quickly aggregate, scrub, and analyse your data is key. It paves the way for differentiated business data with key insights across your technology, tools, and employees which then make it possible to deliver a powerful, personalised customer experience.
How should data owners be using data to successfully unlock actionable insights and tangible business value? Please share your recipe for success.
DataOps’ combination of agile engineering and DevOps best practices in data management can rapidly turn new insights into fully operationalised production deliverables, that unlock business value from data. There are several key components, the first of which is extensive collaboration across the data management ecosystem between business and IT. DataOps enables pervasive automation, which encompasses orchestration, provisioning, configuration, and self-service.
Success requires extensive collaboration across the data management ecosystem between data professionals, such as data owners, stewards, architects, and engineers, as well as data consumers, such as data scientists, business owners, power users and end-users. DataOps platforms serve the needs of multiple data consumers, from traditional data users, such as data warehouses, to more sophisticated analytics platforms typically used by data scientists.
Some business decision-makers may view this value extraction process as daunting. What’s one piece of advice you would give them?
This can certainly be a large undertaking that requires collaboration among stakeholders and pervasive automation to be successful. While there’s no single magic recipe for success, I recommend that organisations approach this as a multi-horizon initiative, with a set of deliberate steps. Start small, with the introduction of DataOps in one or two analytics use cases; learn from these, refine, and then scale DataOps across business functions; and continue applying best practices.