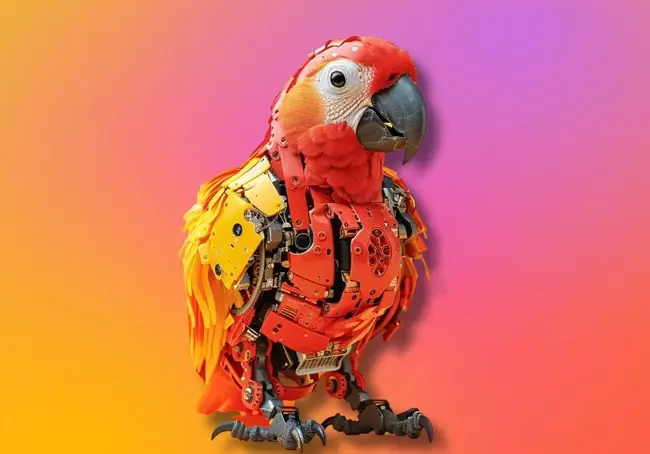
Large language models (LLMs) have taken the world by storm, with almost all companies adopting them into their workflow.
These AI systems can generate realistic and creative text, translate languages, write different kinds of creative content, and answer your questions in an informative way.
But are they truly understanding the language they process, or are they simply sophisticated mimics? This is where the concept of the "stochastic parrot" comes in.
In this article, we’ll explain what is a stochastic parrot and where the term comes from, and explore if large language models are stochastic parrots.
What is a Stochastic Parrot?
A stochastic parrot is the name of the theory that large language models (LLMs) do not understand the meaning of the language they process despite being able to mimic human language patterns.
The original paper where the term emerged, "On the Dangers of Stochastic Parrots: Can Language Models Be Too Big? 🦜" argues that because large language models do not truly understand the meaning of the language they process they can be dangerous.
This includes well-documented issues like AI bias and the potential for unintentional deception, as they can't understand the concepts underlying what they learn.
The rationale behind the theory is that large language models are trained on datasets and therefore limited to only being able to repeat content found within with these datasets.
Because the machine learning models are outputting information based on their training data there is no way for them to understand when they say something that is incorrect or incorrect.
LLM’s are able to identify the relationships between words and phrases, allowing them to generate seemingly coherent text like an advanced auto correct. However, crucially there is much about text that they cannot understand. Not only are they limited to their datasets but they are unable to understand key indicators of meaning like tone, sarcasm or figurative speech.
Are Large Language Models Stochastic Parrots?
Debate remains within the tech community over whether large language model chatbots built on machine learning are simply stochastic parrots.
Many users report that advanced models like ChatGPT appear capable of interacting with users in convincingly human-like conversations.
AI hallucinations are good evidence for the Stochastic parrot theory. In artificial intelligence, hallucinations are outputs that are factually incorrect or misleading, even though they might seem convincing at first.
If the data an AI is trained on is inaccurate, incomplete, or biased, the model will learn from those flaws and generate outputs that reflect them. Though AI models can be identify patterns in data, but they can struggle to grasp the real-world context. In some cases, people can intentionally manipulate AI models by feeding them specially crafted data. This can trick the AI into hallucinating specific outputs.
The SuperGLUE test is a benchmark dataset designed to assess a large language model's general-purpose understanding of the English language. It goes beyond simply measuring an LLM's ability to mimic human language patterns. The tasks are designed to be challenging for current natural Language Processing approaches, but achievable for college-educated English speakers.
The SuperGLUE test plays a significant role in evaluating the progress of LLMs. It helps researchers understand:
- How well LLMs can grasp the nuances of language beyond just statistical patterns?
- Areas where LLMs excel and areas where they fall short, like reasoning and real-world application.
- How effective new training techniques are in improving LLM comprehension.
As LLMs become more integrated into our lives, it's crucial to address their limitations. Researchers are actively exploring ways to bridge the gap between statistical fluency and genuine understanding and reading comprehension.
This might involve constantly updating real-world knowledge, improving reasoning capabilities, and developing new methods to detect and mitigate biases in training data.
The goal is to create large language models that can not only mimic and parrot back convincing enough language but also understand context and meaning. This would enable greater integration of AI that is beneficial to humans across the scope of our lives from revolutionizing medical care to enhancing our experiences at home and work.