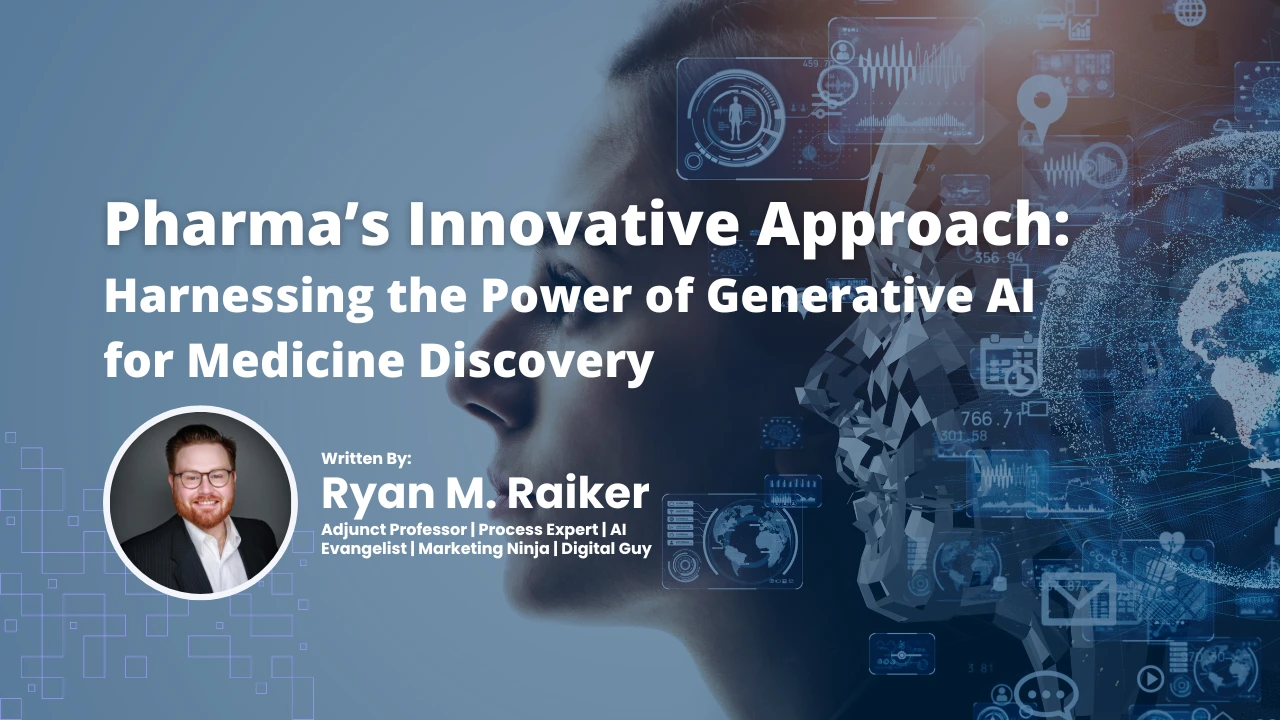
Global leaders in biotechnology like AstraZeneca, Pfizer, and Regeneron are making waves by integrating cutting-edge technology into their research and development processes. One such groundbreaking technology is Generative Artificial Intelligence (Gen AI), a revolutionary approach to drug discovery. This article explores how pharma and biotech companies leverage Gen AI to revolutionize how medicines are developed, ushering in a new era of efficiency and innovation.
Gen AI allows pharma companies AstraZeneca, Pfizer, Regeneron, and others to analyze individual patient data, enabling the development of personalized treatments tailored to a patient's genetic makeup. This approach, known as precision medicine, promises more effective and targeted treatments with fewer side effects. By understanding the genetic variations that contribute to diseases, pharma companies aim to create medications that are more potent and better tolerated by patients.
Understanding Gen AI
Generative Artificial Intelligence refers to a class of machine learning models that have the ability to generate new content, ideas, or solutions based on patterns and information they have learned. At its core, Gen AI goes beyond traditional AI applications by possessing the ability to create novel and meaningful outputs rather than relying solely on predefined responses. This paradigm shift represents a significant leap in the field of artificial intelligence, as it empowers machines to exhibit a level of creativity and problem-solving akin to human-like cognition.
In practical terms, Gen AI's applications are diverse and impactful, with one notable domain being drug discovery in the pharmaceutical industry. Companies are leveraging Gen AI to analyze vast datasets of biological and chemical information, accelerating the identification of potential drug targets and streamlining the early stages of drug development.
Accelerating Drug Discovery
Gen AI’s capacity to discern intricate patterns and relationships in data opens up new possibilities for innovative solutions and breakthroughs in various fields. One of the primary challenges in drug discovery is the time and resources required to identify potential drug candidates. Drug companies are using Gen AI to analyze vast amounts of biological and chemical data, identifying patterns and relationships that may not be apparent through traditional methods. This accelerates the identification of potential drug targets and streamlines the early stages of drug development.
Clinical trials are a crucial phase in the drug development process, but they are often lengthy and resource-intensive. Pfizer is leveraging Gen AI to optimize clinical trial design by identifying suitable patient populations, predicting potential adverse effects, and even suggesting optimal dosages. This data-driven approach helps streamline the clinical trial process, bringing potentially life-saving medications to market faster.
AI at Pfizer
Pfizer recognizes the power of collaboration in advancing healthcare. The company is scaling up and recruiting talent for a collaborative effort to get patients transformative medicines faster. Through partnerships with academic institutions, research organizations, and other pharmaceutical companies, Pfizer is creating a collaborative ecosystem for sharing data and insights. Gen AI facilitates the integration of diverse datasets, leading to a more comprehensive understanding of diseases and potential treatment options.
Working with AWS, Pfizer is already harnessing its power to drive innovation and productivity across 17 use cases. From scientific and medical content generation to manufacturing and more, prototypes using Amazon Bedrock and the AWS AI/ML ecosystem have been spun up in a matter of weeks. Pfizer estimates that some of the priority use cases will see cost savings from $750 million to $1 billion annually.
As an illustration, generative AI plays a crucial role in pinpointing novel targets for oncology. Currently, the process involves manually gathering information from various sources. However, AI can efficiently identify and compile pertinent data and scientific content from a multitude of sources in a fraction of the time. Subsequently, AI algorithms can analyze trends, generate potential targets, expedite validation processes, and ultimately enhance the likelihood of success.
Using AWS services, Pfizer quickly deployed VOX, its own Pfizer-certified generative AI platform that allows colleagues to innovate in a secure way with access to the best large language models, including Amazon Bedrock and Amazon SageMaker.
Pfizer employs automation, artificial intelligence, and predictive analytics to modernize and simplify medicine development. This approach allows for the precise collection and analysis of vast amounts of data, minimizing the risk of errors and accelerating drug development. Researchers are developing algorithms for wearable devices to help scientists monitor symptoms, assess health, and better understand how treatments work.
AI at AstraZeneca
AstraZeneca is leveraging artificial intelligence (AI) on Amazon Web Services (AWS) to revolutionize drug discovery and deliver impactful medicines. They have developed an AI-driven platform that enhances the quality and expedites the discovery of potential drug candidates. Utilizing various AWS services such as Amazon Elastic Kubernetes Service (Amazon EKS), Amazon Open Search Service, Amazon Kinesis, Amazon Aurora PostgreSQL, alongside open-source tools, AstraZeneca optimizes its platform to host predictive machine learning models, generative AI, a global analytical database, and advanced molecule search capabilities. AstraZeneca has moved 25 petabytes of data across the AWS global network, generating 75 petabytes of intermediary data in the process, resulting in the ability to run 110 billion statistical tests in under 30 hours and gain insights that influence patient health.
AstraZeneca’s use of AWS Graviton processors has resulted in up to 20 percent performance improvement for Amazon Aurora and up to 35 percent in cost savings.
Results:
Incorporates artificial intelligence and machine learning to accelerate drug discovery pipelines
Reduces time to market
Improved performance of Amazon Aurora by 20%
Saved up to 35% in costs
“The pace of change in healthcare is moving more rapidly than ever before as data and AI technology dramatically accelerate our progress. At AstraZeneca, we are embracing the adoption of responsible AI solutions, from discovery to clinical trials, treatment delivery and beyond to bring the right medicines to the right patients, faster than ever before.” - Sharon Barr, Executive Vice-President, BioPharmaceuticals R&D, AstraZeneca
AI at Regeneron
More than 95% of all experimental medicines that are currently in the drug development pipeline are expected to fail. To improve these efforts, the Regeneron Genetics Center built one of the most comprehensive genetics databases by pairing the sequenced exomes and electronic health records of more than 400,000 people. However, they faced numerous challenges analyzing this massive set of data:
Genomic and clinical data is highly decentralized, making it very difficult to analyze and train models against their entire 10TB dataset.
Difficult and costly to scale their legacy architecture to support analytics on over 80 billion data points.
Data teams were spending days just trying to ETL the data so that it can be used for analytics.
With Databricks, the team at Regeneron no longer needs to waste excessive resources on DevOps work setting up and maintaining infrastructure to support their analytics. Today, bioinformatics teams, data scientists and computational biologists can spend more time on higher-valued tasks such as developing novel new treatments.
Using Amazon Elastic Compute Cloud (Amazon EC2), a web service that provides secure, resizable compute capacity in the cloud, Regeneron and its collaborators have accelerated exome sequencing and processing of these genetic samples. The resulting petabyte of de-identified health and genomic data are stored securely using Amazon Simple Storage Service (Amazon S3), an object storage service. Storing this information on Amazon S3 offers Regeneron 90 percent cost savings compared to on-premises servers. By 2021, the RGC sequenced over one million genomes at 10 times the rate that would have been possible with local storage and computing.
Results:
Accelerated drug target identification: reduced the time it takes data scientists and computational biologists to run queries on their entire dataset from 30 minutes to down 3 seconds – 600x improvement!
Increased productivity: improved collaboration, automated DevOps and accelerated pipelines (ETL in 2 days vs 3 weeks) have enabled their teams to support a broader range of studies.
Sequenced over 1 million exomes at a 10x accelerated pace
Stored genomic data on AWS with 90% cost savings over on-premises storage
Enabled agnostic all-by-all data analyses to uncover genomic insights
Democratized access to improve diversity in genomic datasets that inform precision medicine
Discovered novel drugs based on genetic targets
Ethical AI in Biotechnology
Ethical AI, also known as Responsible AI, refers to the practice of developing and deploying artificial intelligence systems in a manner that aligns with ethical principles, fairness, transparency, accountability, and human values. As with any innovative technology, companies must remain committed to addressing ethical considerations associated with the use of Gen AI in medicine. Notably, each company researched for this article has emphasized transparency in its processes and decisions, ensuring that patients and the broader healthcare community are informed about the ethical implications of AI-driven drug discovery.
Addressing the ethical implications associated with their use becomes imperative as AI technologies continue to advance. Here are the key aspects of ethical AI:
Fairness and Bias: Ensuring fairness in AI systems involves preventing bias and discrimination. Developers must be vigilant about biases present in training data that could lead to unfair outcomes, especially in areas like hiring, finance, or law enforcement.
Transparency: Ethical AI requires transparency in the decision-making processes of AI systems. Users and stakeholders should clearly understand how AI systems operate, make decisions, and use data. Transparent AI helps build trust and accountability.
Privacy: Respecting user privacy is a fundamental ethical consideration in AI development. Companies must implement robust data protection measures, obtain informed consent for data usage, and prioritize the security of personal information.
Accountability: Developers and organizations must take responsibility for the impact of their AI systems. This involves addressing errors, providing avenues for redress when AI systems fail or produce unintended consequences, and establishing accountability frameworks.
Explainability: Ethical AI requires that AI systems are explainable and interpretable. Users and stakeholders should be able to understand the reasoning behind AI decisions, especially in critical domains such as healthcare or finance.
Social and Environmental Impact: Consideration of AI's broader societal and environmental impact is crucial. Ethical AI development involves minimizing negative consequences, such as job displacement, and ensuring that AI technologies contribute positively to society.
Inclusivity: Ethical AI promotes inclusivity and accessibility, ensuring that AI technologies benefit all members of society. Efforts should be made to prevent the exacerbation of existing social inequalities.
Collaboration and Standards: Collaboration among industry players, researchers, policymakers, and the public is vital to developing ethical AI standards and guidelines. Establishing ethical frameworks helps create a shared understanding of responsible AI practices.
As AI technologies become increasingly integrated into our daily lives, prioritizing ethical considerations is essential for building trust, safeguarding individual rights, and ensuring that AI serves the broader well-being of society. Ethical AI practices contribute to a future where artificial intelligence enhances human life without compromising fundamental values and principles. The biotechnology industry is at the forefront of AI breakthroughs and looks to be committed to upholding ethical standards in the usage and deployment of AI.
Ed Nowicki, Senior Vice President, Deputy Chief Compliance Officer at Pfizer, agrees. “New technologies call for new risk management practices," he says. “We partner across the enterprise to deliver innovation in alignment with our values and standards. It’s all about being proactive.”
2023 saw the adoption of Generative Artificial Intelligence grow tremendously, representing a significant leap forward in the quest for innovative and effective medicines. By harnessing the power of Gen AI, pharma companies are expediting the drug discovery process and paving the way for more personalized and precise treatments. As Generative AI continues to unfold its potential, the pharmaceutical sector stands at the forefront of a new era, where artificial intelligence becomes an invaluable ally in the quest for safer, more effective, and tailored treatments.