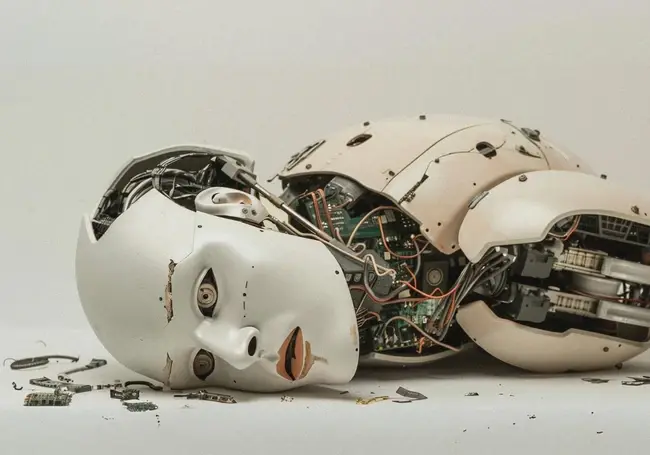
When OpenAI’s ChatGPT launched at the end of November 2022, it took the tech world by storm in a way few technologies had before.
Not only did it send Silicon Valley into a panic as tech companies big and small scrambled to embark on their own AI projects, but it also pushed generative AI into the mainstream so that everyone – in the tech space or not – had begun talking about AI.
But over two and a half years since the initial AI boom, there is growing evidence that the hype machine is beginning to slow down. Since peaking last month the share prices of the big tech firms driving the AI revolution have dropped by 15%, and a growing number of investors have begun to worry that AI may not deliver the vast profits they seek.
At the same time, experts are questioning the limitations of large language models (LLMs), which power AI technologies like ChatGPT. Big tech firms have spent tens of billions of dollars on developing these models since the AI boom began, but very few companies are actually using them.
In fact, the latest data from the US Census Bureau show that only 4.8% of American companies use AI models to produce goods and services, down from a high of 5.4% early this year.
While the longer-term predictions for AI remain optimistic, with all major tech titans continuing to splash the cash in the race, this drop in adoption marks a recalibration in the AI boom.
For the first time, it looks like the AI hype machine is losing steam.
Understanding the AI Hype Cycle
The AI hype cycle is a recurring pattern of intense enthusiasm and subsequent disillusionment surrounding artificial intelligence.
It follows a similar trajectory to other technological innovations as outlined by Gartner's Hype Cycle.The hype cycle typically follows these stages:
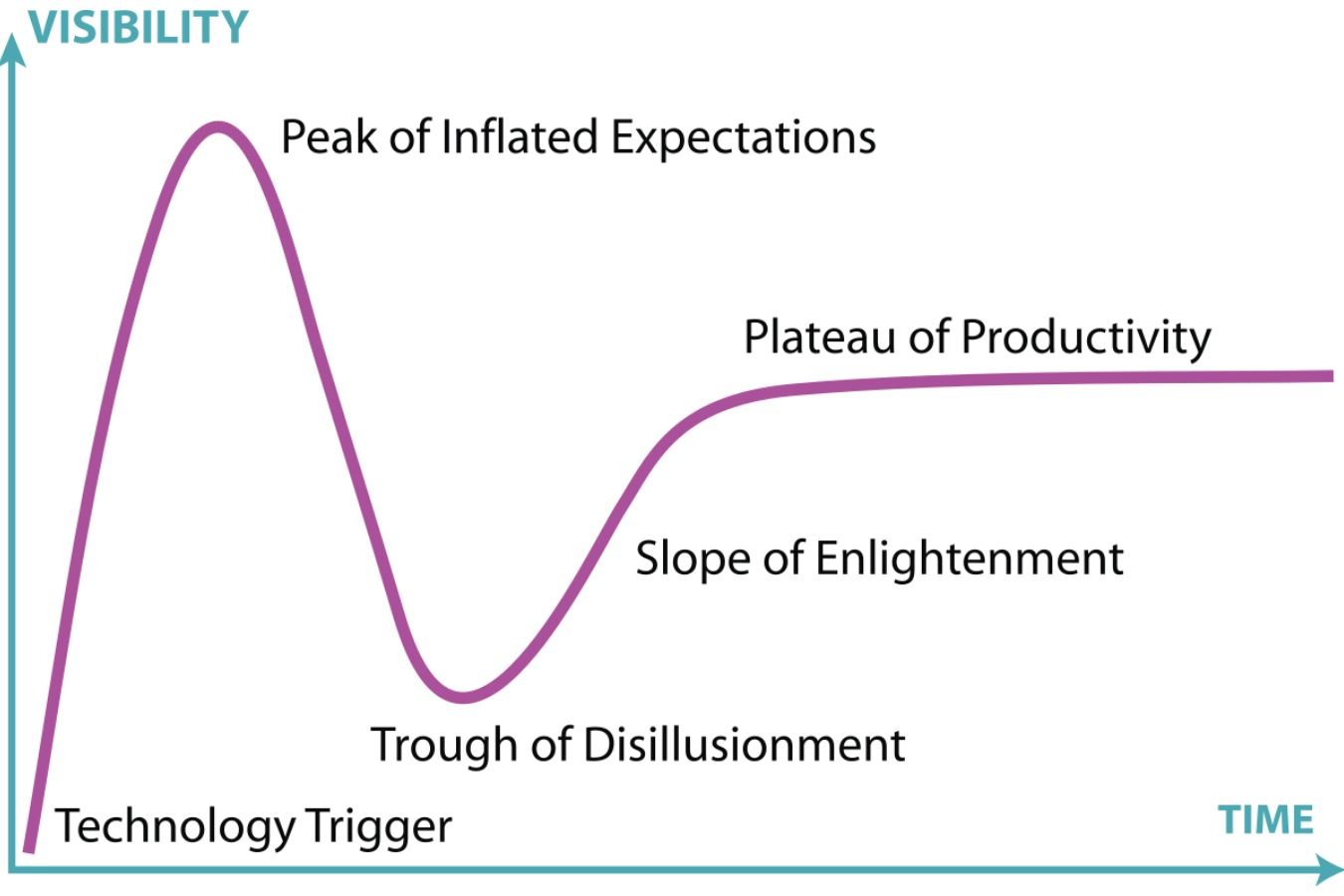
- Technology Trigger: A breakthrough in AI technology generates significant buzz and media attention.
- Peak of Inflated Expectations: Overhyped claims and unrealistic expectations about AI capabilities emerge.
- Trough of Disillusionment: As initial hype fades, challenges and limitations of AI become apparent, leading to decreased interest.
- Slope of Enlightenment: Practical applications and real-world use cases of AI start to emerge, leading to a more realistic understanding of its potential.
- Plateau of Productivity: AI has become widely adopted and integrated into various industries, delivering tangible benefits.
A Gartner report published in June listed most generative AI technologies as either at the peak of inflated expectations or still going upward. The report suggested most of these technologies are two to five years away from becoming fully productive.
But as the limitations of current AI technologies become more evident, it's likely we'll enter a period of disillusionment before AI matures and reaches this stage.
AI can’t keep up with the hype
Breakthroughs in generative AI, particularly in LLMs, have captured the world's imagination and led to extravagant claims about AI's capabilities and potential.
But the issue with many of today’s AI models is that they’re simply not powerful enough to be tangible. A study published last week by American think tank RAND showed that 80% of AI projects fail, more than double the rate for non-AI projects.
The RAND report lists many difficulties with generative AI, ranging from high investment requirements in data and AI infrastructure to a lack of needed human talent.
But above all, the unusual nature of GenAI’s limitations is one of its biggest barriers to success. Generative AI systems can solve some highly complex university admission tests but fail very simple tasks.
This makes it very hard to judge the potential of these technologies, which leads to false confidence in AI and ultimately failed projects.
Take for example McDonald’s attempt to automate drive-through ordering using AI, which went viral on TikTok after failing dramatically. There was also the US Government’s efforts to make systems to summarise public submissions and calculate welfare entitlements, which have also been abandoned.
If AI can solve complex differential equations or write an essay, it should be able to take simple drive-through orders or summarise information, right?
Another study by Cornell University showed that the abilities of large language models such as GPT-4 do not always match what people expect of them. In particular, more capable models severely underperformed in high-stakes cases where incorrect responses could be catastrophic.
The study also showed models can induce false confidence in their users. Because they fluently answer questions, humans can reach overoptimistic conclusions about AI’s capabilities and deploy the models in situations they are not suited for.
Experience from successful projects shows it is tough to make a generative model actually give accurate responses with human prompts. For example, Khan Academy’s Khanmigo tutoring system often revealed the correct answers to questions despite being instructed not to.
While AI has made astonishing strides, it's essential to remember that it's still a tool with capabilities and constraints in areas like common sense reasoning, understanding context, and generalizing knowledge across tasks.
Where is AI on the hype cycle?
As more organizations deploy AI solutions, the challenges of implementing AI in the real world are becoming increasingly clear.
As these challenges become more apparent, it's possible we're nearing the trough of disillusionment, where the gap between AI's actual capabilities and overblown promises becomes evident.
Of course, this is the natural and optimal progression for AI. Once the initial hyper fixation on AI subsides, the tech space can focus on practical applications, address limitations, and ultimately reach the plateau of productivity, where AI delivers tangible benefits across various industries.
Will AI lose its hype?
The current frenzy surrounding AI is undoubtedly fueled by recent breakthroughs, particularly in large language models. However, as with any new technology, the initial euphoria often gives way to a more sober assessment of capabilities and limitations.
However, it's important to note that a decline in hype doesn't necessarily equate to a decline in AI's importance. Just as the internet and smartphones endured their own hype cycles, AI is likely to become an integral part of our lives, even if the public fascination wanes.
AI is rapidly improving, too. Studies show that the size of language models (number of parameters), as well as the amount of data and computing power used for training, all contribute to improved overall model performance.
Large language models also display so-called emergent abilities, which are unexpected abilities in tasks for which they haven’t been trained. Researchers have reported new capabilities “emerging” when models reach a specific critical “breakthrough” size or amount of parameters.
This means that the more parameters that big tech developers add – which continues to grow day by day – the better AI will become. So with AI firms constantly at work building bigger and more expensive models, and tech companies such as Microsoft and Apple are still betting on returns from their existing investments in generative AI.
According to one recent estimate, generative AI will need to produce $600 billion in annual revenue to justify current investments – and this figure is likely to grow to $1 trillion in the coming years.
Ultimately, the true measure of AI's success will be its practical applications and the tangible benefits it delivers to society. For now, we’re yet to see those applications in the real world.