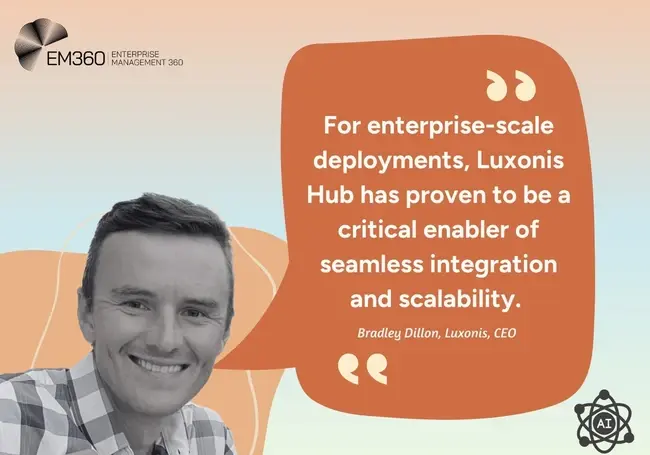
With rapidly advancing artificial intelligence (AI) driven technologies such as Vision AI and Voice AI, enterprises are seeking solutions with easier and more efficient edge AI integration and scalable systems.
According to Fortune Business Insights, the global edge AI market size was valued at USD 20.45 billion in 2023. The market is projected to grow from USD 27.01 billion in 2024 to USD 269.82 billion by 2032, exhibiting a CAGR of 33.3% during the forecast period.
To make such technologies easily accessible to organisations, tech companies are investing in advanced machine learning models.
One such company, Luxonis provides seamless integration of traditionally separate components, addressing the challenges of thermal management, power efficiency, and on-device processing.
EM360Tech interviewed Bradley Dillon, Chief Executive Officer (CEO) of Luxonis about machine learning (ML) processing, its limitations, advantages and the most effective ways for enterprise-scale deployments.
What specific hardware limitations must be overcome when integrating machine learning (ML) processors into high-resolution camera systems?
At Luxonis, we integrate multiple systems traditionally treated as separate: computational power for business logic, AI power for high-level world understanding, and camera/computer vision capabilities for extracting images and feature data.
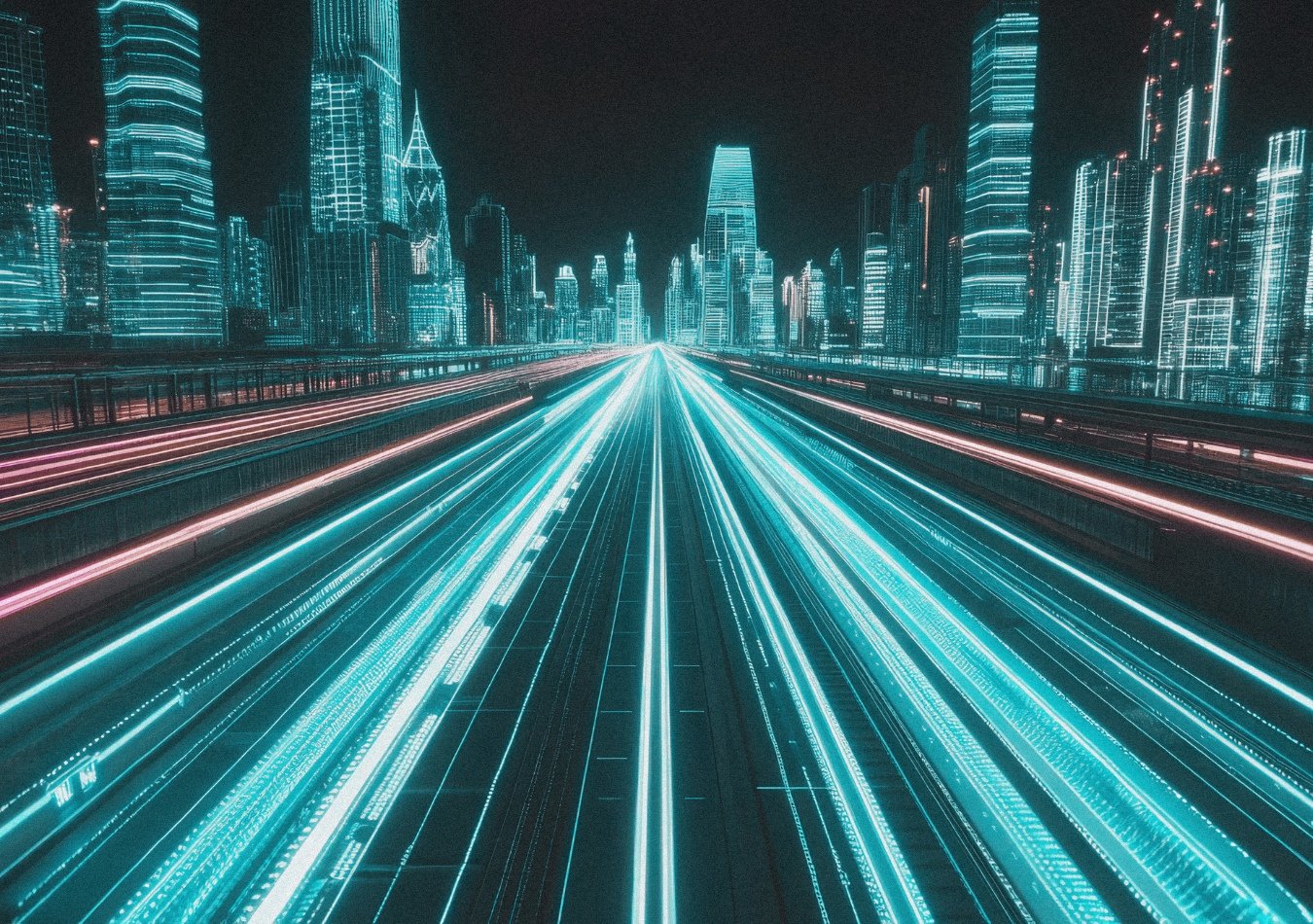
The main challenges involve balancing these elements to function seamlessly in edge devices. Specifically, combining high computing power and AI capabilities with vision processing in a compact form and ensuring efficiency in both hardware and software to address real-world challenges effectively.
How do thermal constraints affect the performance of on-chip ML processing in-depth vision cameras?
Thermal constraints are critical as onboard computing and AI power requirements grow to solve increasingly complex real-world problems.
At Luxonis, we focus on high-performance, low-power chipsets which ensure sufficient computational power within the thermal envelope of compact edge devices. Additionally, effective thermal management is essential for maintaining reliability and performance in small form-factor devices.
Also Read: Offline AI Helps Businesses Tackle Unpredictable Environments, Says Vivoka CPO
Which real-time processing bottlenecks persist despite current technological advances in on-chip ML?
The most significant bottlenecks come in the form of model performance or accuracy, rather than latency. This could be explained by accuracy vs. model size. Larger models provide better accuracy but are challenging to deploy on edge devices. Smaller models, which are edge-compatible, can suffer accuracy degradation.
While latency is less of an issue, technological advances are gradually enabling larger, more accurate models to run directly on edge devices.
What security vulnerabilities emerge when implementing on-device ML processing for depth vision systems?
Security concerns primarily involve data protection and intellectual property (IP).
Safeguarding data processed on-device from interception or misuse. Whereas IP ensures AI models and business logic deployed on edge devices are secure from potential tampering.
This is because edge devices are often in physical proximity to adversaries, security measures must be robust and tailored to the application.
How do compression algorithms impact depth accuracy in ML-enabled camera systems?
Luxonis employs CV-based depth estimation methods that are not affected by model sizes or compression algorithms. However, ML-based depth estimation networks, which are deployable on Luxonis devices, rely heavily on large network sizes. Compressing these models can significantly reduce depth accuracy.
Advances in architecture are leading to smaller, more efficient models, improving performance on edge devices without significant compromises.
What metrics effectively measure the success of ML-integrated depth vision implementations?
Metrics are task-specific. For straightforward tasks like Optical Character Recognition (OCR), success is measured against ground-truth data.
For more complex applications like 3D perception and tracking, metrics such as Root Mean Square (RMS) error are used to evaluate model performance across datasets and sequences.
Which integration protocols have proven most effective for enterprise-scale deployments?
For enterprise-scale deployments, Luxonis Hub has proven to be a critical enabler of seamless integration and scalability. Luxonis Hub provides the tools and protocols necessary for managing and deploying vision systems efficiently across large and complex enterprise environments.
Luxonis Hub simplifies the integration and management of devices across multiple locations. It provides version control, real-time monitoring, and over-the-air (OTA) updates.
Version control Ensures all devices run consistent firmware, software, and ML models, reducing errors and simplifying updates. Real-time monitoring allows enterprises to track the health and performance of their devices, enabling quick troubleshooting and proactive maintenance. Over-the-air (OTA) updates seamlessly deploy updates to firmware, software, or AI models across hundreds or thousands of devices without manual intervention.
How do current ML models balance processing efficiency with depth vision accuracy?
The design of ML models for edge devices involves architectural efficiency. Unlike cloud models that improve accuracy by increasing size, edge models must operate within fixed computational resources (for example, frame rate and processing power).
Also, prioritising smaller, task-specific models tailored for high-speed, real-time processing while maintaining as much accuracy as possible.
Comments ( 0 )