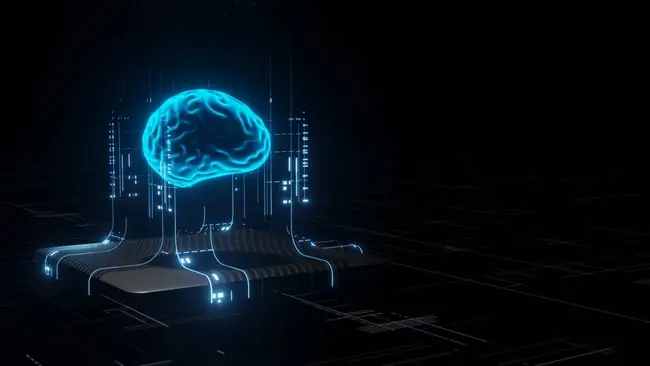
The COVID-19 pandemic has imposed lots of ‘firsts’ on businesses. For most companies, this year marks the first time that they have ever operated exclusively remotely. Similarly, some organisations had to pivot their offering and follow a new strategy for the first time, such as breweries making hand sanitiser or clothing designers manufacturing face coverings. Elsewhere, other businesses had to find new ways to reach customers, such as restaurants offering delivery services and launching their own apps – again, for the first time.
Despite the typical 2020 buzzwords (‘uncertain’, ‘unprecedented’), businesses could more confidently carry out these decisions because actually, they’re in a better position to take risks.
This is largely down to shifts in attitudes. In acknowledgement of the challenging times, customers are more patient and understanding, whether that’s in regard to later delivery times or slower resolution of their queries.
Businesses have also changed their attitude towards failure, simply because they’ve had to. Trial and error has been a large part of navigating through the pandemic, and as a result of that, companies have had to become more open to risks. What’s more, perceptions of failures are less about nails in the coffin; rather, failure is now regarded as a way to learn and move on.
This is especially true where machine learning (ML) and artificial intelligence (AI) are concerned. The very foundations of ML are that it improves from experience, which makes it one of the unique disciplines that is not focused on getting it 100% the first time.
Looking at failure in a different light
Failure is nothing new in the ML and AI spheres, with over 95% of related projects never coming to fruition. However, that statistic could be challenged if we alter perspectives of what constitutes success and failure in ML.
In our latest podcast with Cloudera, Cloud Machine Learning Specialist Jeff Fletcher reminds us that your ML model doesn’t always have to be perfect. Building the best possible data model is a huge challenge in ML, largely due to the difficulty of acquiring the necessary high-quality training data.
Furthermore, training data is just the tip of the iceberg. Yes, it needs to be in a specific format to work, however, as Jeff points out, “the difficulty is, once you’ve got it in the right format, machine learning algorithms do their own thing.”
In particular, he describes how despite being well-handled by academic and engineering teams, it’s difficult to “get it through that flow.” The flow is built of numerous steps: getting the data in a reasonable amount of time, getting enough data to meet predictions that the model in question would be valid, and having it flow all the way through, free of errors.
The difficulty for most customers, Jeff explains, is getting it all the way through. Problems often occur due to an oversight by a team somewhere in the flow, such as between the data ingest team and the data cleaning team.
Jeff’s advice is to focus not on having the best model in place, but having a model in place. “Get something working. Get something that’s delivering a result because then you’ve understood the pipeline.”
Jeff reassures that even if your model is not amazingly accurate, but say, 80% accurate, it’s still useful. In the context of fraud detection, if fraudsters have an 80% chance of getting caught, that’s enough to keep them at bay.
The other issue Jeff discusses is how you can affect the data environment you operate in. Putting the case in point, Jeff shares the example also with fraud detection. “As you get better at detecting fraud, fewer people will commit fraud that same way and the actual valid data – the real fraudulent transactions – used to make predictions reduces to the point that your machine learning models are no longer accurate. Then, you get a much higher false positive rate.
“So, you’re then predicting that something is fraudulent, when actually, it wasn’t. It’s just that your model has done such a good job that its no longer useful”
Sometimes, it’s best to keep it simple
Similarly, Jeff stresses that data practitioners shouldn’t fixate on trying to be overly complicated. Rather than trying to do something state-of-the-art, it’s more useful within a business setting to solve the more simple – and frankly, boring – problems.
“Something I often see is data science teams overreaching and trying to solve a problem like building a chatbot rather than a basic classification problem.” Jeff recalls. “It’s just fundamental. Chatbots are really hard to get right, and they have limited scope in terms of their utility, but it’s a thing that people want to build.”
Instead, he explains that there is a sense of utility that comes from solving something as simple as better churn prediction or revenue forecasting rather than trying to come up with a fancy deep-learning model for a chatbot that customers are never going to interact with.
Of course, in tech, there will always be that burning desire to work on career-defining, groundbreaking projects. As Jeff told us, a lot of data scientists and practitioners want to be seen as “the person who’s creating a new neural network to solve a new, problem.” However, he points out that frankly, that’s not the reality for most practitioners.
“The problem space we’re trying to solve is much simpler, but hard to implement because of the complexity of machine learning as a workflow.”
The fact of the matter is that while data practitioners are unlikely to get much public recognition for solving a simple problem, it’s the resolution of those simple problems that are most useful for a company.
“What you actually need to do is something simpler, but just do it better, because that’s what’s in the business’s interests.”
The takeaways are clear in that it’s time to challenge our perceptions. As attractive as flashy machine projects might be, the real value is in solving simpler problems – and if you don’t get it perfect? Well, that’s not always a bad thing.