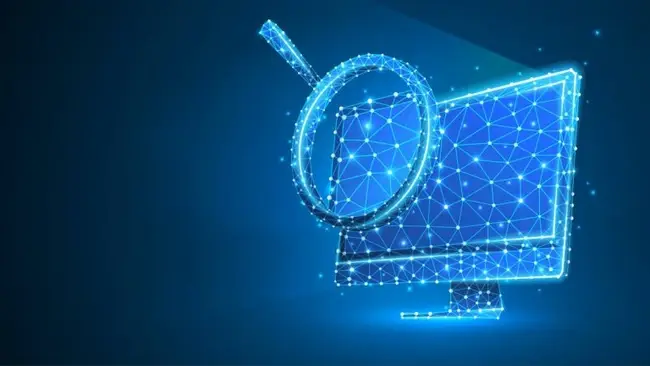
Everything in the enterprise landscape right now is go, go, go. Businesses are upping their game in any way they can to be more competitive, more secure, more agile, more automated – the list goes on.
It's an exciting time, for sure. Organisations are becoming increasingly digital, and business as we once knew it is no longer the same. Today, the lifeblood of companies is their data – data, data, data. In particular, businesses zero in on their data to know their customers better, improve decision-making, fashion more accurate predictions, and much more.
Organisations can also use data to facilitate technologies such as artificial intelligence (AI). The two have a reciprocal relationship; AI helps organisations do more with their data, but it also relies on accurate data to prosper. The more data businesses can get their teeth into, the better. Simultaneously, the more clean data that companies can channel into their AI and machine learning (ML) models, the better the models are.
It's not all rainbows and butterflies
Despite the highly anticipated synergy between data and AI, a new report by Trifacta, titled Obstacles to AI and Analytics in the Cloud, found that over one-third of AI and analytics projects in the cloud fail due to poor data quality.
The global leader in data wrangling surveyed 646 data professionals across different industries. In particular, the research explored how organisations are handling the transition of data to the cloud, the obstacles of data cleaning for analytics, and the time constraints data professionals face when preparing data for analytics, AI, and ML initiatives.
The move to the cloud is hugely important for businesses to withstand the enterprise landscape today. Data storage and management is far better in the cloud, particularly in light of the sheer volume we produce today. Keeping in line with this, Trifacta's research uncovered that 68% of IT professionals reported that all or most of their data is currently stored in the cloud, rather than on-premise.
However, as relayed in Trifacta's research, the move to the cloud can have a negative impact on an organisation's AI and ML initiatives if the data companies are using for these initiatives is inaccurate. One of the research's biggest take-aways is that poor data quality is a significant hindrance to AI and analytics in the cloud. It makes sense: if you're going to cook with rotten ingredients, you'll only dish up a rotten meal. According to Trifacta's research, poor data quality led to delays in AI/ML projects. Furthermore, it proved costlier and did not meet expectations.
Adam Wilson, CEO of Trifacta, shared the impact of cloud computing on AI initiatives:
“The growth of the cloud is essential to the future of AI and ML initiatives, but this rapid pace can cause challenges for many organizations. It’s important for organizations to remember to remain disciplined with their data preparation processes as they move workloads to the cloud.”.
As cloud migration continues to rise, the problem with data quality is not going away. Trifacta’s report finding that data quality is a top obstacle in organisations’ efforts to modernise analytics processes in the cloud demonstrates the focus every organisation needs to place on data preparation.
Dealing with data inaccuracy
Data inaccuracy falls under the poor quality umbrella. Unfortunately, inaccurate data is commonplace everywhere (like when you Google the opening times for a store, only to arrive and find it's not open), and the enterprise is no exception. As detailed by Trifacta, common mistakes include miscalculating demand and targeting the wrong prospects, which can have negative impacts on a company’s bottom line.
However, to avoid data inaccuracy, data professionals are instead having to spend a lot of time combing through it. In fact, some are spending upwards of 40 hours working on it.
The research shines a spotlight on the fact that AI/ML projects need clean data. Businesses should explore innovative data technologies to improve data quality and accuracy, rather than continue soaking up data professionals' time sorting through it. Organisations can leverage these technologies to also deliver better speed, and at scale.
Today, there is a fantastic portfolio of tools available to help businesses get the most out of their data. With these, organisations can go on to truly make the most of AI-driven opportunities. For companies that don't want to fall behind, this is non-negotiable.
Are you inspired? Why not start by checking out our Top 10 Data Cleansing Solutions?
Comments ( 0 )