AWS vs Azure: What’s the Difference in 2024?
The Chip Shortage Is Leaving a Mark on Big Data Deployments
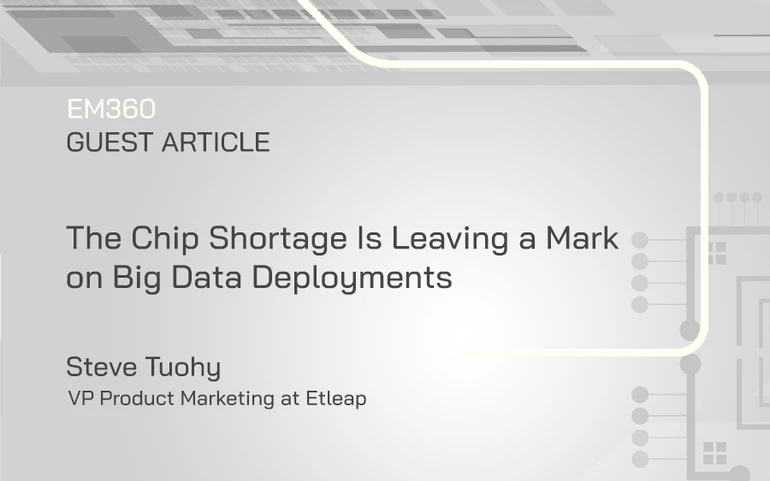
The long reach of the chip shortage
By now, most of us have seen headlines of the global semiconductor chip shortage. The underlying supply chain challenges are not an arcane matter of the global economy, but one with widespread impact that may extend for years.
Tech-heavy industries like data management depend heavily on microchips. But these days, the impact of these shortages has made its way into the formerly low-tech world. One example of this is the dog-washing business, whose precision equipment now is microchip-powered. The global shortage has left manufacturers juggling for supply and dog owners facing price increases.
The crunch has challenged many industries between the low-tech pet care and high-tech enterprise data worlds. Apple stocked older iPhones when it could not source enough components. GM and Ford cut vehicle production and idled factories, reducing pay for thousands of workers. Sony can’t keep up with demand for its PlayStation5 gaming console.
Impact on big data use cases
The modern data management technology stack has grown to be able to process unprecedented amounts of data. Given that this processing depends on various microprocessors, one is right to wonder how the chip shortage will impact organizations’ ability to keep up.
Big data processing platforms promise near infinite ability to scale out; simply add additional servers to increase compute capability. There have always been limits to this promise, and in a world of scarce processors, the limits matter even more today.
As with many parts of the global economy, the impact of the chip shortage is complex. Some sub sectors have greater shortages than others as manufacturers have prioritized their higher profit areas. For example, server CPUs have thus far been more available than network switch chips. That may seem like good news for big data scaling, but as organizations grow compute clusters, each CPU server requires a wide variety of components. The significant shortage of power management chips, circuit boards, and other components can be an equal challenge to scaling out.
In August, TSMC, the world’s largest contract chip maker, announced it would raise prices 10-20%. When a single server can have a lifetime cost of $40K or more and may not be immediately available, management is apt to scrutinize Hadoop and Spark scaleouts of 10s or 100s of servers or more.
Data demands can’t wait for supply chain stability
What is a data-driven organization to do amidst these shortages and high prices? Forrester, the industry analyst and research firm, counsels tech buyers “to be flexible, patient, and improvisational” and lays out several options. The first two options are to “wait” and “pay more.” In other words, basically deal with the sometimes painful laws of supply and demand. “Cancel your order” is another option for those who view their data capability as … optional.
The other options are to find more efficient approaches. Forrester suggests finding other suppliers, buying used, and tapping into underutilized assets. “Choose another configuration” is a specific suggestion.
Accelerate what you have
One particularly efficient approach is big data acceleration - software that speeds up specific computing tasks with the existing hardware or taps into more efficient computing. Bigstream software is one option that enables software-only acceleration or empowers the seamless, no-code addition of advanced compute, including field-programmable gate arrays (FPGAs). This can double an organization’s computing performance without expanding server infrastructure on Apache SparkTM and Hadoop clusters. FPGA hardware is often less than a tenth the price of a server. Graphics processing units (GPUs) are another form of acceleration often used for machine learning workloads.
The chip shortage may be the ideal time for organizations to examine their environments and move to more efficient configurations. While infinite horizontal scaling (adding more and more nodes) is attractive, network overhead and other communication become bigger challenges in larger clusters. Performance scales much worse than at a linear rate.
“Run to the cloud” comes to many CIOs’ minds for all headache-inducing infrastructure challenges and is another Forrester suggestion. Most organizations have evaluated that process prior to the chip shortage. Security and other concerns prevent many large organizations from moving data to the public cloud, yet a growing share of big data workloads is still shifting to the cloud. Organizations moving workloads to the cloud should also be mindful of scaling in the most efficient ways. Managing costs with optimal cloud instances and spot pricing can help, and big data acceleration is also an easy step to add.
The public cloud providers are also not immune to the global supply chain challenges. As data workloads do move to the cloud, the increased demand and the chip shortage will create upward pressure on cloud computing prices. It could also reduce availability of spot instances. All of these increase the incentive for organizations to build to get the most out of their selected compute instances.
Scaleout needs a better approach
CPUs are ideal for general-purpose computing. They can process almost all workloads even if it’s not in the fastest way. There are many situations where general-purpose is not ideal, and for big data, acceleration improves on CPU-only clusters by: 1) enabling the existing CPUs to process specific operators more efficiently, and 2) letting existing CPU clusters incorporate advanced processors that process many operations more efficiently than a CPU can.
Acceleration lets big data teams double their performance (or better) without needing to add new racks or servers or cloud instances. Even for approaches that introduce new hardware - such as GPUs and FPGAs - total cost of ownership can be 40-90% lower than traditional scaleout.
Smooth seas don’t make great sailors
Market analysts don’t expect this chip shortage to go away soon, and it will continue to impact businesses and consumers. As the saying goes, “smooth seas don’t make great sailors.” In the face of a challenging economic environment, data management leaders will deploy innovative approaches like these as historical approaches become increasingly impractical.