Darktrace Acquired by Private Equity Firm Thoma Bravo in $5 Billion Deal
Why real-world data matters to patients
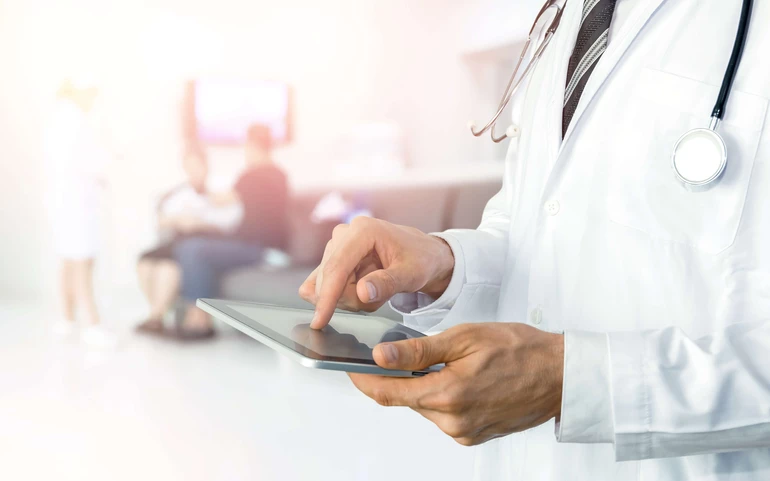
Healthcare systems globally are increasingly recognising the value of healthcare data. From developing improved care pathways to driving cost savings through data analytics, arguably the true value of healthcare data lies in patient outcomes.
Good data can power better, safer and more effective models of care for patients. As technology unlocks new data sets and more sophisticated tools of analysis, our ability to harness information will be key to driving better health outcomes and more sustainable models of care.
Yet while the value of ‘longitudinal, real-world data’ is widely recognised by healthcare organisations, policy advisors, research & academia, and pharma, more could be done to make patients aware of this data, and how they can contribute to both their own improved outcomes and those of other patients, insist Samir Dhalla, Head of THIN and Richard Ballerand, Co-Chair of THIN’s Patient Advisory Committee.
Public Health Benefit
Patient awareness of the importance of public health data has without doubt increased during the COVID-19 pandemic. From the initial use of NHS data to identify those 2.2 million individuals who were advised to shield to the on-going tracking and analysis to better understand the different COVID-19 patient experiences – such as the risks associated with obesity and Type 2 diabetes – UK citizens are becoming increasingly aware of the relevance of real-world health records.
National and international collaboration has also escalated rapidly in response to COVID-19. The UK government highlighted the importance of data in managing the pandemic and has created the NHS COVID-19 Data Store. The European Health Data & Evidence Network’s (EHDEN) took part in the OHDSI COVID-19 Virtual Study-a-thon which rallied 330 researchers with very different backgrounds and from thirty different countries and also launched its COVID-19 Rapid Collaboration Call in a bid to plug the gaps in fragmented, siloed and poorly interoperable real-world health data in order to characterise patients, manage their care and assess treatment safety.
The importance of population health data in both identifying and managing such pandemics cannot be overstated. Analysis of 16.2 million anonymised longitudinal patient records revealed a significant increase in the proportion of lower respiratory tract infections diagnosed by late 2019 compared to previous flu seasons, raising the odds of UK COVID-19 cases earlier than previously thought. By harnessing the information in electronic health records, such findings clearly open path to near real-time tracking of large-scale population health events that can support public health bodies to detect, investigate, and respond timely and effectively to them.
Reinforcing Value
The dialogue surrounding patient data is changing, however it remains important to recognise wider trust issues affecting the use of all data. A number of studies have confirmed that people are generally comfortable with anonymised data from medical records being used for improving health, care and services, for example through research. However, this is dependent upon a perception of public benefit; individuals are concerned about health data being accessed by commercial organisations. It is important to emphasise the broader value of patient data.
Speed of diagnosis is one of the most visible aspects of effective use of patient data. The ability to overlay anonymised patient-level data onto NICE guidance, as well as local and national patient pathways, provides more insight into what treatment may work better for cohorts of patients based on subsections of similarities, such as existing conditions and co-morbidities. Delving deeper into this data to examine the influence of age, , weight, faith, ethnicity and lifestyle choice, is helping clinicians to get closer to the best treatment first time.
Pancreatic cancer is a prime example of a disease that is often diagnosed late and progresses quickly. The deadliest common cancer, by the time a diagnosis is achieved, many individuals are at Stage three or Stage four and 75% do not survive a year after diagnosis. The symptoms – including unexplained weight lost, jaundice, abdominal pain and indigestion- can be vague, and easily confused with other conditions such as pancreatitis, gallstones, irritable syndrome (IBS) or hepatitis. For both GP and patients, embedding this information within clinical systems would be hugely beneficial, enabling far earlier diagnosis and hospital referral. The GP would spend far less of the budget on one specific patient and that patient has received the best treatment possible for that diagnosis and faster referral to the right clinician.
Predictive Modelling
Real-world data can also help to better understand patient responses to treatment and surgery. Combining data with predictive modelling to explore outcomes, pharma can work with healthcare services to get closer to ‘the right medicine, to the right patient, first time’. By tailoring and marketing specific medicines to cohorts of patients, the potentially arduous ‘trial and error’ process to find the appropriate treatment can be reduced.
For example, analysis of cardiac surgery patients has evaluated the risks for certain types of heart surgery as well as post-surgery, taking into consideration a number of factors, including age and ethnicity to improve recovery.
This can be extended to provide individual patients with information about their health conditions and risks to empower and encourage them to make changes to lifestyle or behaviours. This Predict, Preventive and Personalised Medicine (PPPM) explores complex mix of personal and population health data to avoid future health deterioration or detect problems before they arise.
Data Volume
Key to this process is early identification of potential health issues, which requires proactive collection of patient health data – from weight to cholesterol and blood pressure readings. Understanding the trajectory of these readings over the past year, combined with predictive modelling as to the potential outcome if no preventative measures are taken, will help clinicians to present personalised patient advice.
Ultimately, PPM will help patients to take control of their own health issues. Retaining individuals within primary care will both save money by reducing the pressure on secondary care and release investment in other areas of high demand healthcare. On a broader level, this detailed predictive model will allow the healthcare system to potentially predict population health issues over the next three to four years, helping to allocate resources to the correct specialties at the right time.
Critical to the success of these models is data volume: a large volume of anonymised, reliable patient data is required to support in depth analysis and informed decisions. While there is a global awareness of the value of this data, many researchers are compelled to rely on synthetic datasets which mirror real-world data, to support the development of medical technologies – such as those being used in the response to COVID-19. Such data simply fails to deliver the depth of insight delivered by real-world patient data.
Conclusion
The dialogue surrounding patient data has without any doubt evolved over the past few months but it is important to reinforce the value of patient data in supporting both individual and population health. Primacy Care data is being utilised to inform patient pathways across a range of disease areas and enabling better understanding of local health economies. GPs have a chance to inform life-changing medical research, supporting research crucial to gaining insights and developing policies, and helping to highlight trends in clinical effectiveness within the NHS. Patients have the opportunity to enable extraordinary changes in diagnosis and preventative care.