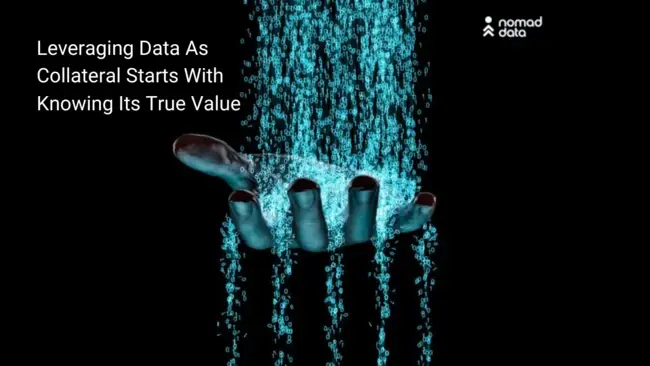
It isn’t just the December chill rolling in. The frosty winds of recession are blowing steadily and prompting many companies to brace against a global financial downturn. While many in the tech sector are opting to cut costs through staff cuts and hiring freezes, companies without such a payroll cushion are seeking ways to squeeze value from every asset they have.
The time is ripe for a fresh look at data as an asset. Though often dismissed when performing a business valuation or gathering collateral for a loan, data may actually hold more value for some businesses than anything else on the balance sheet.
Data as collateral is catching on
Big players are already leveraging data to weather tough economic times, like when United Airlines and American Airlines secured multi-billion dollar loans backed by customer loyalty data at the onset of the pandemic. In contrast, small and medium-sized businesses (SMBs) face a much more difficult path to data collateralization.
This is a shame because data as collateral has unique upsides for both lender and borrower. A borrower can leverage its data for non-dilutive funding, enabling founders to retain equity and maintain control of their company. Compared with traditional venture debt, the stakes are lower when data is put up as security. Even in default, the worst case scenario is that the lender retains a copy of data assets to sell and the borrower keeps the original version, with no interruptions in operation. Contrast that with the seizure of physical assets or a hostile creditor takeover, and the terms clearly are friendlier.
Data assets also offer unique perks for the lender. Data is a progenerative, non-depleting, and non-exclusive asset that can be broadly monetized to satisfy a defaulted loan. Lenders also can investigate data assets to reveal an intimate and up-to-date portrait of a borrower, both before and during the term of a loan. Data can be the canary in a coal mine, telling the true story of a company’s health well before any other lead indicator.
Valuing the intangible
Getting a lender and a borrower to agree on the value of a given data set is a big stumbling block. While there are mature models for assessing value of physical assets like real estate and inventory, data is intangible and a novel asset class. Still, there are third party experts and consulting firms that do offer valuation services for data and other intangible assets that lend some common ground to loan proceedings.
While any given consultancy may choose to keep exact valuation methodology proprietary, some of the common valuation methods for data include the relief from royalty method (RRM) and the cost basis method. Both methods account for the direct financial value the business generates from its datasets, but differ in assigning costs to holding the data assets themselves.
RRM is rooted from other intangible asset classes like trademarks and copyrights, and estimates the hypothetical royalty payments to lease an asset from a third-party licensor. Cost basis instead calculates the price to produce or buy the data, taking into account factors like research and development, storage and server costs, and labor.
Intangible asset valuation can be labor and time intensive, with even the most well-heeled consultants taking weeks or months to deliver. The expertise required also can command a hefty price tag, often in the neighborhood of a quarter million dollars.
Traditional data valuation misses the mark(et)
Perhaps the greatest challenge with traditional data valuation is the lack of marketplace comps. While some valuation models make attempts to determine a willingness to pay for data, the fundamental lack of transparency in the data brokerage market obscures market rates and potential buyers.
At a 30,000 foot view, the data marketplace is massive, dispersed, and highly opaque. Spanning more than 5,000 firms worldwide and growing, the global market for data is forecast to grow $462 billion by end of the decade according to Transparency Market Research. However, these marketplaces and exchanges do not publish transaction details. And with many data exchanges still peer-to-peer, relying on homegrown solutions or purpose-built platforms like Revelate, it can be difficult to understand how the markets function in practicality nor what the supply and demand are for different kinds of data.
Some emerging players in the data valuation space are leveraging search and AI technologies to pierce that veil and tap directly into these markets. One company, Nomad Data, a search platform for 3rd party data has begun collecting and analyzing such metadata. And Gulp Data, a neo-lender offering data-backed loans, uses machine learning trained on thousands of data sets from active markets to perform data valuations in a matter of hours, instead of weeks and months. By tracking the data liquidity markets on various data exchanges with over 15 billion records listed, they have real-time visibility into true market demand for data. They also can spot differences in demand between different markets globally and reveal specific buyers, unlocking the mysteries of monetization and offering true market comps.
Nimble, technology-backed valuations like these will become increasingly necessary as data markets evolve and demand rises from companies looking to leverage their data assets. These tools, in conjunction with true expertise, can knock down some of the barriers to entry for SMEs in leveraging their data and democratizing access to the burgeoning global information market. Making the most of what data you have means first understanding what it is worth. Fortunately the path to understanding data’s real market value is becoming clearer.