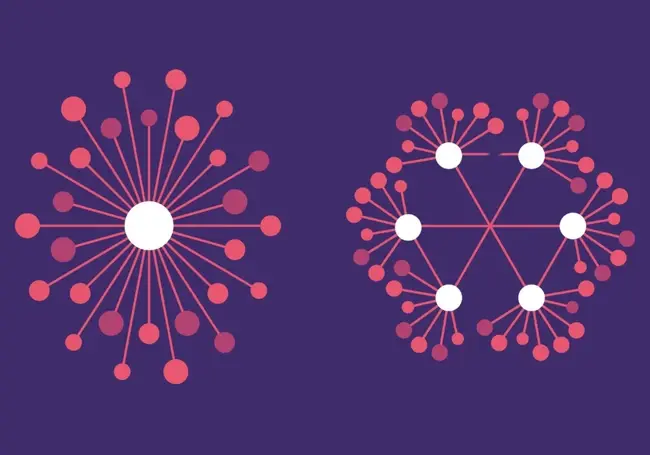
Fully centralizing your data environment is infeasible due to data gravity, migration costs, ad-hoc projects, and lock-in risk. So how do you balance?
Modern data teams contend with a patchwork of tools and platforms that span data centers, clouds, and regions.
They struggle to manage and prepare all these distributed inputs to feed analytics initiatives, especially those involving voracious AI models.
Emerging approaches offer part of the solution.
1. The data mesh proposes that business owners publish standardized data products to the rest of the organization.
2. The data fabric offers a common platform for integrating, cataloging, and governing assets across diverse environments.
But in the end, data leaders face a complicated balancing act: support innovation where you can; control and govern where you must.
Here's my take on what this means for key dimensions of modern data environments, working from the bottom up in this diagram.
Operational: decentralized
Data sources include operational applications, databases, files, IoT sensors, and so on. These often persist on-premises and reside on one or more cloud platforms.
Analytical targets: in between
Over the last decade, companies have centralized as much analytical data as possible onto cloud-based data warehouses, data lakes, and now lakehouses.
But centralization only goes so far. Some data is costly to replicate, distributed teams often spin up new platforms, and companies should try to avoid locking into just one cloud platform.
Data mgt: in between
Companies consolidate tools where possible for pipelines, observability, MDM, etc. But different tools specialize in different use cases, data types, and platforms, making it impossible to fully centralize.
Analytics: it depends!
Many analytics projects start with distributed teams within the business units. They spot business pain, and then have data analysts or data scientists tackle the problem.
Central data teams often expand analytics projects that succeed to other business units. They also manage corporate analytics projects.
Best practices
Wherever possible, central data teams should promulgate best practices across the company, for example with Centers of Excellence.
Governance programs
Wherever possible, central governance teams should build and enforce policies to mitigate risks related to privacy, accuracy, compliance, etc.
Would welcome feedback from data leaders. We're exploring this topic on a few fronts here at BARC. For example, Carsten Bange discusses centralization and decentralization with data leader Santiago Tacoronte of Mondelēz International in BARC's latest Data Culture Podcast. I listened to it twice.
https://barc.com/media/podcast-episode/centralization-vs-decentralization/