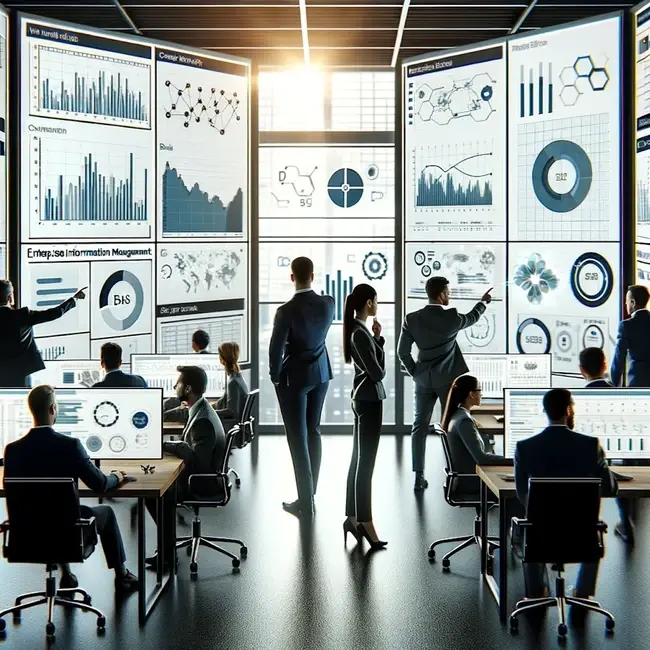
Companies generally struggle with collecting, storing, managing, discovering and distributing enterprise information. Knowledge management solutions have been around for many years, yet have mostly fallen short of a full solution for use across the business. There are many reasons for it, and we’ll take a look at those reasons and how to overcome them, but overall the problem gets worse every year, as every business has more and more information to deal with.
There are several reasons why businesses often struggle with effectively managing their data, information, and knowledge:
Data volume and variety: Companies generate and collect vast amounts of data from various sources, such as transactions, customer interactions, social media, and IoT devices. Managing such large volumes and diverse types of data can be challenging.
Data silos: Different departments or business units often store data in separate systems or databases, creating data silos. This makes it difficult to gain a comprehensive view of the data and share information across the organization.
Data quality: Ensuring data accuracy, consistency, and completeness can be challenging, especially when data comes from multiple sources. Poor data quality can lead to incorrect insights and decision-making.
Legacy systems: Many organizations rely on outdated or legacy systems that are not designed to handle modern data management requirements. Integrating these systems with newer technologies can be complex and time-consuming.
Lack of data governance: Without proper data governance policies and procedures, organizations may struggle to maintain data consistency, security, and compliance. This can lead to data misuse, breaches, or legal issues.
Unstructured data: A significant portion of company data is unstructured, such as emails, documents, and multimedia content. Capturing, storing, and analyzing unstructured data requires specialized tools and techniques.
Knowledge capture and sharing: Capturing tacit knowledge held by employees and making it accessible to others can be challenging. Encouraging knowledge sharing and collaboration across the organization requires a supportive culture and the right tools.
Rapidly evolving technologies: The data management landscape is constantly evolving, with new technologies and best practices emerging regularly. Keeping up with these changes and adapting the organization's data strategy accordingly can be difficult.
Skills gap: Managing and analyzing data requires specialized skills, such as data science, data engineering, and data visualization. Many organizations struggle to find and retain talent with these skills.
Budget constraints: Implementing and maintaining robust data management systems can be costly, and organizations may struggle to allocate sufficient budget and resources to address these challenges effectively.
Addressing the Knowledge Challenges
Companies can address the challenges of managing data, information, and knowledge by implementing a combination of organizational, technological, and process-oriented solutions. Here are some approaches and the role of AI and generative AI in supporting these efforts:
Develop a comprehensive data strategy: Create a clear data strategy aligned with business goals, covering data governance, architecture, security, and quality. This strategy should consider the role of AI and generative AI in data management and analysis.
Implement a data governance framework: Establish policies, procedures, and responsibilities for managing data throughout its lifecycle. This includes data quality management, data security, data privacy, and compliance with regulations such as GDPR or HIPAA.
Invest in data integration and data management platforms: Use tools like data lakes, data warehouses, and master data management systems to integrate and manage data from various sources. AI and generative AI can help automate data integration, data cleansing, and data transformation processes.
Encourage a data-driven culture: Foster a culture that values data-driven decision-making and encourages data sharing and collaboration across the organization. Provide training and support to help employees understand and utilize data effectively.
Leverage AI and generative AI for data analysis and insights: AI and generative AI can help businesses extract valuable insights from large volumes of structured and unstructured data. Some applications include:
Predictive analytics: AI can identify patterns and predict future trends, enabling proactive decision-making.
Natural Language Processing (NLP): AI can analyze unstructured data like customer feedback, social media posts, and support tickets to identify sentiment, topics, and trends.
Anomaly detection: AI can detect unusual patterns or anomalies in data, helping to identify potential issues or opportunities.
Implement knowledge management systems: Use tools like knowledge bases, wikis, and collaboration platforms to capture, store, and share knowledge across the organization. AI can help automate knowledge discovery, tagging, and organization, making it easier for employees to find relevant information.
Invest in data literacy and skills development: Provide training and resources to help employees develop data literacy and the skills needed to work with data effectively. This includes data analysis, data visualization, and data storytelling skills.
Establish data-driven business processes: Integrate data and analytics into core business processes, such as marketing, sales, and operations. Use AI and generative AI to automate and optimize these processes where possible.
Collaborate with external partners: Work with external partners, such as data providers, analytics firms, or AI companies, to access specialized expertise and resources to support data management and AI initiatives.
Continuously monitor and improve: Regularly assess the effectiveness of data management and AI initiatives, and make iterative improvements based on feedback and results.
Using Generative AI in Knowledge Management
Generative AI has the potential to revolutionize how businesses handle enterprise search and knowledge management. While it might not entirely replace current solutions, it can act as a powerful enhancement. Here's how:
More Natural Language Interaction: Generative AI can understand the intent behind a user's query, even if it's phrased poorly. This eliminates the need for complex keyword searches and allows users to ask questions in a natural way, like they would a colleague.
Intelligent Information Retrieval: Instead of just delivering documents, generative AI can analyze them and provide summaries, key points or even directly answer the user's question in a concise way. This saves time and effort spent sifting through irrelevant information.
Personalized Search: Generative AI can learn from user interactions and search history to personalize results. This means users will see information most relevant to their role and current tasks, improving efficiency.
Knowledge Discovery and Insights: Generative AI can go beyond simple retrieval and analyze vast amounts of data to uncover hidden patterns and relationships. This can lead to new insights and inform better decision-making.
Content Generation: Generative AI can create new content, such as product descriptions, marketing copy, or even code snippets, based on existing data and patterns.
However, some challenges exist:
Data Quality and Training: Generative AI models rely on high-quality data for training. Inaccurate or incomplete data can lead to unreliable outputs.
Explainability and Bias: It can be difficult to understand how generative AI arrives at its answers. This lack of explainability can raise concerns about bias and accuracy.
Overall, generative AI offers a promising new approach to enterprise search and knowledge management. By overcoming the current limitations, it can empower businesses to unlock the true potential of their information resources.
Process Enhancements
To support these efforts, organizations need to establish business processes that enable the effective management and utilization of data, information, and knowledge. Some key processes include:
Data governance processes: Processes for data quality management, data security, data privacy, and compliance.
Data integration processes: Processes for data ingestion, data cleansing, data transformation, and data consolidation.
Analytics and AI processes: Processes for data analysis, model development, model deployment, and model monitoring.
Knowledge management processes: Processes for knowledge capture, knowledge organization, knowledge sharing, and knowledge maintenance.
Change management processes: Processes for managing organizational change, user adoption, and continuous improvement.
By implementing these solutions and processes, and leveraging the power of AI and generative AI, companies can effectively capture, store, manage, discover, and distribute their data, information, and knowledge, driving better decision-making and business outcomes.