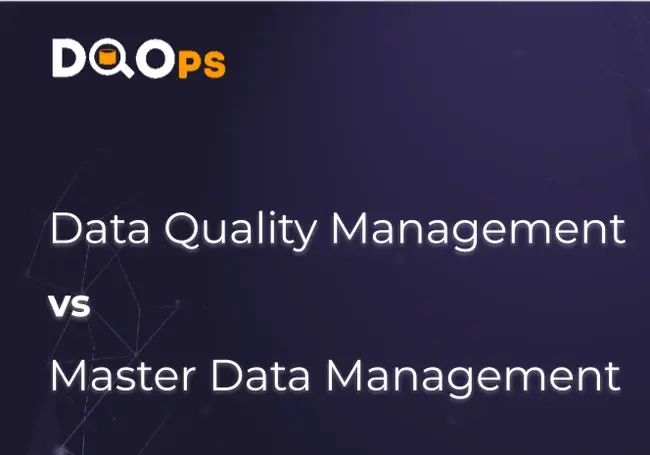
In today's data-driven world, accurate and trustworthy data is essential for success. "Data quality" and "master data management" are two concepts frequently discussed in organizations striving for data excellence. Both are critical, yet they serve distinct purposes, often leading to confusion among data professionals. This article aims to clarify the differences and highlight why both are crucial for any organization seeking to unlock the true potential of its data.
While both contribute to overall data health, their approaches differ significantly. Data quality management (DQM) casts a wide net, employing a methodology rooted in quality assurance principles. Think of it as the data equivalent of Six Sigma, meticulously assessing vast volumes and diverse datasets to identify and rectify inconsistencies, errors, and gaps. Master data management (MDM), on the other hand, adopts a laser focus, meticulously ensuring the accuracy and consistency of individual records within critical domains like customer data or product information.
This fundamental difference in scope and approach often leads to confusion. To truly understand their distinct roles, we need to delve deeper into the origins and methodologies of each.
Data Quality Management
Data quality management (DQM) takes a holistic approach to ensuring data integrity across an organization. It's not just about fixing errors; it's about establishing and maintaining standards that guarantee data remains reliable and fit-for-purpose. Imagine it as a continuous cycle of monitoring, analysis, and improvement, applied across a vast landscape of data assets.
DQM leverages various techniques to assess and enhance data quality. Key performance indicators (KPIs) provide quantifiable measures of data health, allowing organizations to track progress and identify areas needing attention. These KPIs might include things like the percentage of complete records, the conformity to data format standards, or the timeliness of data updates. By monitoring these metrics, organizations can gain a clear picture of their overall data quality and pinpoint specific areas for improvement.
To manage the sheer volume of data, DQM systems often employ automated monitoring and notification systems. These systems act like vigilant watchdogs, constantly scanning data for anomalies and alerting relevant teams when potential issues arise. This allows for prompt intervention and prioritization of remediation efforts, ensuring that critical data problems are addressed swiftly.
Furthermore, DQM relies on a framework of data quality dimensions to evaluate data against specific criteria. These dimensions provide a structured approach to assessing data completeness, accuracy, consistency, timeliness, validity, and uniqueness, among other factors. By evaluating data against these dimensions, organizations can identify specific weaknesses and tailor improvement strategies accordingly.
In essence, DQM is about proactively managing data quality across the entire data lifecycle. It's a discipline that combines technology, processes, and people to ensure data remains a trusted and valuable asset for the organization.
Master Data Management
Master data management (MDM) focuses on creating and maintaining a single, authoritative source of truth for the most critical data entities within an organization. These "golden records" represent core business concepts like customers, products, employees, or locations, and their accuracy is paramount for operational efficiency and informed decision-making. Unlike DQM's broad scope, MDM drills down to the individual record level, ensuring that every piece of information is meticulously validated and reconciled.
Think of MDM as a meticulous curator, carefully collecting and refining data from various sources to create a definitive representation of each entity. This often involves complex matching and merging processes to identify and eliminate duplicates, resolve inconsistencies, and enrich records with supplementary information. For example, an MDM system might combine customer data from multiple systems, such as CRM, billing, and marketing platforms, to create a unified and comprehensive customer profile.
Due to the granular nature of MDM, it often necessitates a higher degree of human intervention. Data stewards play a crucial role in this process, using their domain expertise to resolve data conflicts, validate information, and ensure data adheres to defined standards. They act as guardians of data quality, ensuring that each golden record is accurate, complete, and up-to-date.
While MDM might deal with a smaller volume of records compared to DQM, its impact is significant. Accurate master data provides a solid foundation for business operations, enabling consistent reporting, improved analytics, and more effective customer engagement. By creating a single, trusted source of truth, MDM helps organizations avoid costly errors, streamline processes, and make better-informed decisions.
In essence, MDM is a specialized discipline that focuses on the quality and consistency of the most vital data assets. It's a collaborative effort between technology, processes, and people, all working together to ensure that master data remains a reliable and valuable asset for the organization.
Why Scale Matters
The explosion of data in recent years has brought with it a growing reliance on external sources. While partnerships and third-party data providers offer valuable insights, this trend diminishes the level of control organizations have over their data landscape. It often favors quantity over quality, creating new challenges for data management.
Acquiring datasets from external business partners necessitates a shift in perspective. While data quality remains crucial, it's no longer economically feasible to scrutinize every single record with the same rigor applied to master data. The sheer volume of data makes this approach impractical and inefficient.
Furthermore, the rise of real-time analytics and interactive dashboards has added another layer of complexity. Users expect instant access to information, forcing data engineering teams to move away from traditional, scheduled reporting models. Data lakes and lake houses have emerged as popular solutions, providing centralized repositories for large volumes of data from diverse sources.
However, these platforms introduce their own set of data quality challenges. Data transformations, inherent in the data pipeline process, can introduce errors or inconsistencies. More importantly, data lakes are secondary data stores, meaning that any direct modifications made to the data within them are susceptible to being overwritten during the next synchronization with the primary source.
This issue is further compounded by the increasing prevalence of SaaS-hosted business applications. These platforms store data in databases managed by external vendors, making direct data remediation difficult, if not impossible. Data stewardship teams often resort to updating replicated copies of the data and then using complex scripts and APIs to propagate those changes back to the SaaS platform.
This is where data quality management truly shines. By identifying tables with significant quality issues within the data lake, DQM teams can prioritize data cleansing efforts and justify the investment in automated remediation tools. When data quality metrics reveal a substantial number of invalid records, building a custom tool to continuously fix those issues becomes a strategic imperative. DQM provides the framework and insights necessary to make informed decisions about data quality investments and ensure that data remains reliable, even at scale.
Comments ( 0 )