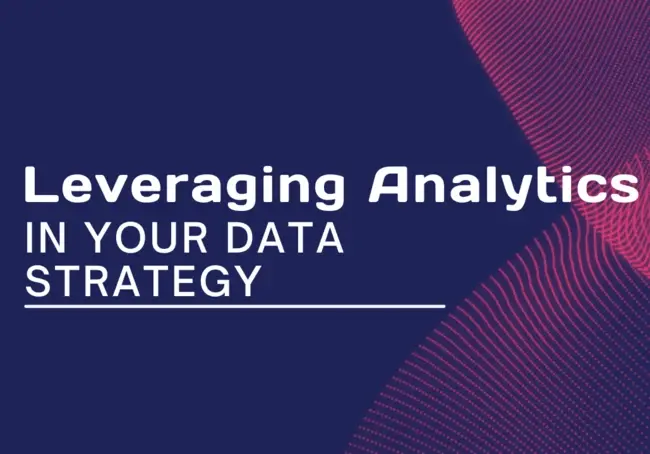
From testing a product feature to preparing for the potential macroeconomic slowdown, businesses rely on data and leveraging analytics to ensure the effectiveness of their decisions and policies.
While data can comprise unstructured assets, they no longer pose a challenge thanks to modern analysts’ machine learning (ML) models. So, most brands have integrated analytics to enhance their strategies. This post will elaborate on how to leverage analytics in your data strategy.
What is Data Analytics?
Data analytics involves computer-aided statistical problem modeling that identifies patterns and insights after processing multiple data resources. For instance, an analytics operation can describe how the user’s company performed compared to its rivals’ last business quarter.
Analysts, the professionals specializing in this domain, can also estimate how a decision or strategy will improve or decrease a company’s sales revenue. Similarly, reputed predictive analytics services extend the scope of conventional analytical programs to events that are yet to take place. These technological wonders enable corporations to upgrade their risk management and financial forecasts.
Automating data collection and transformation through artificial intelligence (AI) accelerates insight exploration. However, experienced data analysts’ oversight is crucial across all data processing phases to prevent biased results. They also know how to optimize data gathering and formatting using a well-crafted data strategy and multidisciplinary team coordination.
What is a Data Strategy?
A data strategy describes the scope and interdependencies between data operations like validation and reformatting. Its application extends to legal data processing compliance measures, including determining appropriate encryption and firewall technology integrations.
Therefore, corporations leverage comprehensive data strategy and consulting offerings that are scalable, accessible, and business relevant. Doing so enables them to enhance their data collection and transformation activities by borrowing experienced professionals’ skills.
Multi-stakeholder coordination improves when a data strategy informs your IT teams and business unit leaders. After all, each data strategist must optimize the communication documentation to help less tech-savvy employees grasp the core operating protocols.
For instance, reputed data strategists often simplify technical ideas when creating skill development material for non-tech departments. As such, strategizing your data analysis and digital transformation initiatives requires efficiently educating all the employees. Otherwise, an on-paper strategy increases implementation delays due to confusion among workers.
Is Analytics Necessary for a Data Strategy?
Technological disruptions and everchanging consumer preferences make analytical modeling indispensable to data strategy creation. Today’s managerial leadership cannot depend on intuition, age-old adages, or empirical evidence to navigate the competitive landscape.
Thankfully, artificial intelligence (AI), machine learning (ML), and natural language processing (NLP) facilitate extensive data acquisition. So, enterprises can reduce the resources required to deliver reliable data analysis reports. These documents will also comprise business performance insights and risk-related discussions.
Since data strategy focuses on what to do to accomplish a performance milestone in the future, integrating appropriate analytics tools is a must. Furthermore, no two organizations can benefit from a standard data strategy. This fact highlights the urgency for data processing workflows optimized for every company’s unique use cases.
How to Leverage Analytics in Your Data Strategy
Varying use cases of data management and analytics often result from enterprise data strategies that must accommodate departmental objectives. The following applications demonstrate how an analytics-enhanced data strategy can facilitate impressive improvements.
1| Customer Insights for Retention Strategy
Customer analytics examines whether your marketing, sales, and customer service channels increase satisfaction among your client base. Consider the following queries.
-
Why did the customer purchase your products or services?
-
How did they discover your brand?
-
Also, how long did they use your offerings?
-
What might have prompted previous clients to select your competitors?
-
Can modifying some aspects of customer journeys increase acquisition and retention?
A global firm would require a lot of employees, capital resources, and high-end computers to investigate such queries. However, automated insight exploration platforms streamlining data processing are available worldwide. Using them, brands can gain insights into these queries. Later, they will revise their customer relationship management (CRM) approach to address retention or consumer engagement issues.
Data strategists must also embrace alternative data sources that leverage user-generated content (UGC) to augment customer persona definitions. For instance, influencer or not, most consumers love sharing their opinion of a brand or a product with their social media communities. Track these ecosystems like you would track consumer discussion forums or news-related brand mentions.
2| Optimizing Supply Chain Management (SCM)
Supply chain analytics can provide ideas for new opportunities across distinct transportation and raw material sourcing channels. It will also reveal secure methods to obtain equipment and resources at competitive pricing. Therefore, enterprises engaged in transboundary shipments or import-export trades can experience tremendous boosts in profit margins.
If you leverage SCM analytics reporting, you can adjust your data strategy to focus on critical factors affecting how much your company pays for materials. Given the rising popularity of value-based pricing and protectionist tariff hikes, it is essential to track the required parameters.
Has a supplier nation exploited market fluctuations through unreasonable price increments? Did your government impose additional duties on a few resources your industry peers have been importing? Is a supplier not complying with international laws, fare trade policies, or sustainability requirements? These inspections allow leaders to assess their supply chain strategies and associated challenges.
3| Data Quality Assurance
Data strategists can craft the best procedures, but using poor-quality data will turn them into ineffective ideas. So, data quality management (DQM) is vital to making a data strategy work as intended, and analytics techniques help identify quality-related obstacles.
An analyst might collaborate with a strategist to shortlist the top priority DQM consideration, as described below.
-
Relevance: A data strategy must direct all the stakeholders to collect data relevant to the target industry and market demographics. Simultaneously, analysts will use extract-transform-load (ETL) pipelines to filter out irrelevant business intelligence.
-
Integrity: Datasets containing records from social networking sites and media reports can contain errors. Therefore, eliminating or replacing incorrect and biased entries from the company’s databases is crucial.
-
Freshness: What your competitors did a decade ago has a negligible bearing on what your company must do to compete today. As a result, global firms leverage analytics to customize a data strategy that outlines what to do with outdated or historical intelligence assets.
-
Governance: Unauthorized modification of corporate data can disrupt workplaces, factory production, and stakeholder goodwill. So, a data strategy must encourage authentication and usage analytics implementation to combat “human risks” threatening data quality.
Conclusion
Every company’s modern data strategy will help its departments get and manipulate valuable databases to solve business problems. While data strategists can guide leaders in devising a robust data processing roadmap, they can do more with the help of predictive and diagnostic analytics tools.
Automation and NLP have proved that businesses can rely on advanced computing technologies for accelerated data collection, sorting, validation, and insight extraction. Moreover, strategists can perform scenario analytics to estimate how their decisions might affect a company’s quarterly progress.
Analytical models let organizations compare identical activities to determine their priorities and ensure efficient resource allocation per operation. This advantage saves the time and effort needed to upgrade current strategies at an enterprise. Ultimately, aligning all data processes with a client firm’s mission statement is at the core of analytics-based data strategy development.