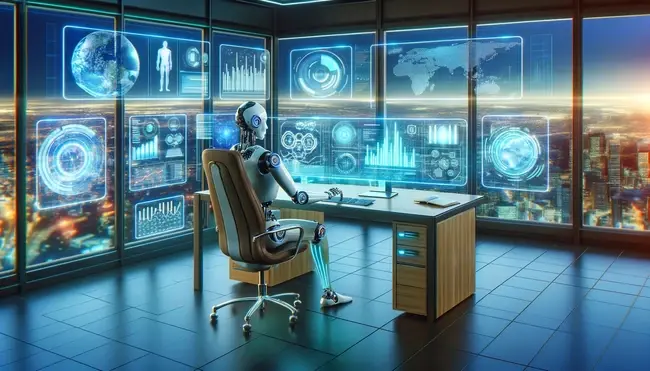
Business decision-making processes are undergoing a transformative change, thanks to the use of Artificial Intelligence (AI) and Data Analytics. These technologies are not just buzzwords but are real, practical tools that are reshaping how businesses operate, innovate, and grow. Here are a few ways that AI and Data Analytics are revolutionizing various aspects of business decision-making.
Predictive Analytics: Anticipating the Future
Predictive analytics is an advanced form of data analytics that uses historical and current data to forecast future outcomes with a high degree of precision. This approach enables businesses to anticipate market trends, consumer behaviors, and potential risks. It employs techniques such as data analysis, machine learning (ML), AI, and statistical models to identify patterns that might predict future behavior. Predictive analytics models are designed to assess historical data, discover patterns, observe trends, and use that information to predict future trends. For instance, a retail company might use predictive analytics to forecast seasonal demand for products, enabling more efficient inventory management.
Predictive analytics involves a variety of technologies and techniques. These include:
-
Data Mining: This involves the analysis of large sets of data to detect patterns.
-
ML: ML algorithms are used to find patterns and make predictions.
-
AI: AI is used to automate the creation of analytical models.
-
Statistical Models: These models are used to identify correlations between different elements.
-
Predictive Models: These models are used to forecast future outcomes based on historical data.
Predictive analytics finds application across a variety of industries and job functions. Some of the key use cases include:
-
Retail: Retailers use predictive analytics to analyze customers' buying behavior and predict future buying trends.
-
Fraud Detection: Predictive analytics can pinpoint abnormalities that indicate fraud. This is often used in the financial services industry.
-
Healthcare: In healthcare, predictive analytics can be used for diagnosis and predicting patient outcomes.
-
Finance: Predictive analytics can forecast future cash flow, revenue, profit margins and other financial metrics.
-
Manufacturing and Supply Chain: Predictive analytics can help optimize inventory management and identify factors that may lead to production downtime.
-
Marketing: Predictive analytics can forecast the performance of marketing campaigns and shape marketing strategies.
Predictive analytics can significantly improve business strategy in several ways:
-
Risk Reduction: By predicting future trends and patterns, businesses can anticipate potential risks and take preventive measures.
-
Operational Efficiency: Predictive analytics can help identify inefficiencies and streamline operations, thereby enhancing productivity.
-
Customer Behavior Analysis: By analyzing customers' buying behavior, businesses can tailor their products and services to meet customer needs.
-
Strategic Decision Making: Predictive analytics provides data-driven insights that can inform strategic decisions, helping businesses to navigate through challenges and align with market dynamics.
-
Performance Forecasting: Predictive analytics can provide an estimation of how a particular campaign or strategy is likely to perform, setting realistic goals and expectations.
Predictive analytics is a powerful tool that can help businesses forecast future trends, make informed decisions, optimize processes, and increase efficiency and profitability
Machine Learning for Market Analysis
ML is particularly adept at analyzing large datasets to identify patterns and insights that would be impossible for humans to discern. In market analysis, ML algorithms can sift through social media, online forums, and customer reviews to gauge public sentiment, extract insights and make predictions about products or brands. This real-time analysis helps businesses adjust their strategies promptly and effectively. Here are some applications of machine learning in market analysis:
-
Predictive Analytics in Market Analysis: ML algorithms can forecast market trends, consumer behavior, and stock prices by analyzing historical data. For instance, stock price prediction using machine learning involves training models like Long Short-Term Memory (LSTM) networks on historical stock data to predict future stock prices.
-
Sentiment Analysis: Sentiment analysis uses natural language processing (NLP) and ML to gauge market sentiment from text data such as news articles, social media posts, online reviews and financial reports. This helps businesses understand the overall attitude towards a particular market and adjust strategies and go-to-market (GTM) motions to maximize business performance.
-
Risk Management: ML models can assess the risk levels in businesses by identifying, assessing, and controlling threats to an organization's capital and earnings. These risks can stem from a variety of sources, including financial uncertainties, legal liabilities, strategic management errors, accidents, and natural disasters. Effective risk management is crucial for businesses to mitigate potential losses and enhance decision-making.
-
Market Research: ML can automate the analysis of vast datasets in market research, identifying patterns and trends that inform product development, marketing strategies, and GTM through competitive analysis. For example, a methodology developed by researchers at MIT uses convolutional neural networks to identify customer needs from user-generated content.
ML offers a multitude of applications for market analysis. With its ability to handle large volumes of data and uncover patterns, it’s a valuable tool for businesses looking to gain insights and make data-driven decisions in the marketplace.
Forecasting: Navigating the Business Terrain
Forecasting is an essential aspect of business strategy and planning. AI-driven forecasting tools can process and analyze data from various sources to make accurate predictions about sales, market trends, and economic shifts. These insights are invaluable for businesses to strategize their operations, marketing, and financial planning and plays a crucial role by enhancing accuracy, efficiency, and comprehensiveness. Here are some of the key ways AI contributes to forecasting:
-
Data Analysis and Pattern Recognition: AI algorithms excel at processing large volumes of data and identifying patterns and trends that might not be apparent to human analysts. This capability is essential for accurate forecasting, as it allows businesses to make predictions based on comprehensive data analysis.
-
Predictive Analytics: AI systems use historical data to predict future outcomes. In business forecasting, this means predicting market trends, customer behavior, sales, and financial outcomes. These predictions help businesses in strategic planning, budget allocation, and risk management.
-
Real-Time Data Processing: AI can analyze data in real-time, which is crucial for businesses operating in dynamic environments. This allows for more timely and relevant forecasts, enabling businesses to react quickly to changing market conditions.
-
Automated Learning and Adaptation: AI systems can continuously learn from new data and adapt their predictions accordingly. This means that forecasts can become more accurate over time, as the AI system refines its models based on the latest information.
-
Scenario Analysis: AI can simulate various scenarios based on different variables. This helps businesses understand potential outcomes in different situations, aiding in contingency planning and decision-making.
-
Customization and Personalization: AI can tailor forecasts to the specific needs of a business, considering unique factors such as industry specifics, company size, and market position. This customization leads to more relevant and actionable forecasts.
-
Reducing Human Error and Bias: AI algorithms, while not infallible, are less prone to the biases and errors that can affect human judgment. This leads to more objective and reliable forecasts.
-
Cost Efficiency: Automated AI systems can perform complex analyses more quickly and with fewer resources than traditional methods, leading to cost savings for businesses.
-
Integration with Other Technologies: AI can be integrated with other technologies like IoT (Internet of Things), blockchain, and cloud computing to enhance forecasting. For example, IoT devices can provide real-time data feeds that AI systems analyze for instant insights.
-
Enhanced Decision-Making: By providing more accurate and timely forecasts, AI empowers business leaders to make better-informed decisions, ultimately leading to improved business outcomes.
AI transforms business forecasting by providing more accurate, efficient, and adaptable tools for analyzing data and predicting future trends. This helps businesses to strategize more effectively and respond promptly to market dynamics.
Brainstorming New Products and Services
AI offers a range of opportunities for businesses to enhance their brainstorming processes for new products and services, or for improving existing ones by analyzing current market trends, customer preferences, and emerging technologies. AI can help businesses identify potential new products or services in the ideation phase of new product development (NPD). This approach reduces the risk involved in innovation and ensures that new offerings are aligned with market demand and customer expectations. Here's how AI can be utilized in this context:
-
Data Analysis and Insight Generation: AI can analyze large amounts of data, including market trends, customer feedback, and competitive landscape, to identify unmet needs or gaps in the market. These insights can inspire new product ideas or improvements to existing services.
-
Trend Prediction: By leveraging ML and predictive analytics, AI can forecast future trends and consumer behaviors. This predictive capability enables businesses to stay ahead of the curve in developing products or services that will meet future demand.
-
Enhanced Creativity: AI tools, like generative design software, can produce numerous design variations by setting certain parameters. This not only speeds up the creative process but also introduces novel concepts that might not have been considered by human designers.
-
Customer Feedback Analysis: AI can efficiently analyze customer reviews, social media comments, and survey responses to extract valuable insights about customer satisfaction, preferences, and pain points. This information can be crucial for improving existing products or services.
-
Collaborative Brainstorming Tools: AI-powered collaboration tools can facilitate brainstorming sessions, especially in remote or distributed teams. These tools can suggest ideas, provide relevant data, and even predict the success of proposed ideas based on historical data.
-
Simulation and Prototyping: AI can be used to simulate how a new product or service will perform in the market. This can include testing various scenarios and making adjustments before any physical prototype is created, saving time and resources. Digital twinning can be used to iterate and improve designs, and synthetic customers can “test” products and services virtually.
-
Content Generation: AI can generate creative content, including names, descriptions, and marketing materials for new products or services. This can be particularly useful in the ideation phase to visualize and articulate the concept.
-
Decision Support: AI systems can provide decision support by analyzing the potential risks and benefits of different ideas. This can help businesses make more informed decisions about which ideas to pursue.
-
Customization and Personalization: AI can help in brainstorming personalized products or services. By understanding individual customer preferences and behaviors, businesses can create tailored offerings, enhancing customer satisfaction and loyalty.
-
Efficiency in Idea Screening: AI can quickly sift through and evaluate a large number of ideas, prioritizing those with the highest potential based on set criteria. This streamlines the brainstorming process and ensures focus on the most promising concepts.
AI in business brainstorming enhances creativity, efficiency, and data-driven decision-making. It allows companies to quickly adapt to market changes, understand consumer needs better, and innovate with a higher likelihood of success.
AI and Data Analytics are not just enhancing business decision-making, they are redefining it. By leveraging these technologies, businesses can gain unprecedented insights, anticipate market changes, innovate effectively, and maintain a competitive edge in today’s fast-paced business environment. As these technologies continue to evolve, their impact on business decision-making processes will only grow stronger, leading to more efficient, customer-focused, and innovative business practices.