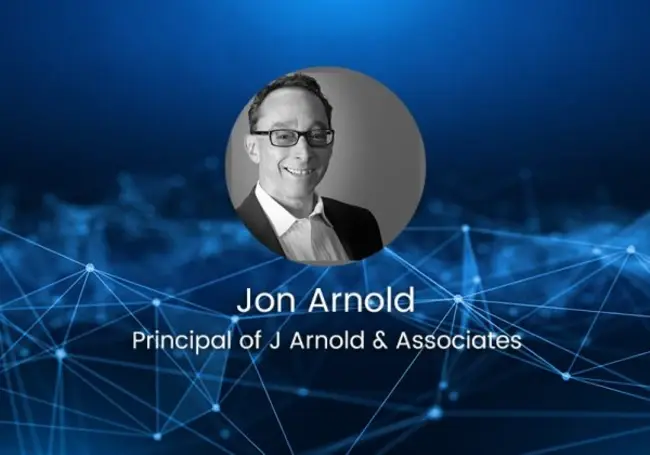
Speech technology has been evolving on many fronts, and while the changes are hard to follow, the benefits are very compelling. For enterprises, most of the use cases are in the contact center where customer expectations are becoming harder to address with legacy voice technologies.
Artificial Intelligence (AI) is driving most of this innovation, where speech recognition is being used to automate service and help agents engage more effectively with customers. Use cases are also emerging in the enterprise workplace to help employees streamline workflows, automate routine tasks and manage the never-ending flows of information.
We’re still in the early stages of applying AI technologies to speech, with the most familiar ones being Natural Language Processing (NLP), Natural Language Understanding (NLU), and Machine Learning (ML). All of these play a key role in working with unstructured data to enable AI-driven speech applications, with Alexa for Business being a prime example that workers can relate to.
Introducing Natural Language Generation
This may be how most IT decision-makers think of AI-driven speech, but there are more technologies to consider. In the course of my ongoing research as a technology analyst, I come across all kinds of innovation, and for this Spotlight article, my focus is on Natural Language Generation (NLG). This branch of the AI tree has been around for some time, but is just now coming into its own for enterprise applications. My intention here is to broaden your thinking about how AI-driven speech can bring new business value.
In brief, NLG addresses a different problem set than NLP/NLU, as the focus is on giving voice to data. Specifically, NLG is meant to extract actionable insights from vast amounts of data. We don’t normally think about voice-enabling data, but the output is similar what text-to-speech produces. That is, the content is converted to a different format that in many cases is easier to consume, and makes the underlying content more valuable.
Whereas NLP/NLU works with unstructured data – voice – NLG works with data, which is highly structured. Rather than focus on the challenges of removing ambiguity from language and understanding intent, NLG applies AI to create narratives around the data, which can then be consumed either in text or voice form. Much like Alexa or Cortana can serve as your personal virtual secretary to manage your daily schedule, NLG platforms can provide workers with a personal virtual analyst to manage various forms of data that otherwise require human effort.
To varying degrees, we all need to manage data – especially knowledge workers – and both the volume and complexity of data continues to grow. Most workers, however, have limited skills and/or inclination to effectively analyze data, and given how central data is becoming to everyday workflows, this can become a serious drag on personal productivity as well as organizational performance.
Enterprises have long struggled with this issue, and thanks to recent advances in AI, NLG is tailor-made to help workers get the most from the data that touches almost every aspect of their jobs. The underlying data science for NLG is complex and beyond the scope of this post, but in terms of outputs, NLG leverages AI to analyze your data and generate “narratives” that explain what the numbers mean. These narratives are user-defined, where workers can select various attributes depending on the desired outputs. The outputs can be text-based or voice-based, hence the connection to AI-driven speech.
Business Intelligence – a Prime Use Case for NLG
While the range of applications is practically limitless, perhaps the strongest enterprise use case at the moment is Business Intelligence – BI – where the supporting platforms and systems provide the richest source of company data used for all levels of decision-making. To illustrate, consider a common scenario such as a quarterly sales report. Instead of the worker reviewing the data and preparing a written report for the upcoming sales meeting, NLG can do all this automatically, freeing up their time for more challenging tasks.
A key reason why BI is a strong use case is that these platforms provide visual representations of the data, but this isn’t always helpful for workers. Not all forms of data can be easily visualized, and visual outputs aren’t always enough. Sometimes a written analysis is what’s needed, and other times voice is the format that works best.
The written report format is familiar, but the possibilities of a voice-based narrative of your data are new, such as using Alexa to initiate a BI query to get the key highlights from the latest quarterly results. Furthermore, NLG platforms enable workers to produce highly customized and personalized reports that go well beyond prepackaged report templates from a BI platform.
Equally important is the scalability of AI, where NLG can process vast amounts of data, so the tools are there to generate new and richer insights that a manual effort could easily miss. Add to that the speed of AI, where the benefit is the ability to generate reports faster than humans can do. Not only that, but this means that reports can be dynamically updated on the fly, such as when tallying real-time data for a survey poll.
There’s a lot to consider with NLG, and these brief examples only hint at the potential to get greater value from the data that’s both being generated by and housed within your enterprise. As conversational AI capabilities improve, the use cases for NLG will become routine, both for data-to-text and data-to-voice queries. Similarly, as trust builds with NLG to generate data narratives that are on par with human-based insights, workers will rely on these platforms for more complex forms of analysis.
Spotlight on Arria NLG
We are still in the early adopter phase with NLG, and being a Spotlight article, I’d like to cite the progress one of the leaders has been making lately. New Jersey-based Arria NLG has been at the forefront of research in this space, with 26 patents and deep expertise in their Scotland-based development center.
You’ll have to do your own research to explore their extensive capabilities, but the core offering is NLG Studio, and to support the BI use case outlined herein, their latest news is noteworthy. They recently announced the integration of NLG Studio with TIBCO Spotfire, adding to their extensive integrations with other leading BI platforms, such as Microsoft Power BI, Tableau and Qlik. This breadth of coverage validates the opportunity for NLG to bring an entirely new value layer to BI. Whether utilizing text or speech-based outputs, companies like Arria NLG are democratizing data for all workers, especially those who have lacked the ability to effectively harness it.
To conclude, I’ll focus on another important development that aligns with the title of this post. At the recent Tableau conference, the company announced Arria Answers, which provides conversational AI capabilities for their Studio platform. Text-based forms of NLG are rather straightforward, but AI-driven speech is more challenging.
The earlier mention of using Alexa for Business to interface with BI using speech is enabled by Arria Answers, but what’s notable is how this goes beyond the static question-answer model such as asking about last week’s sales figures. In the parlance of conversational AI, this would be termed a single-turn conversation. For AI-driven speech to be useful for more complex data analysis, multi-turn capabilities are needed. Instead of just providing last week’s sales figures, the conversation could continue with comparisons against previous quarters, or breakouts by line of business, etc. AI allows that conversation to continue by carrying context forward along the way, so the platform “knows” how the data sets relate to each other.
Not only does this deeper conversation provide richer insights, but the dialogue flow is more natural. There’s no need to repeat basic things each time to engage the application (that’s single-turn), and that’s what makes AI “conversational”. The importance of this capability should not be overlooked, since it characterizes ease of use, which is critical for driving end user adoption. AI is complex by nature, and its potential will only be realized when there’s a seamless user experience that makes it intuitive for everyone to use.
Arria NLG isn’t the only company bringing NLG into the enterprise, but if this topic is new for you, this Spotlight article will be a good starting point for what’s coming with AI-driven speech. Also, if you’re planning to attend Enterprise Connect 2020 next month in Orlando, FL, NLG will be one of the topics I’ll be addressing in my market update talk on speech technologies for the enterprise.
Comments ( 0 )