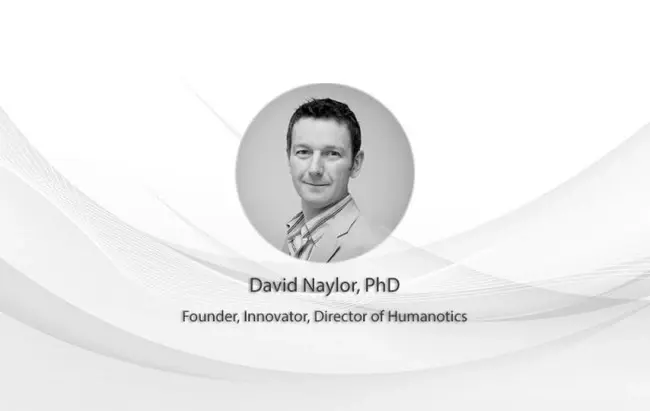
Artificial Intelligence research developments have hit the boardroom in recent years and the message going out to the rest of the organisation is “implement AI!” Unfortunately, there is a perilous rope bridge that hangs over a Chasm of Disillusionment when it comes to adoption and scaling up of effective Artificial Intelligence solutions. I visited the CogX Festival of AI this week during London’s Tech Week. It remains clear that most companies have barely taken the first step across this bridge in terms of embedding AI into their operations. So what’s the challenge? Implementing AI is so much more than buying a new piece of technology and deploying it – we all know that can be tricky enough. It’s less about ‘implementing’ and more ‘embedding’. This impacts across the operation in terms of processes, skills, organisational management and technology. It also needs a strategic approach which will avoid the business working in silos, as this naturally leads to duplication of effort and cost, plus the real chance that learning will not be shared between teams. When we talk about Artificial Intelligence, we are generally referring to the use of Machine Learning models that are trained (using historical data) to predict or optimise an output based on new input data. This could be applied to identifying a customer that may churn, optimising a production management process or summarising a report based on natural language understanding. Machine Learning has taken hold in the last few years over traditional algorithms because of its ability to handle more complex problems and deliver greater accuracy. Let’s briefly look at five important elements of an effective approach to AI deployment. Data is clearly at the heart of machine learning but that is not the key reason why you will already have solid data governance and information management process in place. Critically, do you have the right data to support your solution? The characteristics of data are often talked about as the Four Vs: Velocity, Veracity, Volume and Variety. I like to extend those with Visibility (what can you see in your data that could compromise GDPR?) and Value (how are you ensuring the value in your data is maintained within the organisation?). There’s a lot more to consider on the topic of data but just recognise that garbage in, garbage out absolutely holds true. I’m a big advocate of setting up a Centre of Excellence. This isn’t a team hidden away in some ivory tower thinking up the next big idea. It’s close to the business, leading the execution of the AI strategy, policy and governance, but also getting involved with real projects to help embed the AI technology within real world applications. This means working closely with all departments to understand business issues and educate them on the potential of AI. The key responsibilities of the AI Centre of Excellence are shown in the figure.
There are numerous examples of these teams being set up by utilities, telcos and financial services companies such as Aviva’s Digital Garage, which is also investing in start-ups, but perhaps with questionable results recently. However, they shouldn’t be the domain of only larger businesses. It is important for any organisation to establish this team, even virtually, to maximise opportunity with limited resources. I’d argue that it is the job of this team to make itself redundant in time by pushing the expertise out to the rest of the organisation. For this to happen, you need to ‘democratise’ your data and machine learning models. This means putting the power in the hands of the people who understand the problems they are trying to solve, but it will only work if everyone has better ‘data skills’. Airbnb is one company that has taken action to address this. It was already a data-centric business, but it recognised that not everyone had the skills to use the data it was making available. It introduced its Data University to provide all staff with the training needed to get more from the data at their fingertips. Some were trained in better use of Excel, while others have gone on to build their own machine learning models. Process adaption is another important consideration. AI will require new ways of working that optimise the process to get the best from it. This means considering the limitations it has or exploiting higher performance. For example, natural language understanding is increasingly being used to enhance the capabilities of quality monitoring in contact centre operations. To do this, the existing quality monitoring framework needs to be adapted to deal with the limitations of natural language applications. Word recognition is increasing in performance all the time – take a look at Google Duplex – but understanding the context of the conversation remains a challenge. It is therefore easier to understand what the customer is asking for, based on the first few words and sentences, than it is to know if the advisor followed the right process to resolve the request for the customer. The best approach is to split the monitoring into Compliance and Quality, using AI to analyse the first and humans to evaluate the second based on certain scenarios that the AI is looking for, such as inappropriate language or talk over. Finally, all these points touch on what is perhaps the biggest challenge, and that is access to the right skills. You need to consider two questions when evaluating this. First, what specialist skills do we need that we don’t have and must recruit? There are many organisations now that can support with specialist AI resources for short-term proof of concept work or much longer secondments that will allow you time to understand what you need and recruit the right people, without slowing down your progress. Second, how are we investing in our people so they can use AI to create longer term value for the organisation? The skills you need may include conversational design, chatbot engineering, DevOps, Agile project management, data science and decision engineering, to name a few. Being able to recruit these skills at this moment is a challenge, with high demand and salaries to match. By the way, this makes the shared resource pool of the Centre of Excellence even more attractive. Augmenting your peoples’ skills with AI will add long-term value to the business. The skills you need to develop may focus on enhanced operational roles to manage continuous model improvement or training on how to use the new AI powered tools themselves in their day to day role for maximum effect. It’s very easy to get drawn into AI technology – it’s interesting, shiny and new. It will remain on the margins of many organisations, failing to fulfil it’s potential because the approach to embedding it wasn’t thought through. Stepping out on to the rope bridge can be daunting, but running fast to the other side and hoping for the best is not the route to success.
Comments ( 0 )