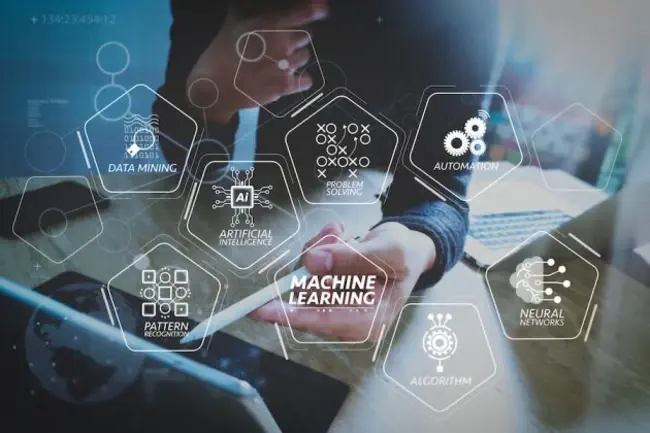
Larger organisations are reportedly leading the way when it comes to implementing machine learning (ML). This is according to Algorithmia's “State of Enterprise Machine Learning” survey, which also found that challenges to widespread adoption persist.
Last year, the company set out to "understand how companies are reacting to Machine Learning, its adoption in the marketplace, how businesses adapt, and how the industry is evolving." In order to do so, Algorithmia developed a survey with over 500 decision makers at companies with various employee sizes and industries.
Bigger companies are ahead
Overall, 80% of respondents said that their organisation's investment in artificial intelligence (AI) and ML had grown by at least 25% in the past year. However, this figure climbed to 92% in companies with over 10,000 employees.
Moreover, respondents within larger companies felt significantly more satisfied with their progress than those in smaller enterprises. In fact, they were 80% more likely to feel “satisfied” or “very satisfied” with their progress in comparison to companies with fewer than 500 employees.
These employees were also approximately 300% more likely to consider their model deployment as sophisticated. As a result, the report states that budgets are "growing faster for organisations that consider themselves sophisticated."
For example, 51% of the larger companies in the survey increased their AI/ML budgets by at least 25% this year. Despite this, many companies were unsure where their ML leadership will come from.
Lack of clarity
37% of respondents said that management are primarily directing their company's ML efforts. However, 55% stated that their efforts are emerging from engineers or other technical teams.
Data Scientists are also reportedly facing a lack of clarity from management. According to the report, Data Science and ML teams spend the majority of their time on infrastructure, deployment, and data engineering.
As a result, this leaves less than 25% of their time for training and iterating models. Therefore, just 8% of respondents said that they consider themselves “sophisticated” in their machine learning programs.
How important is data quality to AI and ML success? Take a look at Debugging Data to find out
Comments ( 0 )