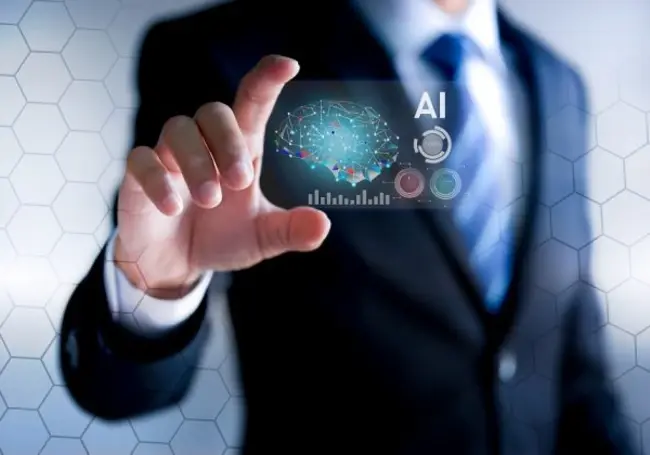
Artificial Intelligence solutions are on the rise. A recent study from Tractica discovered that the market for AI software products and services would surpass $89 billions by 2025. Moreover, investments in AI startups or solutions totalled more than $15 billions in 2017 alone. With these rapid growth rates ahead, there is no wonder that SMEs and enterprises alike are fighting to jump onto the AI trend. Don’t get me wrong that is fantastic. Many market participants generally mean innovation happens faster than usual. Plus, having businesses participate in the AI revolution helps everyone to get closer to the holy grail of developing General AI. Artifical Intelligence will transform from a particular, use case driven technology to an intelligent companion that makes sensible decisions.
What is Artificial Intelligence?
Artificial Intelligence is arguably one of the most debatable fields in contemporary science. Although modern AI it's rooted in the 1930s, we are still unsure how to define it. The recent media attention on the topic hasn’t helped with clearing these debates. Either because we lack better names or to jump on the trend; we now have many applications labelled as artificial intelligence when they are just statistical analysis or manually encoded “if-then” rules. Moreover, the field is still consolidating as old topics transition to other fields and new ones added to the forefront of the field. For example, automated search and planning used to be at the forefront of the AI research once, but is now something taught to undergraduates everywhere. Defining AI becomes even more tricky because we tend to associate intelligence to complex tasks such as chess, Go, or proving maths theorems, while tasks like walking, recognising our loved ones in images, or picking up soft objects are mere commonalities. In reality, AI algorithms excel at solving tasks like playing complex games because the rules are predetermined, and they can handle a much larger amount of information that we can. On the other hand, “soft” tasks are still close to a mystery. Take a look at the evolution of autonomous driving. We are still trying to figure out all the variables involved and the decisions that a human driver makes almost instinctively. Thus, we should be careful when defining something like an AI solution or system. It could be that it looks intelligent when in terms it is just performing a long list of complex operations.
Artificial Intelligence is an attempt to replicate human thinking and decision making. To some extent that is true, but artificial intelligence is about much more than replicating human cognitive abilities. Many applications and solutions today perform human tasks such as speaking, translation, or driving. However, AI solutions should reason, solve problems, acquire and use knowledge, make decisions, and communicate in natural language. The whole purpose of AI is to create systems that are more efficient, more effective, and more productive than humans are. In other words, AI systems should process unfathomable amounts of data in a timely fashion to arrive at practical solutions.
An AI algorithm is a method of computation when the steps towards solving a problem are unknown. In other words, artificial intelligence is using information about the situation to find out how to react to it. Most of the times, the starting point is a basic model of the problem domain and a bunch of data. The data are either labelled or unlabelled. The algorithm adjusts the parameters of the model to represent the data best. In other words, the algorithm adjusts such that its representation of the problem domain is in tune with what the examples reveal about the world. When the model gets to a state that best represents the world, the algorithm will stop learning. Thus, any solution that can improve its performance by learning from experience while performing tasks in complex environments without supervision can be considered artificial intelligence.
Narrow vs General AI
Artificial Intelligence seeks to create adaptive and autonomous systems. In its pursuit, it is common to go onto two parallel routes, either narrow (or weak) AI or general (or strong) AI. The first route leads to the systems that we see in practice today; highly specialised algorithms that are solving one very well defined task or problem. Siri is one example of narrow AI. Even if she appears to perform a plethora of functions, it can only operate within a given domain and is not self-aware.
In contrast, general AI (or strong AI) seeks to create a system that is self-aware and capable of solving any problem. Unfortunately, we are still far from building general AI applications, but the systems like Jarvis of Marvel’s Iron Man series are a good representation for general AI.
AI and Business
Although recent years witnessed an explosion in applications with a form or another of AI, business applications are still limited to a restricted set. Typical examples of AI solutions for business are in recommender systems for content or products, chatbots to ease customer service, intelligent virtual assistants to facilitate internal communication and workflows, fraud detection, bots to improve customer experience, or programmatic advertising and automated marketing solutions. Industrial sectors also benefit from AI developments mostly through intelligent control systems for industrial assets and processes, predictive maintenance, process monitoring and optimisation, autonomous trucks and other smart assets. Although overwhelming, these examples are only the tip of the iceberg when it comes to what AI can do.
Most of the applications I mentioned are prime examples of narrow AI. They are designed to resolve specific use cases. Nevertheless, these systems are working, and businesses have reported improvements in efficiency and productivity after adopting them. However, these improvements don’t come for free. Significant drawbacks on the road to broad AI adoption is not technical, but ethical and skills related. In a recent survey from InfoSys, senior business decision makers revealed that they are concerned about the lack of clear ethical standards when it comes to AI. Furthermore, business leaders are worried about the job displacement that AI will cause. However, AI is not just about automating processes and having machines perform human tasks. In reality, as AI adoption increases, new jobs will emerge from robotic and intelligent systems designers and builders to supervisors or other positions where creativity and intuition are essential. In a recent study, PwC study revealed sectors like professional services, science, and education would see a significant rise in the number of jobs available. Sectors, where people are performing repetitive tasks such as manufacturing or transport, will see a drop in jobs. Nevertheless, if people, business, and governments alike invest in the continual development of their employees' knowledge, especially in STEM subjects and arts knowledge, companies will only have to benefit from adding AI to their workforce.
The future of AI for business is not all gloomy. However, how can companies transition from single, isolated AI solutions to enterprise-wide systems? A solution is to create networks of specific AI solutions and intelligent agents. These agents adapt to their environment and are autonomous. In other words, they continually learn from situations and each other about how to best perform their tasks. Soon, intelligent agents augment the whole enterprise and support employees. As they grow and evolve, these systems become ubiquitous, from internal systems to monitor and control processes to client facing applications and services. Thus, the enterprise backbone shifts from human decision makers to a mix of software or hardware agents performing repetitive tasks and adaptive and autonomous agents augmenting people.