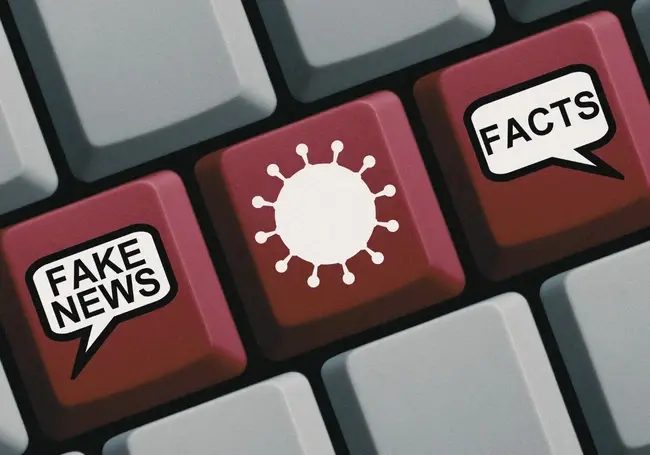
Especially since the COVID-19 lockdowns across the world, fake news has spiked to an uncontrollable extent.
The National Institutes of Health (NIH) reported that a trend analysis of fake news on COVID-19 verified by the International Fact-Checking Network and Google Fact-Checking Tools [13] showed a 900% increase from January to March 2020.
A new machine learning (ML) method could be the solution to tackling the unruly rise of misinformation around the globe which the current fact-checkers cannot optimally detect.
Recently, scientists from Ben-Gurion University have developed the ML approach to identify fake news sources using active learning algorithms that learn from human feedback and improve accuracy accordingly.
This model also examines the contribution of network, audience, and text features to the model's accuracy in the ambition to understand the reasons behind the spread of misinformation.
Fact-Checking Challenges
Dr Nir Grinberg, the research team leader, explained that today's challenge is with the proliferation of fake news where fact-checkers are overwhelmed.
“They cannot fact-check everything, but the breadth of their coverage amid a sea of social media content and user flags is unclear,” he told TechXplore.
“Moreover, we know little about how successful fact-checkers are in getting to the most important content to fact-check," added Dr Grinberg.
This vagueness associated with the current fact-checking methods prompted the researchers to develop a new machine learning (ML) approach.
The new method aims to help fact-checkers direct their attention better and boost productivity.
69% Higher Accuracy In Real-Time Fake News Detection
Scientists carried out tests extensively to evaluate the performance of both offline (historical data) and online (real-time) settings.
They discovered that the ML model outperformed other common misinformation detection methods by 33% in historical data and a staggering 69% when observing online – real-time sources.
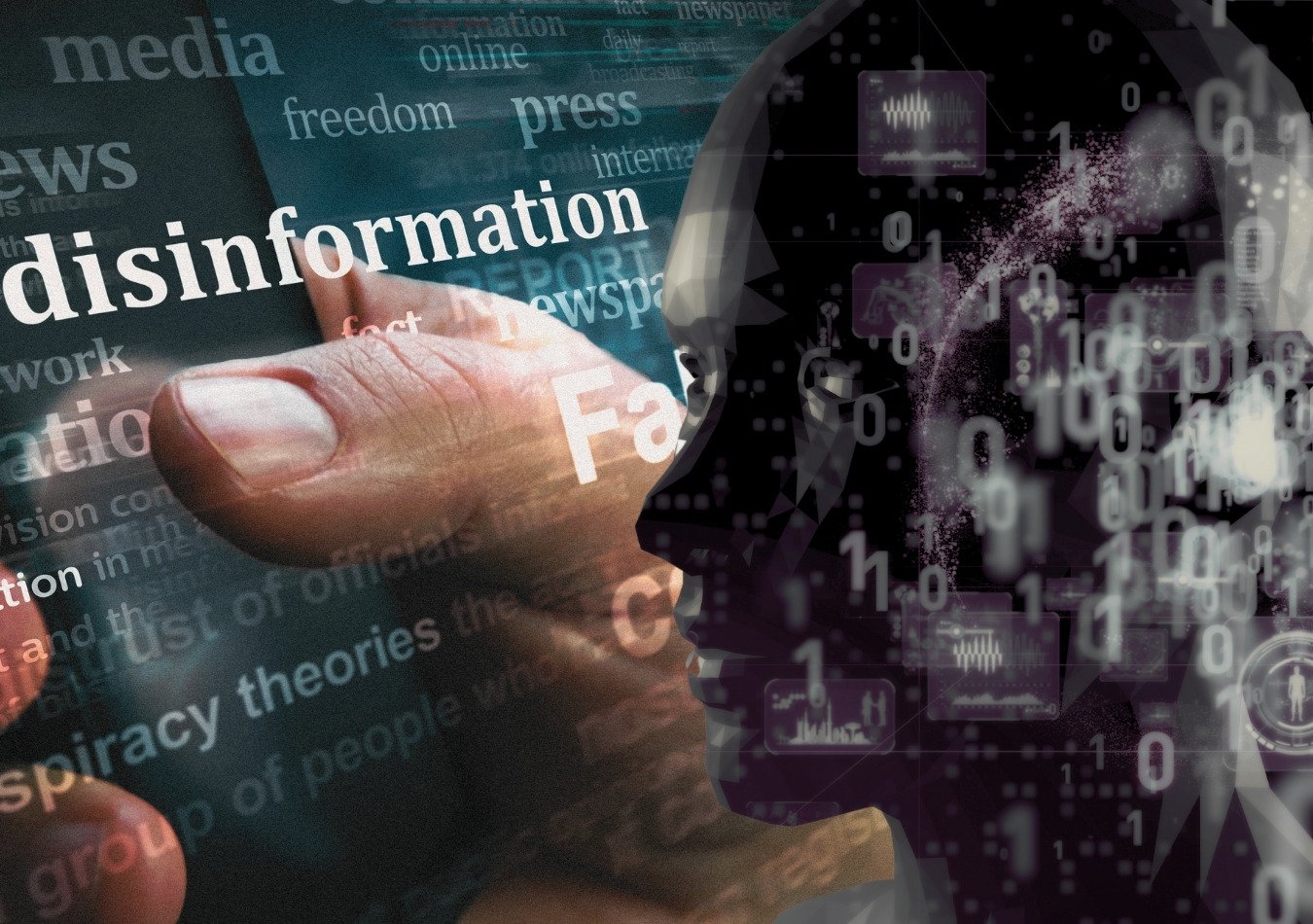
Additionally, the model provides information on almost double the number of sources, 49.6% more than sharing networks.
These settings imitated the strategic choices fact-checkers have to make in practice as news sources emerge over time, the study noted.
Such an advancement could massively minimise the workload and technical challenges faced by fact-checkers regularly.
Reducing Fact-Checking Costs by Over A Quarter
Tracking fake news sources in real-time would benefit them more instead of proofing individual articles or posts.
Also, the researchers found that their method can identify fake news sources with equal accuracy, but at a significantly lower cost, reducing fact-checking expenses by over 75%.
The audience-based ML approach will be able to produce reliable results over time, TechXplore reported.
However, the system requires additional real-world training before it’s ready to enter the market.
Currently, the model has yet to master spotting misinformation in textual features in real-time data which tends to drop significantly between 12 to 18.7% as per the study.
It’s possible that linguistic cues used to identify fake news in historical data may not be as effective in real-time.
Dr Grinberg emphasises that machine systems should never replace human fact-checkers, however, "it can greatly expand the coverage of today's fact-checkers.”
The study was published in the journal – Proceedings of the 30th ACM SIGKDD Conference on Knowledge Discovery and Data Mining.
Watch this video by EM360Tech about AI spotting fake news.
Can AI Detect Fake News?
Yes, there is AI software that detects fake news. For instance, large language models (LLMs) have been used to create AI chatbots such as ChatGPT that are now being subscribed to to spot fake news. Such AI models checking for fake news can likely caution and even counteract serious harms from deepfakes, propaganda, conspiracy theories and misinformation.
The new AI models could have the potential to also personalise the detection of manipulated content and protect users against it.
Now neuroscience is also playing a role in helping scientists discover information being absorbed unconsciously. According to TechXplore, biomarkers like heart rate, eye movements and brain activity appear to subtly change in response to fake and real content.
AI fake news checkers are mincing such biomarkers by learning from their data regarding human eye movement and electric brain activity. This exhibits the greatest impact of false content neurally, psychologically and emotionally. It could essentially spot or predict the kind of information that could trigger the most severe reaction in the consumer.