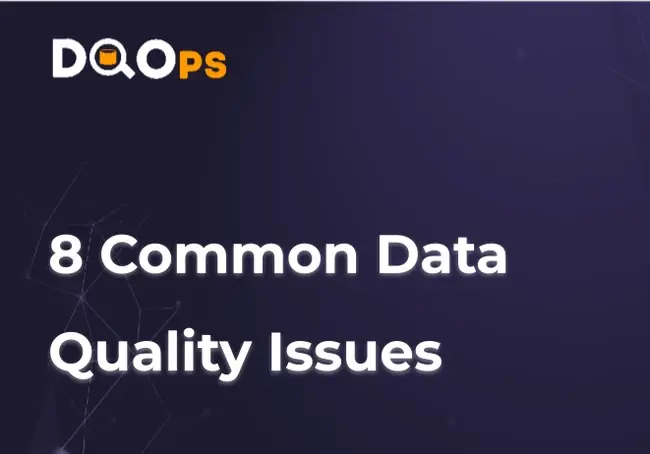
Data is the lifeblood of modern organizations, driving informed decisions and fueling business growth. However, data is rarely perfect. It's often plagued by various quality issues that can undermine its accuracy, consistency, and trustworthiness. These issues can range from simple formatting errors to complex inconsistencies across multiple systems. Even seemingly minor errors can have significant consequences, leading to inaccurate analysis, flawed reporting, and misguided decisions.
How Users Notice Data Quality Issues
Data quality issues often manifest in highly visible ways, particularly within dashboards and reports where users interact with data directly. Users are quick to notice anomalies such as distorted visualizations, inconsistent data, and unexpected values. These issues can lead to frustration, distrust, and a reluctance to rely on data for decision-making. For example, outliers can skew charts and graphs, making it difficult to discern meaningful trends, while duplicate entries or inconsistent formatting can create confusion and raise questions about the data's accuracy.
Most Common Data Quality Issues
Several types of data quality issues commonly occur, impacting various aspects of data integrity. Value-level issues concern the accuracy and validity of individual data points. Extreme values, or outliers, are data points that significantly deviate from the norm, often distorting visualizations and analysis. For example, a single abnormally high sales figure can dwarf all other values in a chart. Values that make no sense are illogical or impossible values that contradict common sense or known constraints, such as a negative inventory count or an age of 300 years. These errors often stand out when reviewing the data.
Structural issues relate to the organization and structure of the data itself. Inconsistent data formats involve variations in how the same type of data is represented, making it difficult to compare and analyze. For example, phone numbers might be stored in different formats (e.g., (555) 123-4567, 555-123-4567, 5551234567). These inconsistencies are often visible when inspecting or sorting data. Values appearing in wrong columns is another structural issue, where data values appear in incorrect fields, potentially leading to misinterpretations and incorrect analysis. For example, customer names might appear in the address column, or sales figures might be entered in the marketing spend column. These errors can often be spotted by comparing column headers to the data they contain.
Temporal issues concern the timeliness and consistency of data over time. Missing updates occur when recent changes in source systems are not reflected in downstream data, leading to decisions based on outdated information. Users might notice discrepancies between data in different systems or observe that expected changes are not reflected in reports. Sudden jumps in values are unexpected spikes or drops in data over time, potentially indicating data processing errors or inconsistencies in data collection. These jumps are easily spotted in charts and graphs, appearing as sharp increases or decreases in values.
Finally, completeness and uniqueness issues relate to the presence of all necessary data and the absence of redundant data. Duplicates are multiple entries representing the same entity, which can inflate counts, skew metrics, and make it difficult to identify unique records. Users might encounter duplicate entries when searching for records or notice inconsistencies in counts or aggregations.
How Data Observability Detects Common Data Quality Issues
Data observability platforms, such as DQOps, offer a proactive approach to data quality management by continuously monitoring data for issues and alerting teams to potential problems. These platforms leverage machine learning and time-series analysis to detect anomalies, inconsistencies, and other data quality issues that may otherwise go unnoticed. By tracking historical data and identifying trends, they can quickly spot outliers, missing data points, or inconsistencies in data formats. This real-time monitoring enables data teams to address issues promptly, preventing them from impacting downstream systems and users.