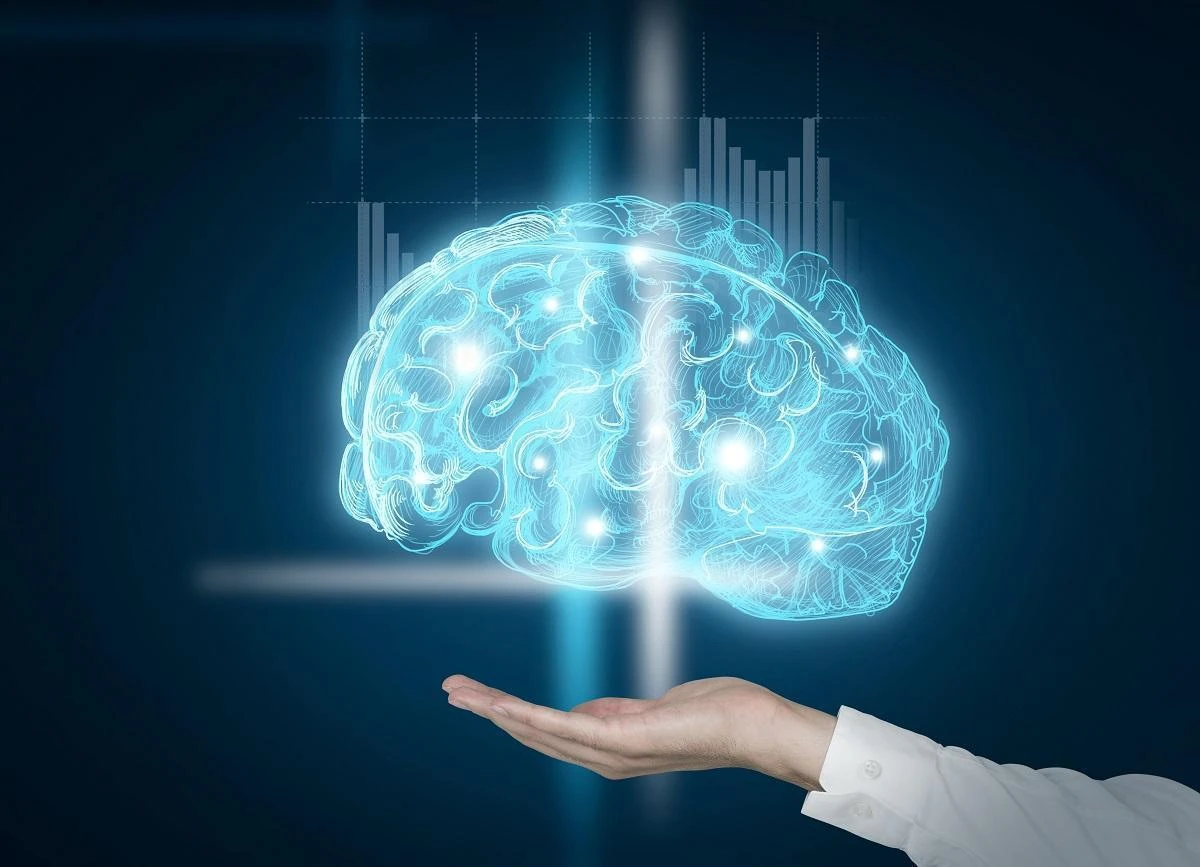
It’s commonly believed that smaller startups are generally more dynamic and more innovative than larger, established market leaders. And it’s a perception which is prevalent in all industries.
But it’s not always the case, since the creation of innovative products and services is often very expensive, and only a company with significant resources can realise these types of ambitions.
Much as we’d prefer not to use Apple as an example – because we can’t afford their fancy-pants products – but its latest smartphones always pack a huge number of innovations, both in terms of hardware and software.
Starting up in hardware is probably far more costly and complex – because manufacturing can be a nightmare, and so too can logistics. In fact, everything in the real world is a nightmare.
Software is more of a level playing field. Basically, all you need is a computer and an internet connection, and if you can code, you can pretty much do anything.
These days, big tech companies like Google, IBM, Intel, and even Apple and Baidu are falling over themselves to offer cloud-based software tools for developers.
These companies are particularly interested in finding people with talent, skills and development experience in the areas of artificial intelligence and its branches, machine learning and deep learning.
Many a small startup has been bought for big bucks by one of the big-tech moneybags, Google being the most acquisitive, according to CBInsights.
The data research website calculates that around half of all AI startups acquired since 2012 have venture capital backing from some giant company or other.
But it’s not all about selling up or selling out, depending on your point of view. It’s about developing powerful AI solutions which are not only interesting now, but will also prove to be important in the future.
So what we’ve tried to do in this top 10 is round up some companies which have either been acquired for a large sum of money in the past year or two, or have an interesting approach to developing AI solutions which may prove important in the future.
And while we are calling it the top 10 machine learning companies, it’s probably more accurate to say it’s about AI with an emphasis on ML.
Also, although we’re looking to find less well-known companies which are either developing or using machine learning in interesting and innovative ways, it’s impossible to ignore the big tech companies.
And for those of us who might not know or remember, machine learning refers to a machine’s ability to learn. Meaning, it doesn’t have to be programmed for every single detail of a specific situation, just given parameters for its decision-making, which, together, are usually called machine learning models.
Google
Google is regarded by experts to be the most advanced company in the field of AI, machine learning and deep learning.
The main reason for this is probably the amount of money the company has spent acquiring startups – Google has spent more than any other, according to CB Insights.
Whether it’s something it acquired or developed in-house, Google last week launched an AI chatbot which answers your messages for you – like a more sophisticated auto-response email – in a range of contexts, including Skype, Slack and Twitter direct messages.
But Google’s strongest point in this area is probably the range of cloud-based services it offers developers, including the Google Cloud AI machine learning tools.
It’s worth noting that Facebook, Microsoft and others are encouraging the development of chatbots for use in their messenger apps.
And although chatbots have had some bad publicity for using profane language and displaying signs of burgeoning bigotry, chatbots can be useful in filtering out spam and, er, bigotry, ironically enough.
There are, of course, other companies offering similar services, and we will mention some of them below. But, without evaluating them on a technical level, we can’t really say if one is better than the other – it’s just our perceptions about a range of factors.
IBM
A long time ago – way back in the 1990s – IBM challenged Russia’s greatest chess player, Garry Kasparov, to a match against its Deep Blue computer.
Kasparov trounced Deep Blue in the first match, but then the Russian grandmaster raged against the machine after losing to it in subsequent contests.
He probably still hasn’t got over it, claiming there was something amiss in the way IBM played. Others, however, have urged Kasparov to play along and admit defeat, but he still refuses to do what he’s told.
The successor to Deep Blue is the famous Watson AI computer, which beat the best contestants on a US television quiz show called Jeopardy!.
Watson Machine Learning is available much like Google Cloud AI machine learning is, but being elitist, IBM probably charges more.
And IBM’s chess challenge has been usurped in terms of media hype by the recent human-vs-machine contest over an ancient board game called “Go”, which was won by the machine, of course.
In this instance, the machine – or algorithm – was developed by DeepMind, which Google bought a few years ago for about $525 million.
Baidu
Another company which has been very active on the mergers and acquisitions scene is Chinese search giant Baidu.
The company is particularly interested in natural language processing, with a view to developing a workable voice-activated search function.
One of the many machine learning acquisitions Baidu made last year was Kitt.ai, which is said to have a portfolio of chatbots and voice-based applications. The financial terms of that particular deal were not disclosed but Baidu is said to be the 10th biggest-spender on acquisitions in the world, according to CB Insights, which says Google spends the most.
Google’s voice search and voice dictation for Google Docs are not totally terrible, but the problem for tech companies is that human users expect voice systems to be comparable in quality to the experience of talking to another human, and they’re a long way from that now.
The solution may not lie entirely in the software. Machine learning may provide powerful ways of learning how people speak. But an eventual solution may require an upgrade of the hardware. Specifically, the microphones may need to become more sensitive or something.
Whatever the solution, voice systems are yet to pass that quality or usability threshold.
Microsoft
Microsoft has actually been the third-biggest spender on acquisitions over the past five years, according to CB Insights.
The company is well and truly into the internet market, especially after its $26 billion purchase of LinkedIn a couple of years ago.
LinkedIn may provide the best platform for Microsoft to showcase the enterprise applications it develops based on machine learning.
But probably the most significant acquisition Microsoft made in the machine learning space was Maluuba, which the tech giant says has “one of the world’s most impressive deep learning research labs for natural language understanding”.
Voice computing does seem to be the key area of competition between tech giants because success in this field would open up more than just voice search. It would make typing and many other tasks unnecessary.
Twitter
Although Twitter has been one of the world’s largest social networks for many years, it hasn’t been profitable until very recently.
One of the reasons it has seen an increase in revenues leading to profitability is said by analysts to be a change in the way main feed items are chosen by its competitor Facebook.
Facebook is said to have changed the algorithm for choosing main feed items to include fewer news items from recognised media outlets in favour of items from friends and family.
Whatever the reason for Twitter’s profitability, using machine learning to try and choose what people might be interested in is something of great interest to tech companies.
Qubit
As well as using machine learning to choose items of interest for social media feeds, companies are also artificial intelligence to try and find items shoppers are more likely to buy.
Qubit is an example of such a company. Qubit has developed an AI-powered, personalised shopping app called Aura.
The company has patents pending on what is described by MarTechToday.com as a “discovery solution”, which provides an “Instagram-like feed” of images of products users may be interested in.
The Aura app has a database of products from a variety of categories including cosmetics, fashion, clothing and so on, and Qubit partnered with numerous brand names in the development phase.
Amazon has done phenomenally well with its suggestion engine for years, from way before the current interest in machine learning.
But now with companies like Qubit developing more personalised, mobile-oriented shopping experiences, it will be interesting to see how it affects the bottom line of the online retail giant.
Intel
Data processing for artificial intelligence or machine learning applications is said to be slightly different from other types.
Whatever the details, experts say that a new generation of chips being built will be more capable of running AI and machine learning apps.
Still the largest chipmaker in the world, Intel clearly does not want to miss out on this nascent market, and has been active in acquisitions.
One of the largest purchases Intel made in the past few years is the $400 million acquisition of Nervana Systems, which build chips for data centre servers.
Nervana chips are said to be able to transfer data in and out at 2.4 terabytes per second at very low latency. That is supposed to be five to 10 times faster than the fastest input-out interfaces for traditional chips, according to a report on Forbes.
Similarly fast chips for AI have been released by Google and Nvidia, among others, so this could be a critical area of competition for Intel. Amazon is also said to be developing a custom chip for its Alexa AI system, according to TheVerge.com.
Apple
There might be a perception that Apple is late to the machine learning party, but that’s probably not true, especially since it was the first to launch a voice assistant on a smartphone.
Millions of people talk to Siri, even if we don’t, and Apple is looking to extend the application of the talking assistant through its new smart home device or speaker, the HomePod.
Amazon’s Alexa-powered Echo devices and Google Home Assistant for competition, Apple has its work cut out.
But if these companies do take the competition seriously, the inevitable result will be the technology improves and the end user benefits.
Apple has also been active in acquisitions – second only to Google. One of the more notable purchases has been Lattice Data, which has a machine learning system for converting unstructured data – like random text and pictures – into structured data.
Apple paid $200 for Lattice Data, according to 9to5Mac.com.
The company has also allocated significant resources for the development of in-house machine learning systems, many of which are available through its developer program.
Salesforce
It’s probably to be expected that the world’s leading sales platform is very active in acquisitions. CB Insights estimates that Salesforce has been the sixth-largest buyer of AI companies over the past five years.
One of the machine learning systems Salesforce was keen to highlight last year was its so-called “Einstein” technology, which is said to analyse “every aspect of a customer’s relationship” with a particular company.
According to WordStream.com, Einstein enables Salesforce users to build up much more detailed profiles of their customers.
HubSpot, another customer relations management software provider, also uses a machine learning system, called DeepGraph, to analyse prospects and clients.
Pindrop
Pindrop has developed what the company says is a “pioneering” technology for recognising fraudulent activity over the phone channel.
In other words, its “Phoneprinting” technology can create what Pindrop describes as an “audio fingerprint” of each call by analysing 1,300 unique call features – such as background noise, location, number history, and call type – toidentify unusual activity.
The system automatically flags suspicious calls and can even spot ID spoofing, voice distortion and social engineering.
Pindrop’s system is being applied by leading companies such as Lloyds Banking Group.
While many of the stories in the past couple of years have been about how machine learning can be used to analyse images and video, the applications relating to human voice are at least as important.
The new iPhone may be able to unlock itself when it recognises your face, but what you’d probably find more useful is if Siri could save you from all the typing you have to do – for work and in your personal life.
It does seem an extremely tricky challenge, though, since not only is each one of the faces unique, our voices and the way we speak are also particular to us.
Meaning, there probably is no universal solution – one machine learning application that can understand everyone. For now, at least, what might be needed is a solution that is particular to each device and, by extension, each user.
But that sort of solution would only apply to devices like smartphones, which generally have just one user.
Devices which have more than one user will probably have to wait before the technologists figure out a way to give them the necessary wherewithal to understand the world around them and the human voices they hear around them.
But AI and machine learning have many other applications beyond analysing images and sounds, so it will be interesting to see how the world is changed by it.
Comments ( 0 )