AWS vs Azure: What’s the Difference in 2024?
Anodot: Ultimate Guide To Building A Machine Learning Outlier Detection System Part II
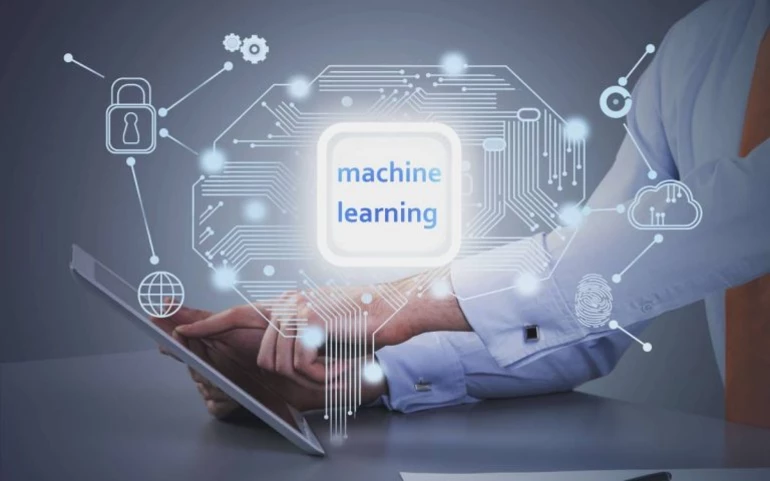
Outlier detection helps companies determine when something changes in their normal business patterns. When done well, it can give a company the insight it needs to investigate the root cause of the change, make decisions, and take actions that can save money (or prevent losing it) and potentially create new business opportunities. High-velocity online businesses need real-time outlier detection; waiting for days or weeks after the outlier occurs is simply too late to have a material impact on a fast-paced business. This puts constraints on the system to learn to identify outliers quickly, even if there are a million or more relevant metrics and the underlying data patterns are complicated. Automated outlier detection is a technique of machine learning, and it is a tremendously complex endeavor. In this series of white papers, Anodot aims to help people understand some of the sophisticated decisions behind the algorithms that comprise an automated outlier detection system for large scale analytics, especially in the monitoring of time series data.
Recommended Content
Trending Content
Infinipoint: Integrating Device and User Authentication to Achieve Zero Trust Workforce Access
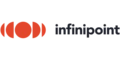
What is Multi-Cloud Architecture? Definition & Use Cases
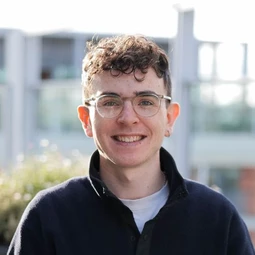
Infinipoint - Rethinking Workforce Access: Augmenting Authentication with Zero Device Trust
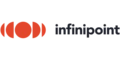
Meet MAI-1: The New AI Model by Microsoft that Rivals GPT-4
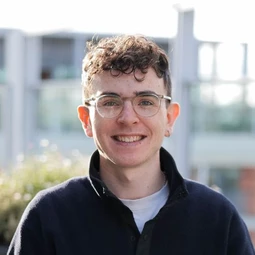
Ministry of Defence Data Exposed in China-Linked Cyber Attack
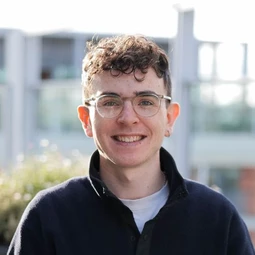