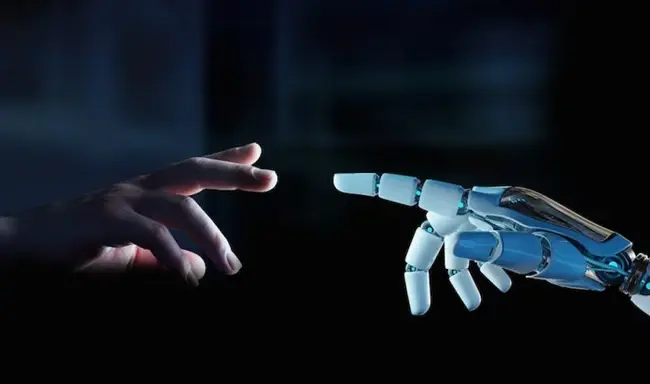
More enterprises are now beginning to reap the benefits of artificial intelligence (AI) and machine learning (ML). While this technology brings a host of rewards, it is also necessary to bridge the growing data literacy gap between AI and humans.
Bridging the gap between AI and humans
In order to extract value from AI projects, it is vital that stakeholders are able to easily understand results. In effect, it is now more important than ever to accurately interpret and effectively communicate findings.
As a new e-book from DataRobot notes, ML use cases, metrics, and charts can be difficult to comprehend, however. Indeed, explaining the AI problem to solve, ML models, and the relationships among variables is often "subtle, surprising and complex."
Analytical communicators must therefore educate stakeholders throughout the entire AI project, rather than during its conclusion. In turn, organisations can make positive steps towards becoming truly AI-driven.
Becoming AI-driven
Although AI is rapidly improving and simplifying processes in the enterprise, many people remain sceptical. DataRobot insists that analysts must therefore prepare to defuse scepticism, apprehension, and fear of the unknown by "relating AI to the processes and tools people already use."
In order to execute this, the company recommends developing an AI roadmap. Organisations must therefore begin by selecting and defining the appropriate AI use cases with an estimated ROI, payback period, and risk assessment.
Turning an AI project from an idea into reality means that analysts must communicate throughout the entire process, however. "Successful AI translators use the entire machine learning project life-cycle to communicate," DataRobot stresses.
This can entail accurately defining projects to visualising findings, which will ultimately deliver more value by consulting subject matter experts. Rather than using unfamiliar graphs, analysts can also make use of storyboards and visualisations in order to decode confusing data science.
Check out the Top 10 Must-Haves in a Machine Learning Platform