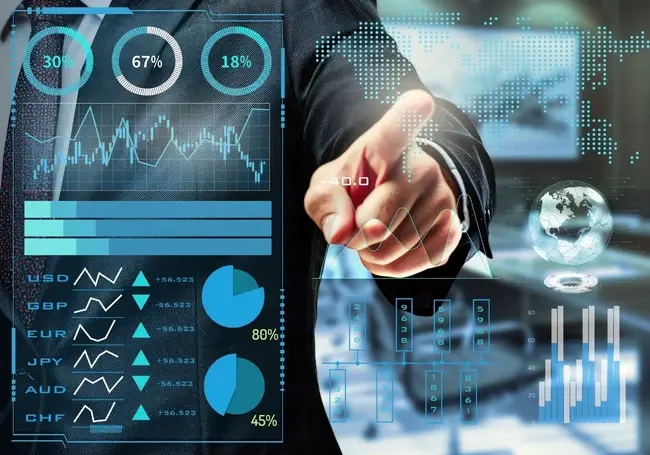
Everyone - i.e. not just a handful of power users - should be able to explore and draw insights from business data. This is the thinking behind self service analytics; a user-centric approach to data analysis that places accessibility and empowerment front and centre.
Not so long ago, the self service analytics label was confined mostly to an emerging breed of easy-to-use and easy-to-deploy BI (business intelligence) tools. These days, self service features can be found in even the most powerful enterprise analytics and BI solutions.
Here’s a closer look at what self service analytics means for IT decision makers and the wider business, and at the self service analytics platform features to look for when considering analytics solutions.
Self service analytics definition: What is self service analytics?
Self service analytics is a user-focused approach to business intelligence. It shares BI’s core aim: enabling businesses to transform raw data into understandable, actionable information.
The big difference between self service analytics tools and traditional BI solutions relates to how that information is generated and consumed.
Under the traditional approach, direct interaction with the data tends to be confined to a handful of specialists. A company’s IT team will generally create and maintain the solution’s data pipeline. Data specialists design and manage the schemas and models for analysis, and design reports. Non-technical users - e.g. your finance manager and heads of department - are consumers of that information, rather than creators of it.
Self service analytics changes this. Under Gartner’s classic self service analytics definition, it’s an approach where “line-of-business professionals are enabled and encouraged to perform queries and generate reports on their own, with nominal IT support”.
What are typical self service features?
These are some of the most common characteristics you can expect to find in a self service analytics platform:
Intuitive interfaces
The look and feel of self service platforms reflects the desire to make sense to a wide range of non-technical users. Instead of a dense layout dominated by text and tables, the typical interface is more akin to a consumer-facing app. Common design elements include intuitive icons, sleek and simple navigation, and an emphasis on data visualisations over text.
No-code report generation
The ability to use business data without the need for advanced technical skills is foundational to self service data analytics. Often working from pre-designed templates, users can populate and customise their own reports, or build them from scratch by dragging and dropping elements into the workspace.
Guided instruction
With traditional BI, a high level of user proficiency is often assumed. Self service analytics tools focus more on contextual guidance. Examples include pointers, suggestions, and step-by-step ‘wizards’ that guide users through specific processes.
Easy search capabilities
Self service analytics platforms focus on making it as easy as possible to get to the information you want, right at the point of need. For instance, you can typically click on a graph, and drill down to the granular data underneath. Search bar functionality is common, too. Type in a question (e.g. “What are this quarter’s Europe sales figures?”), and the platform will generate a report in response.
Visualisation
Self service analytics tools (Tableau, for instance) often include a wide range of user-friendly data visualisation capabilities. The emphasis is on making your data story as clear and compelling as possible. Options for users to experiment with can include everything from collapsible tables with drill-down functionality, heatmaps, and interactive charts, right through to infographics. Some self service analytics platforms will even offer you visualisation recommendations based on the nature of the dataset.
Benefits of self service analytics
Core benefits of self service analytics for tech leaders and the wider business include the following:
Building a data driven culture
Organisations often adopt data analytics to promote more evidence-based decision-making. Self service data analytics actively supports this, by empowering users to actually drill into the data themselves, to experiment with analysis, and uncover insights. Working with data can quickly become second nature.
Faster decision making
Let’s say a procurement manager has been offered a time-limited opportunity, but needs to run through the numbers to check if it’s viable. With self service, users can submit queries and create their own reports on-demand - and make evidence-based decisions faster.
Ease of implementation
Intuitive, user-centric design means that ordinary business users can rapidly start using self service tools independently, often with relatively little formal training required. For IT, this can mean a smoother, faster deployment, with a lower spend on training initiatives compared to traditional BI.
Fewer demands on specialist teams
In contrast to traditional BI, self service analytics can mean fewer ad-hoc report requests for IT or data specialists to handle. IT resources are potentially freed up for more valuable digital transformation projects across the business. Likewise, with a lower routine reporting burden, senior data specialists can focus more on initiatives such as strategic planning.
Self service analytics tools v enterprise BI: Is there still a difference?
Until about a decade or so ago, there was a clear distinction between self service analytics and enterprise BI.
Self service tools (Tableau and Qlik, for instance) have helped to revolutionise data analytics, making it far easier for stakeholders right across the business to put data to work by generating their own reports.
However, especially when they first emerged, the earliest self service platforms tended to rely on a scaled down data model, often comprising topline data from specific areas of the business.
Large organisations - particularly those with complex data architectures, and very large datasets to analyse - tend to have sophisticated integration and data governance needs; something that self service tools have often struggled to meet. An assumption arose that self service analytics tools were for SMEs, while enterprises tended to stick with traditional BI.
These days, that distinction is less relevant. On one hand, there are solutions - Microsoft Power BI being a prime example - that are specifically designed to meet the needs of enterprise, AND have very strong self-service capabilities.
What you also find is that many long-established enterprise BI vendors (the likes of IBM, Cognos, and SAP) have adopted self service principles across many of their solutions.
The result? There is often no need to choose between highly scalable enterprise-grade tools and self service capabilities. Many solutions offer both.