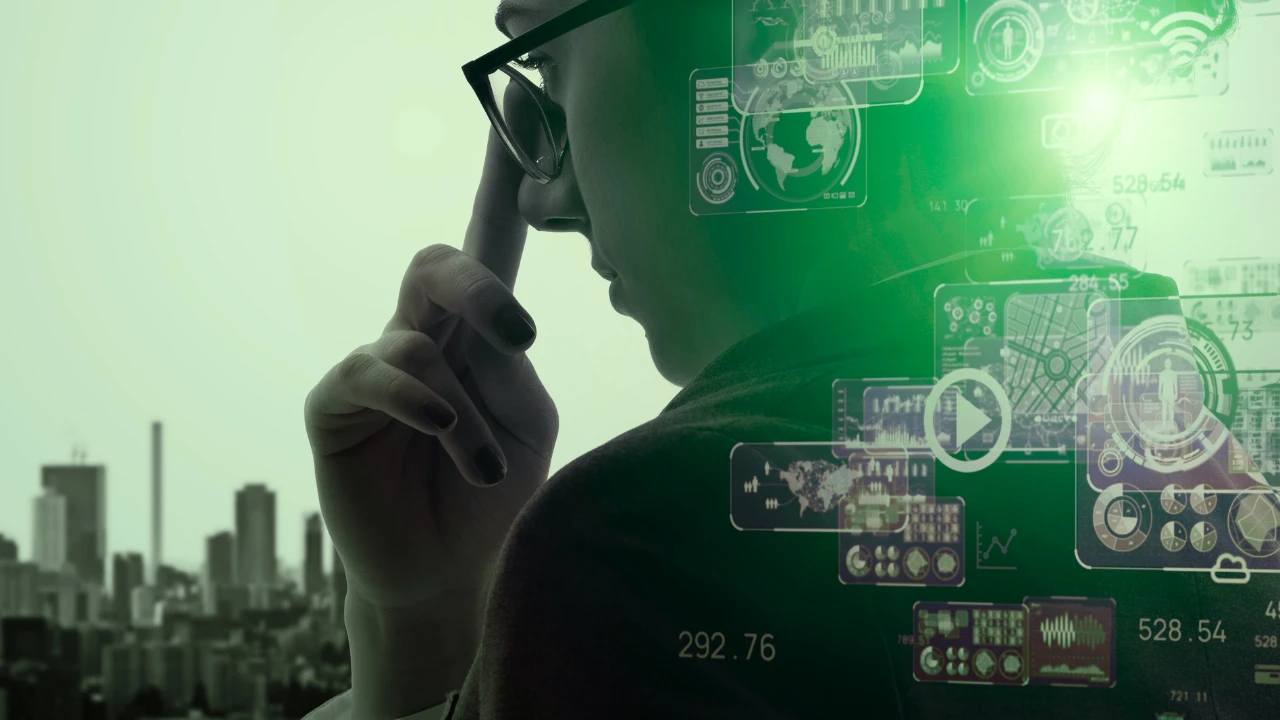
Isn’t it ironic? We’ve got these cutting-edge AI tools that were designed to cut costs, but they’re actually slowing things down because of how costly AI deployment is. Sam Altman, the CEO of OpenAI, has openly shared that training their latest GPT model cost upwards of 100 million USD.
Back in 2021, when AI was just starting to make waves, nobody predicted that by 2025, a staggering 90% of businesses would be pumping the brakes on their AI deployments because the costs were starting to outweigh the benefits. But, according to a recent Gartner report, that’s what’s happening. Additionally, more than 50% of the businesses that tried to build their own large models may quit because it’s becoming too expensive and complex.
The costs of developing, training, and storing AI are on a steady rise year after year. And then there are the hidden costs that caught everyone off guard. In this article, we’re going to delve into all of these costs, how they’re impacting budgets and teams worldwide, as well as discuss potential strategies to mitigate these issues.
Big Budgets, Bigger Surprises
In an effort to stay competitive and meet increasing demand, companies are ramping up their AI budgets. Not all companies had separate AI budgets in the past and often lumped these costs into their overall IT budgets. The seventh annual Appen State of AI report highlights a significant increase in AI budgets across companies of all sizes. On average, companies are allocating between $500,000 to $5 million per year for AI — a big 55% increase from 2020.
Excluded from these budgets, however, are the costs to recruit and retain software developers. A global shortage of more than 80 million developers is expected by 2030, with companies worldwide risking losing $8.4 trillion in revenue because of the lack of skilled talent. Traditional recruitment and hiring methods that glorify formal university computer science credentials are also contributing to the problem. A Stack Overflow survey shows 87% of the respondents admit to have taught themselves a coding language or framework without a formal course, suggesting that transformation doesn’t happen just in the development of AI, but should also happen in the way engineers are recruited to develop it.
Using and Storing Data
Interestingly, the true scale of the costs of storage and networking surprised many UK enterprises. A Seagate survey of 500 tech leaders from mid-to-large UK companies found that many had to prioritize spending on data management over other important areas like employee welfare, training, and even energy costs. What’s more, over 50% of the respondents revealed that their company’s senior leadership were in the dark about the magnitude of the problem.
Also, new research shows that 40% of businesses lack a data governance strategy, attributed to AI-induced “paralysis”. The Data Maturity Index survey found that 41% of businesses have minimal or no data governance framework.
So, it seems there’s a lot more to AI costs than meets the eye.
If you’re not managing your data efficiently throughout its lifecycle, your costs are just going to keep climbing. On top of the various costs, we mentioned so far, consider the big price tag that comes with cloud file and object data storage, which is needed to store the massive amounts of data pumped out by AI (images, audio, objects, and so on).
Most UK enterprises with over 1000 employees are shelling out more than 250K a year just on data storage. As Ravi Naik, Seagate’s executive vice president of storage and services, puts it, “UK Businesses are using and storing data at unprecedented levels.”
Compete by Computing
Companies everywhere are grappling with a GPU deficit, with Nvidia at the helm of both the development and market control of cards that are instrumental in training AI models. The previously mentioned figure from Sam Altman of 100 million USD refers to the cost of training a complete model using GPUs specifically designed for AI.
However, what about the cost implications of updating an existing model? The GPUs required for updating generative AI models can carry a massive price tag, potentially as high as 5M USD per model update.
As highlighted by the MIT Technology Review, the escalating resource requirements underscore the increasing costs associated with advancements in the field. To put it into perspective, compute usage has skyrocketed by a staggering 300,000-fold in the past seven years. Cutting right to the chase, this trend underscores the significant investment required to making progress in AI development is simply not for everyone.
In Conclusion, Time to Lead
Let’s not be taken aback by the reality - and the associated costs - that AI development demands substantial data, storage, and networking power. It’s a call to action for companies and startups involved in AI projects to explore new technologies that can reduce overall development expenses. These could include more cost-effective LLM APIs, strategic decisions about cloud versus data center usage, and data compression solutions that can dramatically reduce data storage and networking costs.
Comments ( 0 )