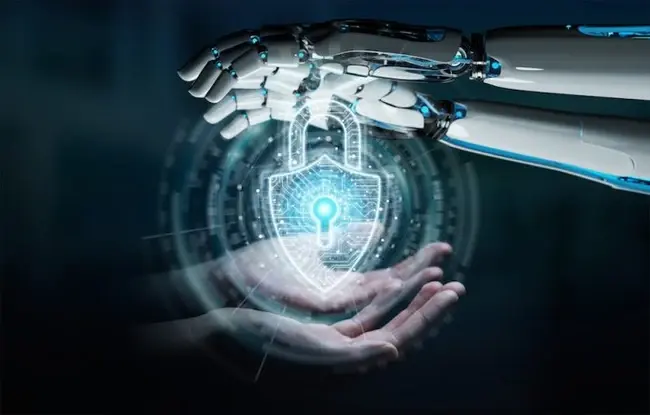
Today, more companies are opting to implement artificial intelligence (AI) in order to improve productivity, efficiency, and overall performance. In the security domain, many organisations are also using the technology to detect and contain cyberthreats.
How can AI and ML detect and contain cyberthreats?
Across the enterprise, the use of the term "AI" is so widespread that it has become difficult to see beyond the hype. In order to determine the true meaning of "AI-based" security, Lastline has released a detailed whitepaper explaining some key applications.
As Lastline notes, AI and machine learning (ML) have found widespread application in the security realm. In particular, supervised ML techniques have the potential to create powerful malware detection systems.
In effect, an ML-based system ingests a large amount of known-benign programs and known-malicious programs in order to generate a classifier. As a result, this enables the tool to determine whether an unknown program is malicious or not.
Companies can also use unsupervised ML to create clusters of similar data, which can address similar events or identify anomalies. Overall, however, approaches to the identification of malicious behaviour should entail both Misuse Detection and Anomaly Detection.
Misuse Detection and Anomaly Detection
The use of signatures to detect network attacks is just one example of a misuse detection approach. While this is generally very precise, this approach can only detect malicious behaviour with an existing model.
Meanwhile, anomaly detection uses a complementary approach by essentially creating a model to identify outliers that are outside the parameters of normality. This means that it is possible to identify previously unseen malicious behaviour, but this approach also makes the assumption that "what is anomalous is malicious and what is malicious will generate an anomaly."
Unfortunately, both assumptions do not hold at all times, causing both false positives and false negatives. Overall, it is evident that supervised ML can easily improve misuse detection, while unsupervised ML can perform anomaly detection.
By automating these two approaches, ML ultimately supports the scalable analysis of large datasets. Only by combining these two techniques is it possible to provide the best AI-detection, however.
Interested in ethical hacking? Check out our latest episode of Tech Chat, in which we spoke to Chloé Messdaghi, Security Researcher Advocate, Co-Founder of Women in Security and Founder of WomenHackerz